On Partly Smoothness, Activity Identification and Faster Algorithms of L_1 over L_2 Minimization
arxiv(2024)
摘要
The L_1/L_2 norm ratio arose as a sparseness measure and attracted a
considerable amount of attention due to three merits: (i) sharper
approximations of L_0 compared to the L_1; (ii) parameter-free and
scale-invariant; (iii) more attractive than L_1 under highly-coherent
matrices.
In this paper, we first establish the partly smooth property of L_1 over
L_2 minimization relative to an active manifold M and also
demonstrate its prox-regularity property. Second, we reveal that ADMM_p (or
ADMM^+_p) can identify the active manifold within a finite iterations. This
discovery contributes to a deeper understanding of the optimization landscape
associated with L_1 over L_2 minimization. Third, we propose a novel
heuristic algorithm framework that combines ADMM_p (or ADMM^+_p) with a
globalized semismooth Newton method tailored for the active manifold M. This hybrid approach leverages the strengths of both methods to enhance
convergence. Finally, through extensive numerical simulations, we showcase the
superiority of our heuristic algorithm over existing state-of-the-art methods
for sparse recovery.
更多查看译文
AI 理解论文
溯源树
样例
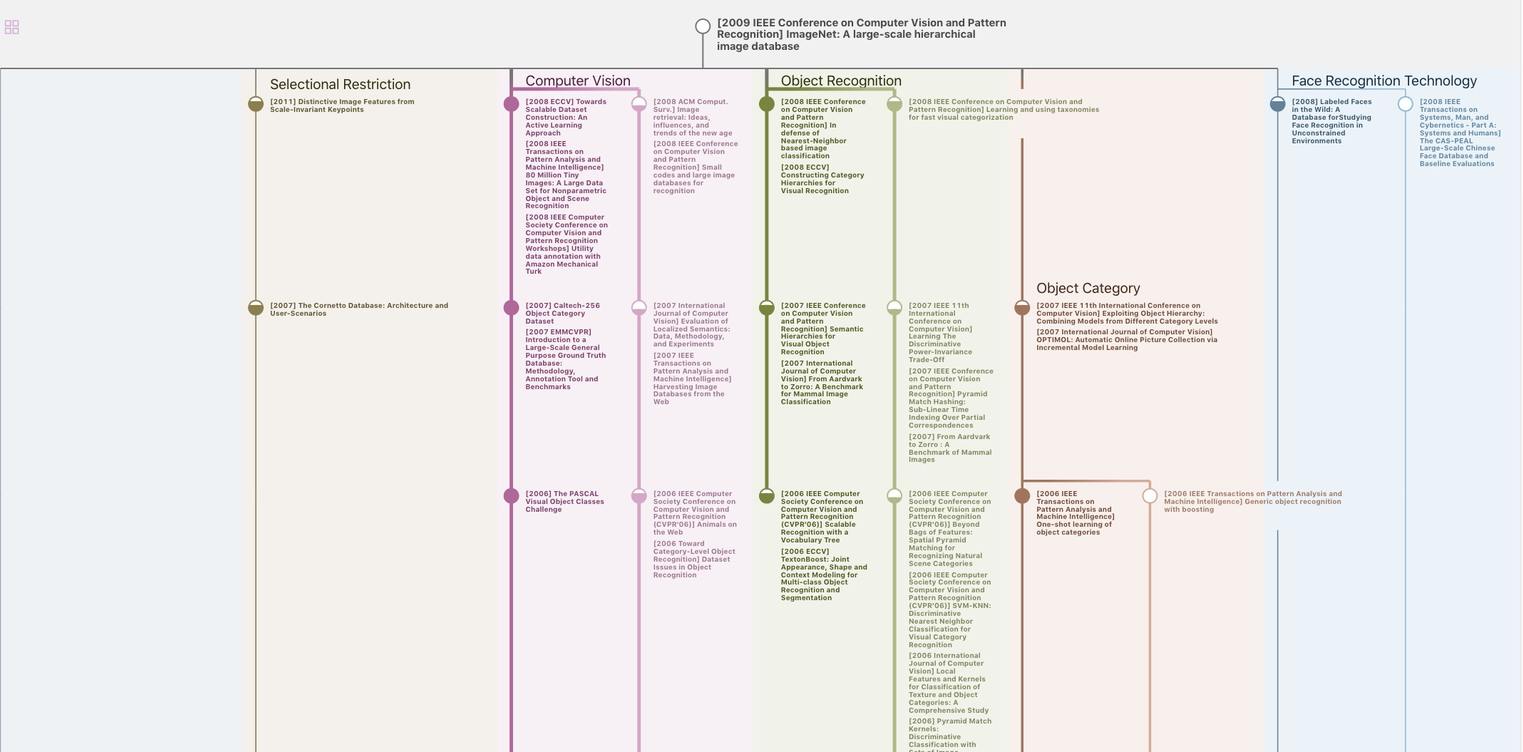
生成溯源树,研究论文发展脉络
Chat Paper
正在生成论文摘要