Prediction of Biodiesel Yield Employing Machine Learning: Interpretability Analysis via Shapley Additive Explanations
FUEL(2024)
摘要
Bioenergy is a sustainable alternative to conventional energy sources. However, production is limited by the complexities of the process and is also time consuming. Recent advancements in artificial intelligence and machine learning offer promising opportunities for streamlining bioenergy conversion processes. This paper aimed to develop a generic model for predicting biodiesel yield percentages from various biomass derived feedstocks through transesterification reactions. The biodiesel yield data and relevant features and properties of the feedstocks are collected from open literature sources. This work mainly focuses on establishing a generic model framework for biodiesel yield prediction. This involves data preprocessing techniques, machine learning model selection, and hyperparameter tuning. The grid search cross-validation was employed to optimize the CatBoost regressor, resulting in a prediction model with a root mean squared error value of 4.288. Moreover, the study conducted SHapley Additive exPlanations analysis to gain insights into the influence of individual data points on the prediction model. This analysis provided valuable insights into the impact of different variables on biodiesel yield prediction. Overall, this research presents a comprehensive approach to predicting biodiesel yield using machine learning techniques and highlights the effectiveness of the whale optimization algorithm for model optimization. The findings contribute to advancing the understanding of the factors influencing biodiesel production and provide valuable insights for optimizing the transesterification process.
更多查看译文
关键词
Biodiesel prediction,Machine learning,Modeling,Hyperparameters tuning,SHapley Additive exPlanation analysis,Optimization
AI 理解论文
溯源树
样例
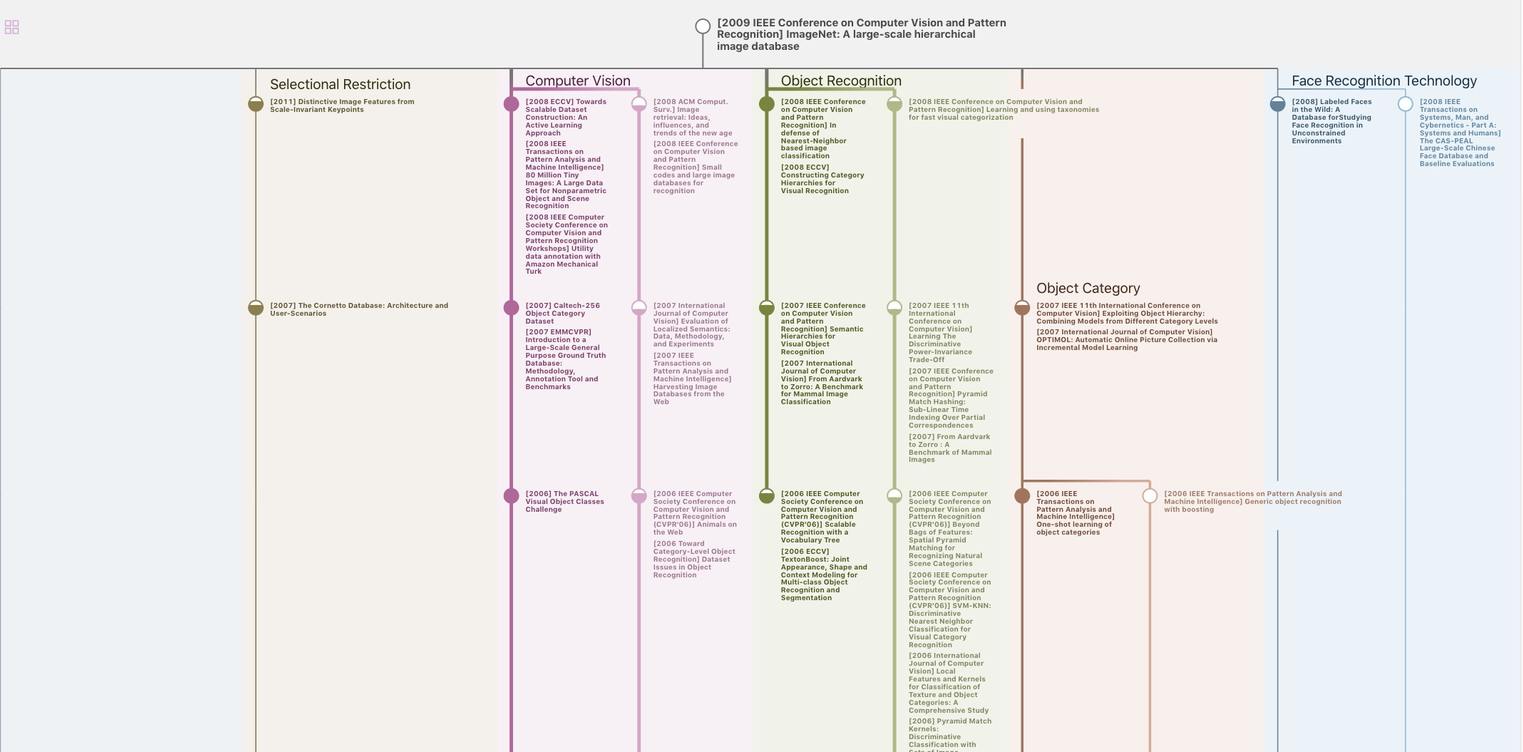
生成溯源树,研究论文发展脉络
Chat Paper
正在生成论文摘要