GAN-Place: Advancing Open-Source Placers to Commercial-Quality using Generative Adversarial Networks and Transfer LearningJust Accepted
ACM Transactions on Design Automation of Electronic Systems(2023)
摘要
Recently, GPU-accelerated placers such as DREAMPlace and Xplace have demonstrated their superiority over traditional CPU-reliant placers by achieving orders of magnitude speed up in placement runtime. However, due to their limited focus in placement objectives (e.g., wirelength and density), the placement quality achieved by DREAMPlace or Xplace is not comparable to that of commercial tools. In this paper, to bridge the gap between open-source and commercial placers, we present a novel placement optimization framework named GAN-Place that employs generative adversarial learning to transfer the placement quality of the industry-leading commercial placer, Synopsys ICC2, to existing open-source GPU-accelerated placers (DREAMPlace and Xplace). Without the knowledge of the underlying proprietary algorithms or constraints used by the commercial tools, our framework facilitates transfer learning to directly enhance the open-source placers by optimizing the proposed differentiable loss that denotes the “similarity” between DREAMPlace- or Xplace-generated placements and those in commercial databases. Experimental results on 7 industrial designs not only show the our GAN-Place immediately improves the Power, Performance, and Area (PPA) metrics at the placement stage, but also demonstrate that these improvements last firmly to the post-route stage, where we observe improvements by up to 8.3% in wirelength, 7.4% in power, and 37.6% in Total Negative Slack (TNS) on a commercial CPU benchmark.
更多查看译文
关键词
Placement Optimization,Generative Adversarial Learning,Transfer Learning
AI 理解论文
溯源树
样例
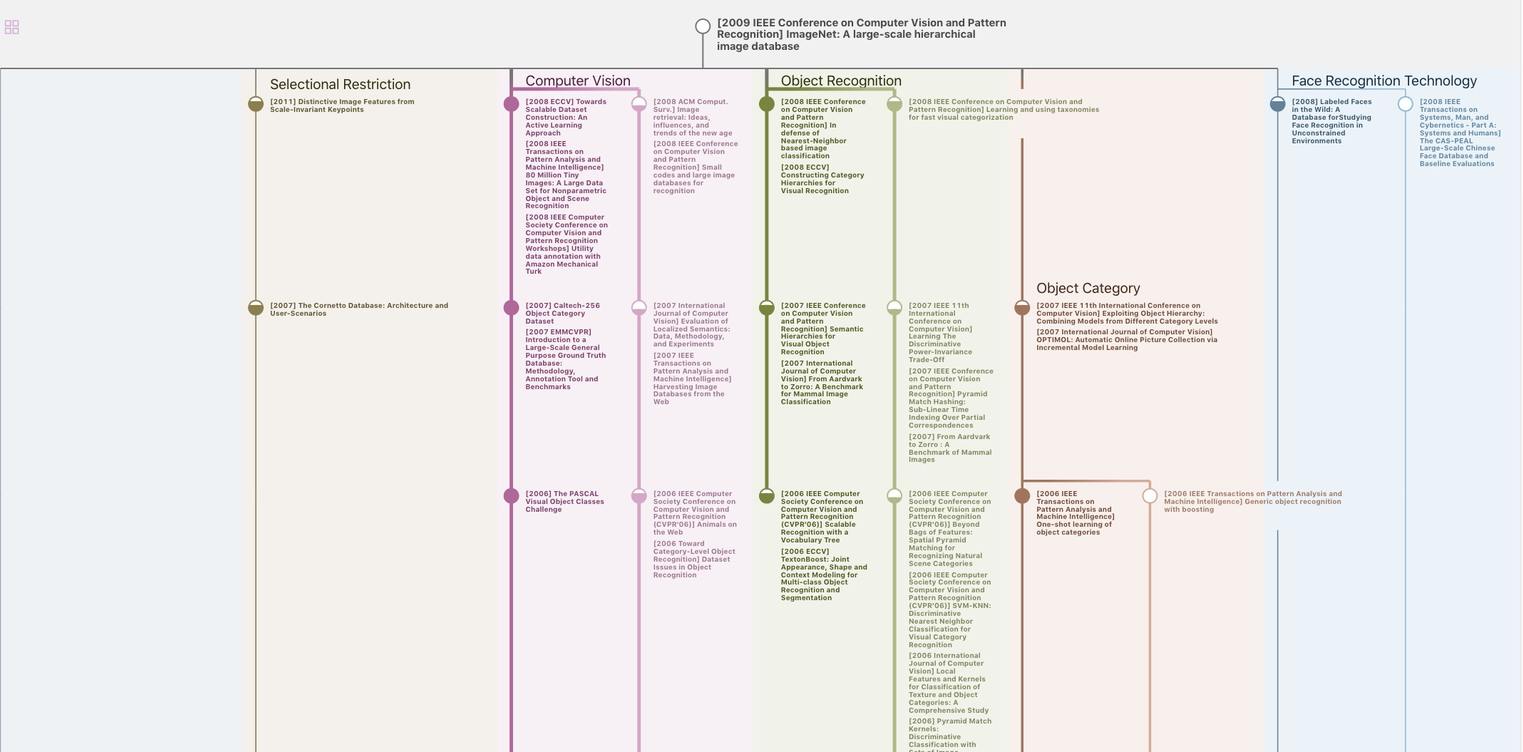
生成溯源树,研究论文发展脉络
Chat Paper
正在生成论文摘要