FairGap: Fairness-aware Recommendation via Generating Counterfactual GraphJust Accepted
ACM Transactions on Information Systems(2023)
摘要
The emergence of Graph Neural Networks (GNNs) has greatly advanced the development of recommendation systems. Recently, many researchers have leveraged GNN-based models to learn fair representations for users and items. However, current GNN-based models suffer from biased user-item interaction data, which negatively impacts recommendation fairness. Although there have been several studies employed adversarial learning to mitigate this issue in recommendation systems, they mostly focus on modifying the model training approach with fairness regularization and neglect direct intervention of biased interaction. Different from these models, this paper introduces a novel perspective by directly intervening in observed interactions to generate a counterfactual graph (called FairGap) that is not influenced by sensitive node attributes, enabling us to learn fair representations for users and items easily. We design the FairGap to answer the key counterfactual question: “ Would interactions with an item remain unchanged if user’s sensitive attributes were concealed? ”. We also provide theoretical proofs to show that our learning strategy via the counterfactual graph is unbiased in expectation. Moreover, we propose a fairness-enhancing mechanism to continuously improve user fairness in the graph-based recommendation. Extensive experimental results against state-of-the-art competitors and base models on three real-world datasets validate the effectiveness of our proposed model.
更多查看译文
关键词
fairness,recommendation,graph neural network,counterfactual
AI 理解论文
溯源树
样例
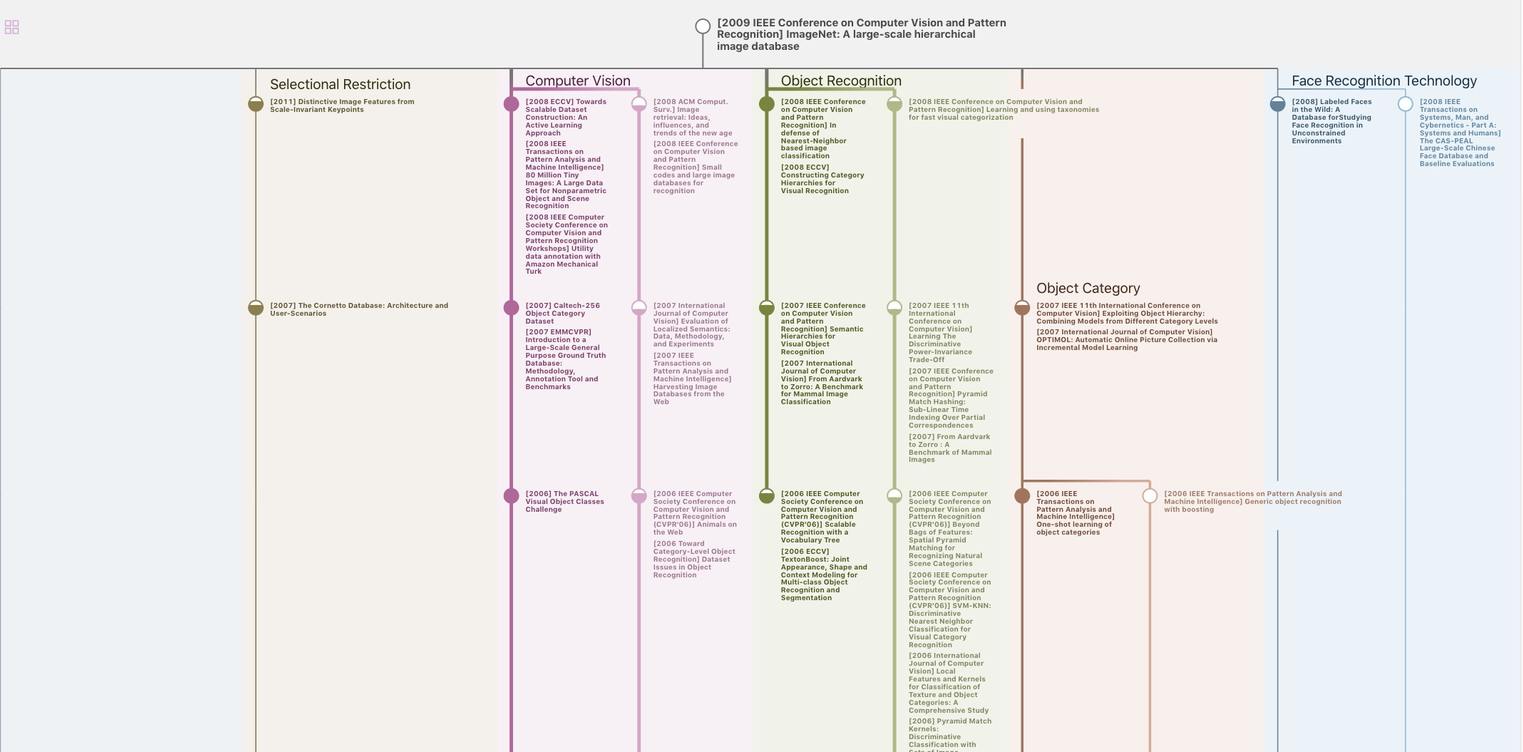
生成溯源树,研究论文发展脉络
Chat Paper
正在生成论文摘要