A data and physical model joint driven method for lithium-ion battery remaining useful life prediction under complex dynamic conditions
JOURNAL OF ENERGY STORAGE(2024)
摘要
Accurate remaining useful life (RUL) prediction of batteries plays an important role in battery management. The existing methods mainly rely on the battery test data under ideal operating conditions to show good performance. However, the actual operating conditions of batteries are usually complex, which bring great challenges to the RUL prediction. To improve the accuracy and the generalization ability of RUL prediction method, a fusion method of electrochemical-thermal model (ECT) and unscented kalman filter (UKF) is proposed for lithium-ion battery. Firstly, an ECT-based capacity degradation model is established by coupling pseudo two dimensions (P2D) electrochemistry model, 3D thermal model and solid electrolyte interface (SEI) formation model. Secondly, the UKF method is used to update iteratively the parameters of model to improve its accuracy. Finally, several sets of operating data of lithium-ion battery under different conditions are used for RUL prediction, followed by the comparative analysis of different models and algorithms. As a result, the proposed fusion method not only exhibits better accuracy in RUL prediction under ideal conditions, but also shows excellent accuracy and generalization for other dynamic stochastic operating conditions.
更多查看译文
关键词
Lithium-ion battery,Remaining useful life prediction,Electrochemical-thermal model,Unscented kalman filter,Dynamic operating conditions
AI 理解论文
溯源树
样例
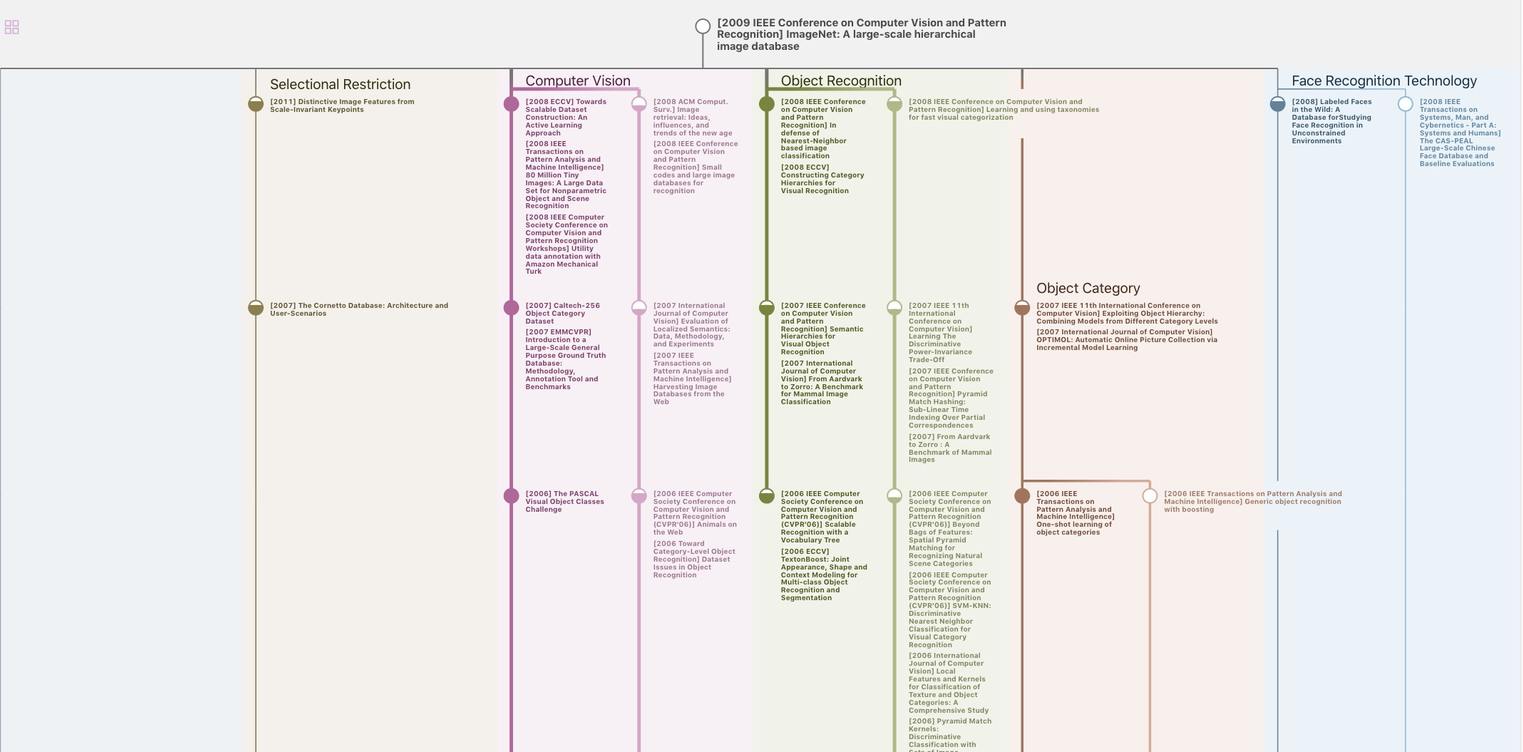
生成溯源树,研究论文发展脉络
Chat Paper
正在生成论文摘要