An Overview of Modern Machine Learning Methods for Effect Measure Modification Analyses in High-Dimensional Settings
SSM - population health(2025)
AI Read Science
Must-Reading Tree
Example
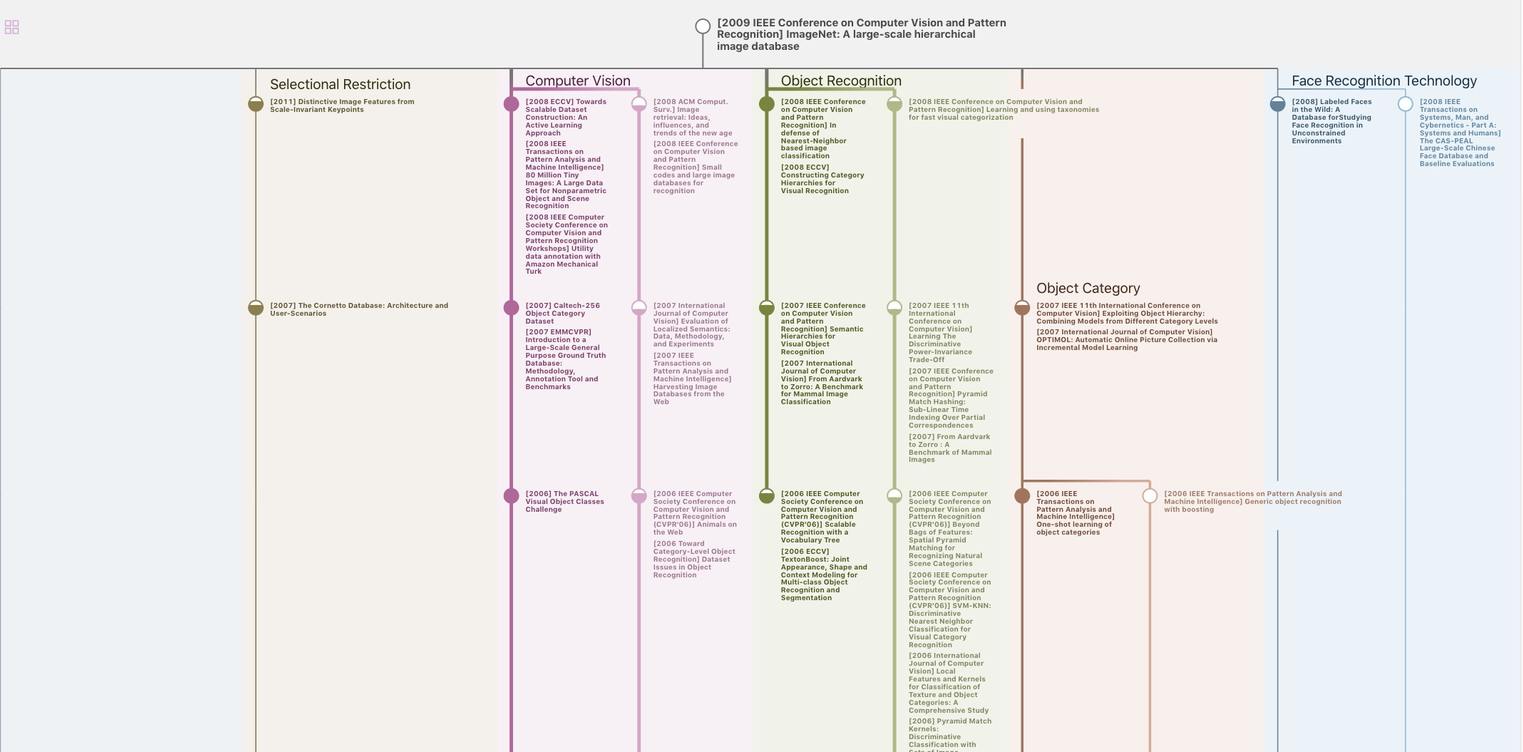
Generate MRT to find the research sequence of this paper
Chat Paper
Summary is being generated by the instructions you defined