Detecting Deepfake Videos Using Spatiotemporal Trident NetworkJust Accepted
ACM Transactions on Multimedia Computing, Communications, and Applications(2023)
摘要
The widespread dissemination of Deepfake in social networks has posed serious security risks, thus necessitating the development of an effective Deepfake detection technique. Currently, video-based detectors have not been explored as extensively as image-based detectors. Most existing video-based methods only consider temporal features without combining spatial features, and do not mine deeper-level subtle forgeries, resulting in limited detection performance. In this paper, a novel spatiotemporal trident network (STN) is proposed to detect both spatial and temporal inconsistencies of Deepfake videos. Since the large amount of redundant information in Deepfake video frames, we introduce convolutional block attention module (CBAM) on the basis of the I3D network and optimize the structure to make the network better focus on the meaningful information of the input video. Aiming at the defects in the deeper-level subtle forgeries, we designed three feature extraction modules (FEMs) of RGB, optical flow, and noise to further extract deeper video frame information. Extensive experiments on several well-known datasets demonstrate that our method has promising performance, surpassing several state-of-the-art Deepfake video detection methods.
更多查看译文
关键词
Deepfake,spatiotemporal,trident networks,feature extraction module
AI 理解论文
溯源树
样例
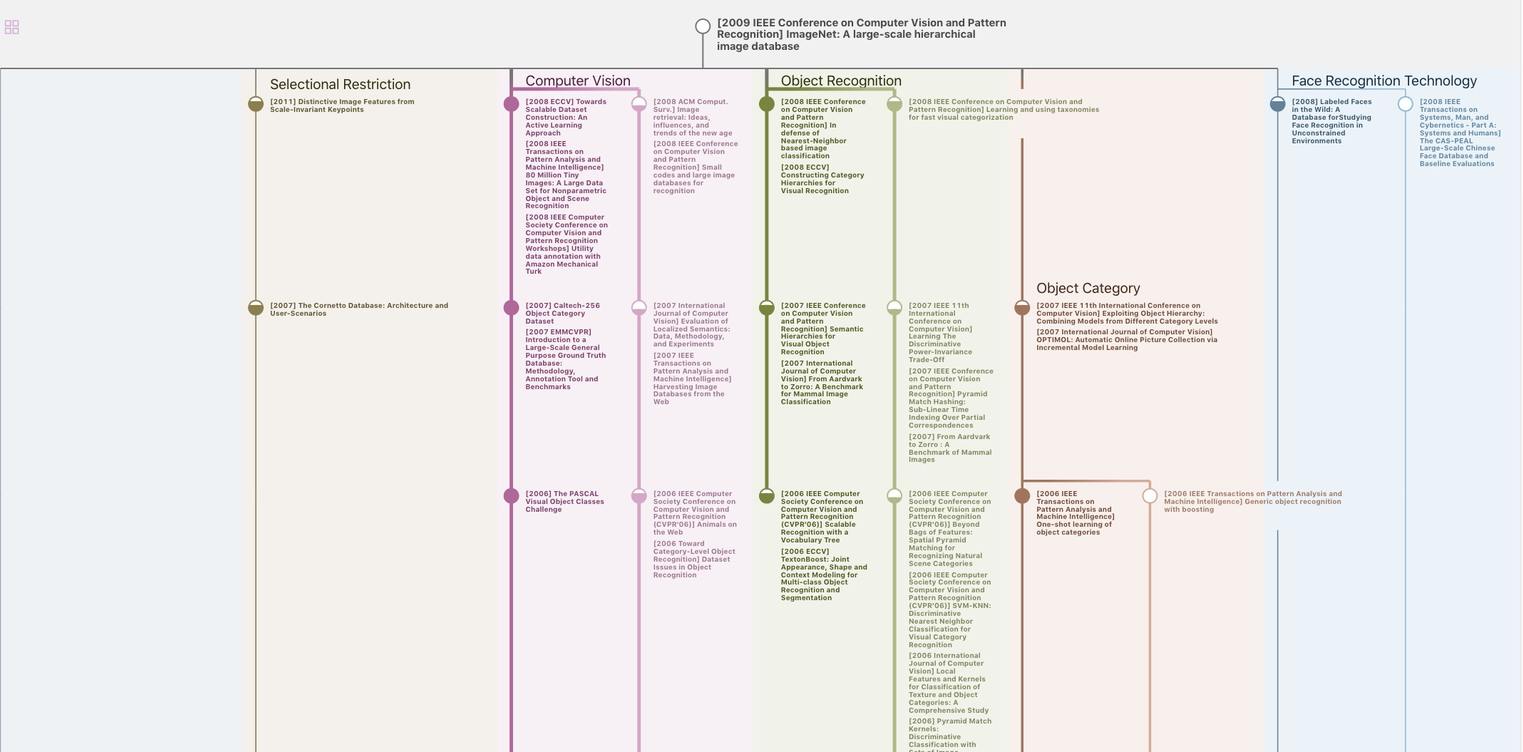
生成溯源树,研究论文发展脉络
Chat Paper
正在生成论文摘要