Side-constrained graph fusion for semi-supervised multi-view clustering
NEUROCOMPUTING(2024)
摘要
In these years, semi-supervised learning arouses ongoing attentions due to its appealing avail and economic expenditure of guiding model training. Semi-supervised multi-view clustering takes advantages of heterogeneous features and a small number of side constraints (i.e., must-link constraints and cannot-link constraints) to partition data points. However, most of existing approaches are very limited in absorbing available side constraints since it is difficult to optimize cannot-link constraints in a provable way, and thus greatly waste the value of prior information. To address it, we innovatively pose a side-constrained multi-view graph clustering method, where the pairwise constraints are flexibly incorporated into the multiple graph fusion framework. The technique definitely formulates the pairwise constraints in the graph clustering model by designing a semi-supervised graph regularization term. In this way, the structured optimal graph that satisfies multiple perspectives and specified pairwise relations is obtained. By virtue of graph fusion, the self-adaptive weight of each single-view is optimally determined without partiality. We demonstrate theoretical feasibility of the proposed method. Extensive experimental results in four multi-view data sets witness our superiority compared to the state-of-the-art approaches.
更多查看译文
关键词
Semi-supervised learning,Multi-view clustering,Side information,Graph fusion,Self-adaptive
AI 理解论文
溯源树
样例
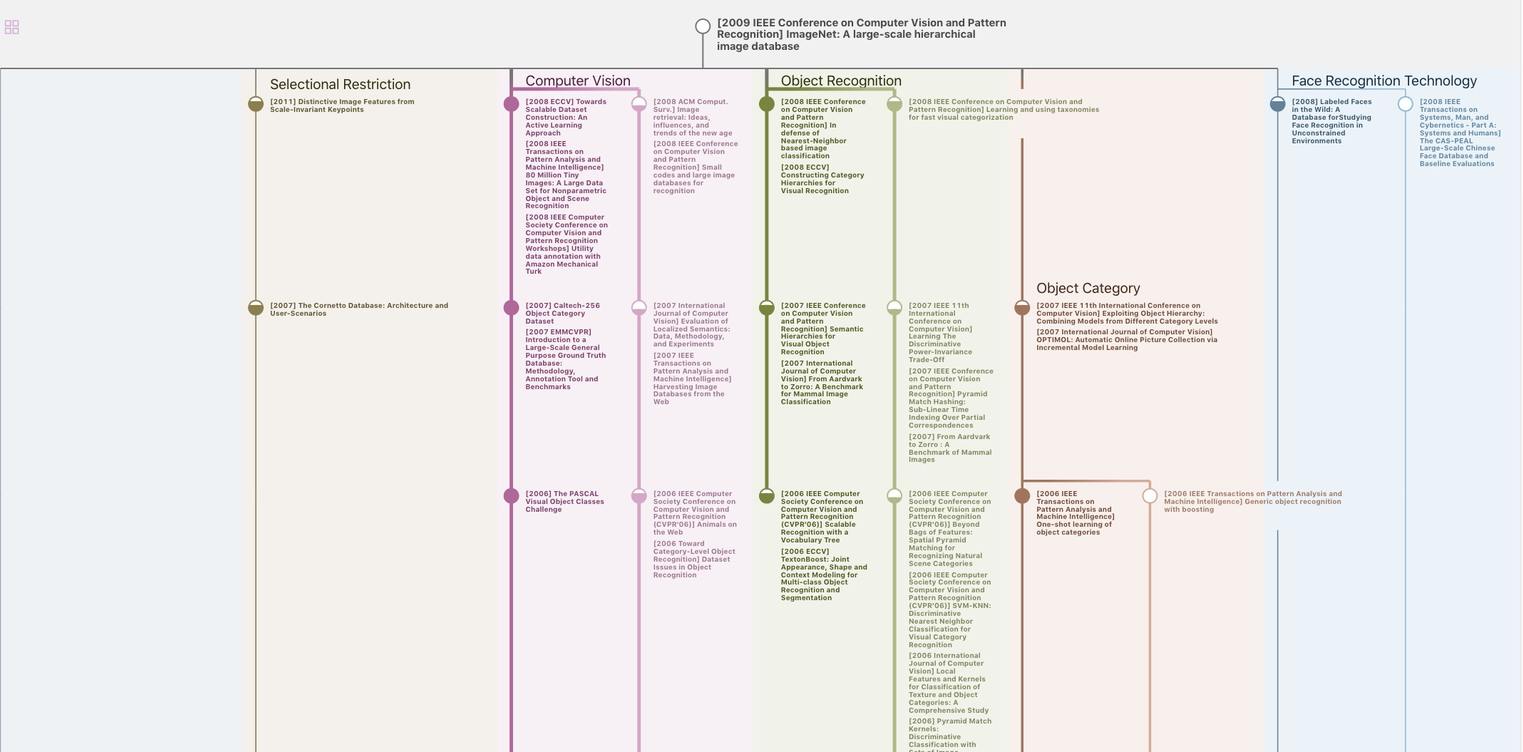
生成溯源树,研究论文发展脉络
Chat Paper
正在生成论文摘要