An Information-Theoretic Analysis of In-Context Learning
ICML 2024(2024)
Abstract
Previous theoretical results pertaining to meta-learning on sequences build on contrived and convoluted mixing time assumptions. We introduce new information-theoretic tools that lead to a concise yet general decomposition of error for a Bayes optimal predictor into two components: meta-learning error and intra-task error. These tools unify analyses across many meta-learning challenges. To illustrate, we apply them to establish new results about in-context learning with transformers and corroborate existing results a simple linear setting. Our theoretical results characterize how error decays in both the number of training sequences and sequence lengths. Our results are very general; for example, they avoid contrived mixing time assumptions made by all prior results that establish decay of error with sequence length.
MoreTranslated text
AI Read Science
Must-Reading Tree
Example
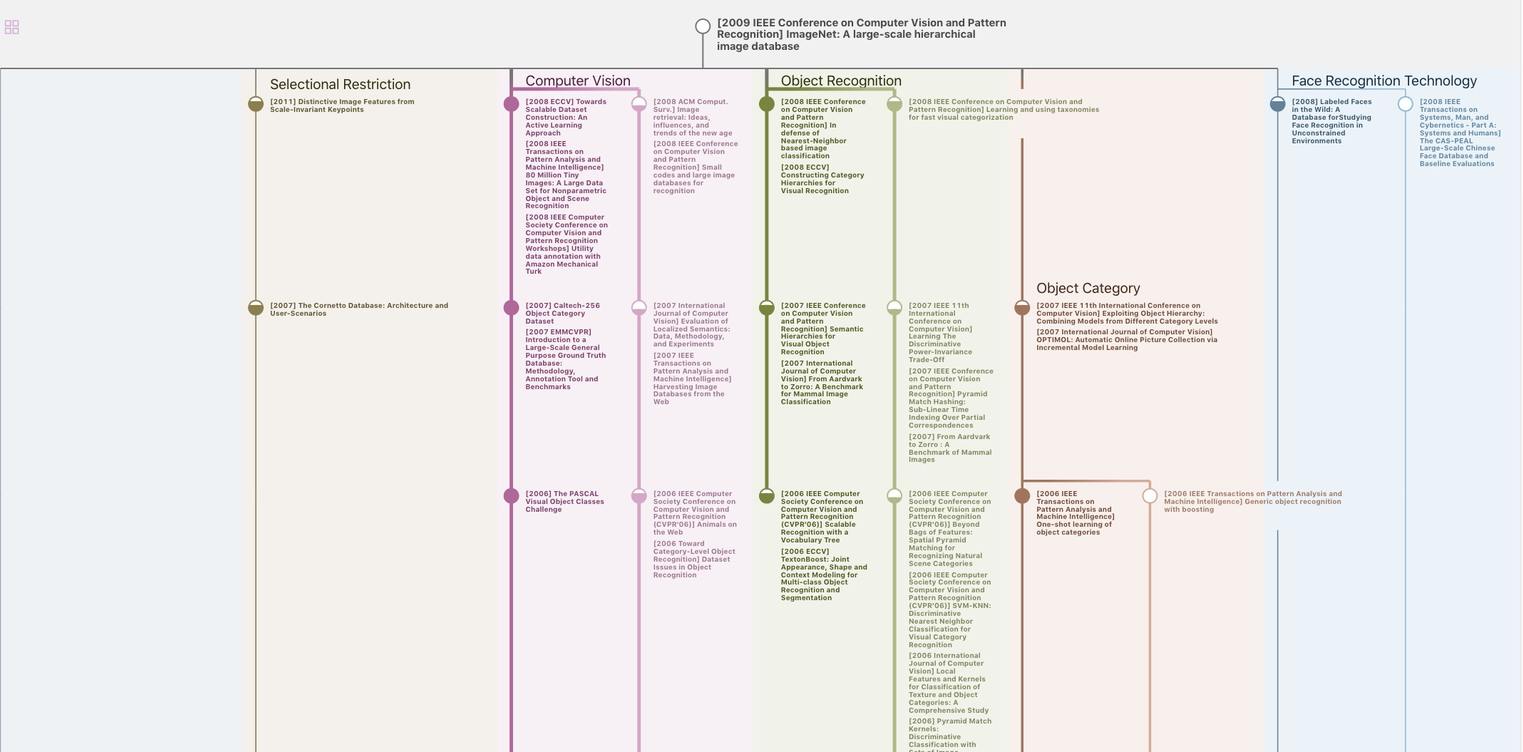
Generate MRT to find the research sequence of this paper
Chat Paper
Summary is being generated by the instructions you defined