Optimal redundant transmission scheduling for remote state estimation via reinforcement learning approach
NEUROCOMPUTING(2024)
摘要
This paper studies the optimal redundant transmission scheduling for remote state estimation. Multiple smart sensors observe some systems, and transmit the local state estimates via independent channels to a remote estimator (RE), where packet losses may occur with some particular probabilities. To improve the estimation performance, some redundant channels are adopted for the data transmission. Since the number of redundant channels is fixed, the optimal redundant scheduling for multiple sensors is worth investigating to determine how to allocate the redundant channels. To address this problem, the redundant transmission scheduling is modeled as a Markov decision process (MDP) to minimize the estimation error for all systems. By constructing a sufficient condition, one ensures that the MDP has an optimal deterministic and stationary policy. Meanwhile, the threshold structure of the redundant transmission scheduling policy is verified to further decrease the complexity of the calculation. Reinforcement learning (RL) is used for this problem, and a near -optimal policy is obtained by dueling double -deep Q -networks (D3QN) algorithm. Finally, an illustrative simulation is presented to demonstrate its effectiveness.
更多查看译文
关键词
Redundant transmission scheduling,Remote state estimation,Markov decision process,Reinforcement learning
AI 理解论文
溯源树
样例
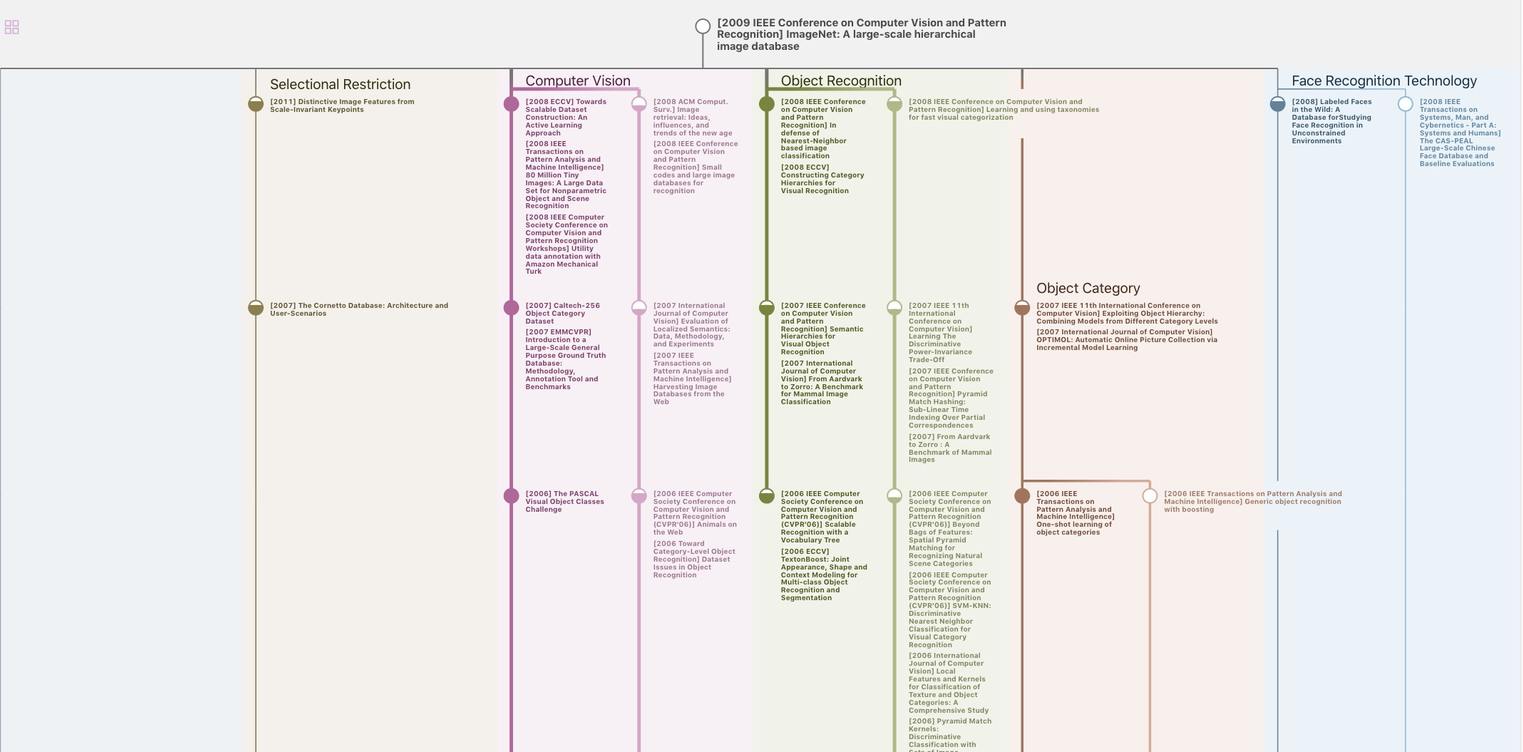
生成溯源树,研究论文发展脉络
Chat Paper
正在生成论文摘要