Unsupervised denoising for seismic data with complementary mask blind spot strategy
JOURNAL OF APPLIED GEOPHYSICS(2024)
摘要
Attenuation of incoherent noise can effectively improve signal-to-noise ratio (SNR) of seismic data. Recently, unsupervised denoising methods have emerged as solutions for the data collection problem to learn directly from single noisy data. However, the loss of input information based on their blind spot strategy leads to poor denoising performance. In this paper, we propose a complementary mask unsupervised learning framework based on visible blind spot (CMVB) to overcome the information loss. First, a Splitter-Splicer is designed to act on the complementary mask of the input. This approach makes full use of all the data to preserve weak signals. Second, a new blind -spot -based loss function is proposed to integrate blind -spot and non -blind -spot data. The addition of non -blind -spot data enhances the continuity of seismic events. Finally, the attention mechanism is integrated into the encoder -decoder structure, improving the ability of blind spot networks to capture detailed information. Experiments are conducted on three synthetic datasets and two field datasets to compare with two existing state-of-the-art unsupervised denoising methods. The results demonstrate that our method excels in recovering weak reflections.
更多查看译文
关键词
Deep learning,Denoising,Seismic data,Unsupervised learning
AI 理解论文
溯源树
样例
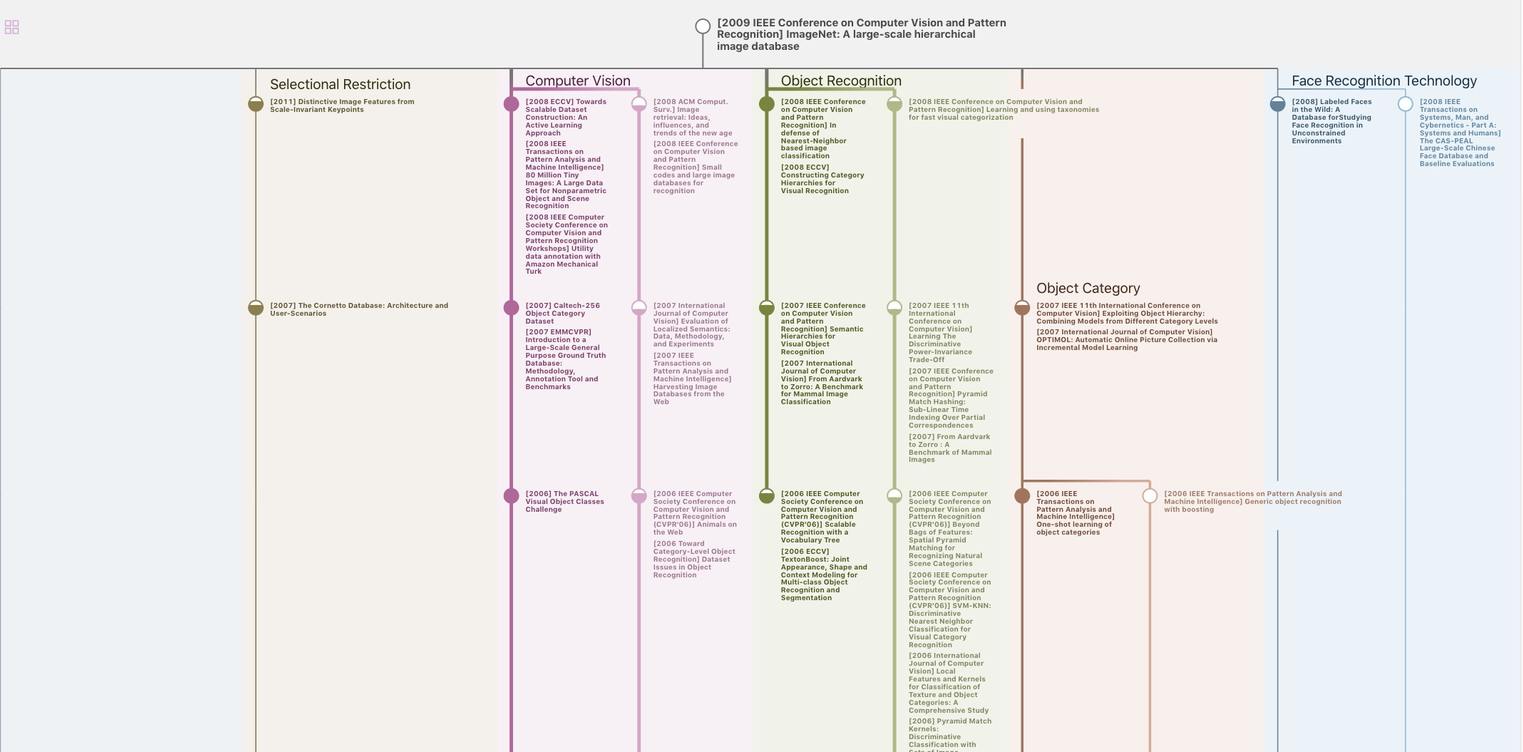
生成溯源树,研究论文发展脉络
Chat Paper
正在生成论文摘要