TESS: multivariate sensor time series prediction for building sustainable smart citiesJust Accepted
ACM Transactions on Sensor Networks(2022)
摘要
Time series analysis can help build sustainable smart cities with high energy efficiency. Smart cities contain a large number of sensors. The sensor data are mostly complex time series, such as traffic, electricity, and gas emission data. Accurate time series prediction can greatly help reduce energy consumption and gas emission. It is difficult to make accurate time series prediction because there are complex correlations among time series and the data have local and global nonlinear dependencies. To overcome the difficulties, we first analyze state-of-the-art works of time series prediction. Then we propose a new framework for Time series prediction evaluation for Energy conServation and emission reduction in Smart cities, called TESS. TESS is used to compare the latest time series prediction models based on six publicly available datasets and three simulation datasets. Based on the theoretical analysis and the experiment result, we get some interesting ideas: time series in smart cities often have obvious periodicity and scale changes, and need to be collected over the long term; skip-connection is helpful for learning long-term features of time series; the recurrent layer is good at predicting time series with obvious periodicity; and linear regression may be more appropriate for predicting time series with scale changes.
更多查看译文
关键词
deep learning,smart city,energy conservation,traffic prediction,electricity consumption prediction,multivariate time series prediction
AI 理解论文
溯源树
样例
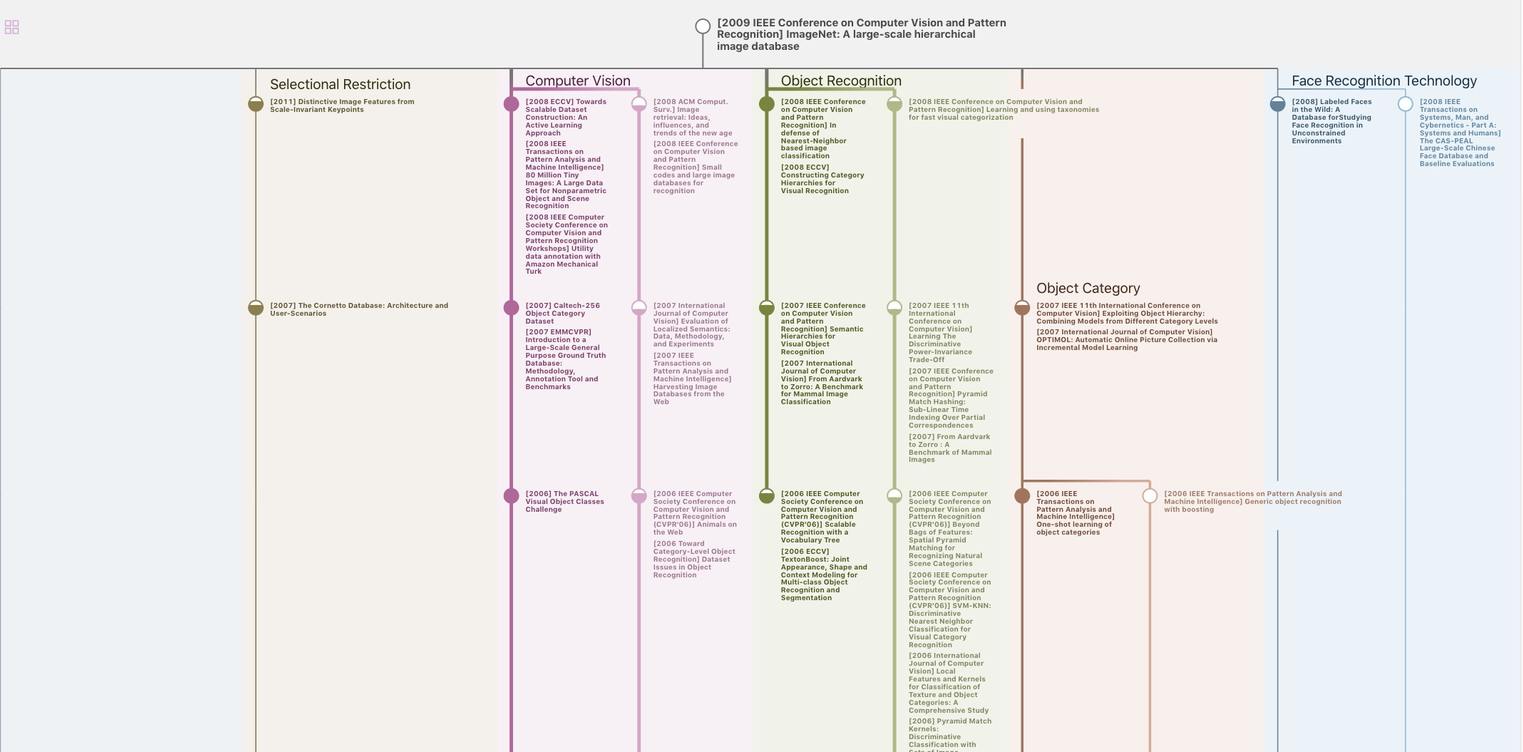
生成溯源树,研究论文发展脉络
Chat Paper
正在生成论文摘要