Combining artificial neural networks and genetic algorithms to model nitrate contamination in groundwater
Natural Hazards(2024)
摘要
Increasing the concentration of nitrates in aquifer systems reduces water quality and causes serious diseases and complications for human health. Therefore, it is important to monitor nitrate levels in groundwater resources and identify contaminated aquifers. In this research, multiple artificial neural network (ANN) structures and a genetic algorithm (GA) were combined to predict groundwater nitrate levels using its affecting factors in Mazandaran plain (north of Iran). Five ANN algorithms were trained, and their performances were evaluated during the training, cross-validating, and testing stages. Then, GA was combined with the ANNs, and the process of training, cross-validation, and testing was repeated with the same data. The results showed the factors of distance from industrial centers (R = − 0.57), population density (R = 0.56), and groundwater depth (R = − 0.15) are the most important factors in groundwater nitrate contamination. Further, a modular neural network (MNN) model showed the highest performance among the networks used in nitrate concentration modeling. Additionally, combining ANNs with GA enhanced the performance of the models in predicting nitrate concentration. The MSE and R-srqr of the MNN model in the test stage were estimated to be 0.2 and 0.7, respectively. After combining with GA, these values were improved to 0.1 and 0.8, respectively. Finally, the zoning map of nitrate pollution in groundwater was prepared using the ANN-GA hybrid model in the GIS environment. The cost-effective methodology presented in this study can be used to predict the spatial and temporal changes of nitrates in the study area and other areas with similar settings.
更多查看译文
关键词
Nitrate contamination,Training,Optimization,Testing,Pollution zoning map,Mazandaran plain
AI 理解论文
溯源树
样例
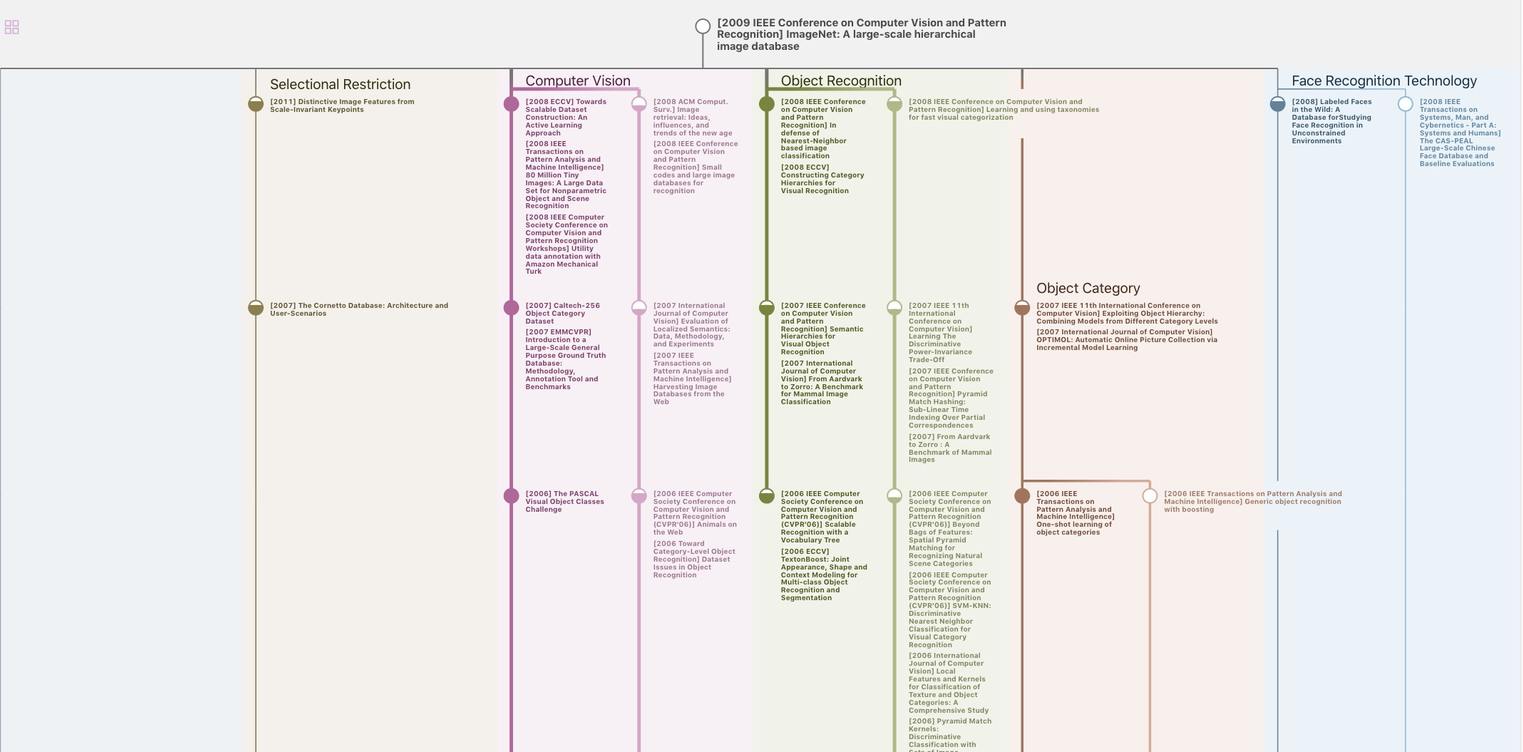
生成溯源树,研究论文发展脉络
Chat Paper
正在生成论文摘要