When Average Isn't Good Enough: Identifying Meaningful Subgroups in Clinical Data
Cognitive Therapy and Research(2024)
摘要
Background Clinical data are usually analyzed with the assumption that knowledge gathered from group averages applies to the individual. Doing so potentially obscures patients with meaningfully different trajectories of therapeutic change. Needed are “idionomic” methods that first examine idiographic patterns before nomothetic generalizations are made. The objective of this paper is to test whether such an idionomic method leads to different clinical conclusions. Methods 51 patients completed weekly process measures and symptom severity over a period of eight weeks. Change trajectories were analyzed using a nomothetic approach and an idiographic approach with bottom-up clustering of similar individuals. The outcome was patients’ well-being at post-treatment. Results Individuals differed in the extent that underlying processes were linked to symptoms. Average trend lines did not represent the intraindividual changes well. The idionomic approach readily identified subgroups of patients that differentially predicted distal outcomes (well-being). Conclusions Relying exclusively on average results may lead to an oversight of intraindividual pathways. Characterizing data first using idiographic approaches led to more refined conclusions, which is clinically useful, scientifically rigorous, and may help advance individualized psychotherapy approaches.
更多查看译文
关键词
Intraindividual differences,Idionomic analysis,Nomothetic,Processes
AI 理解论文
溯源树
样例
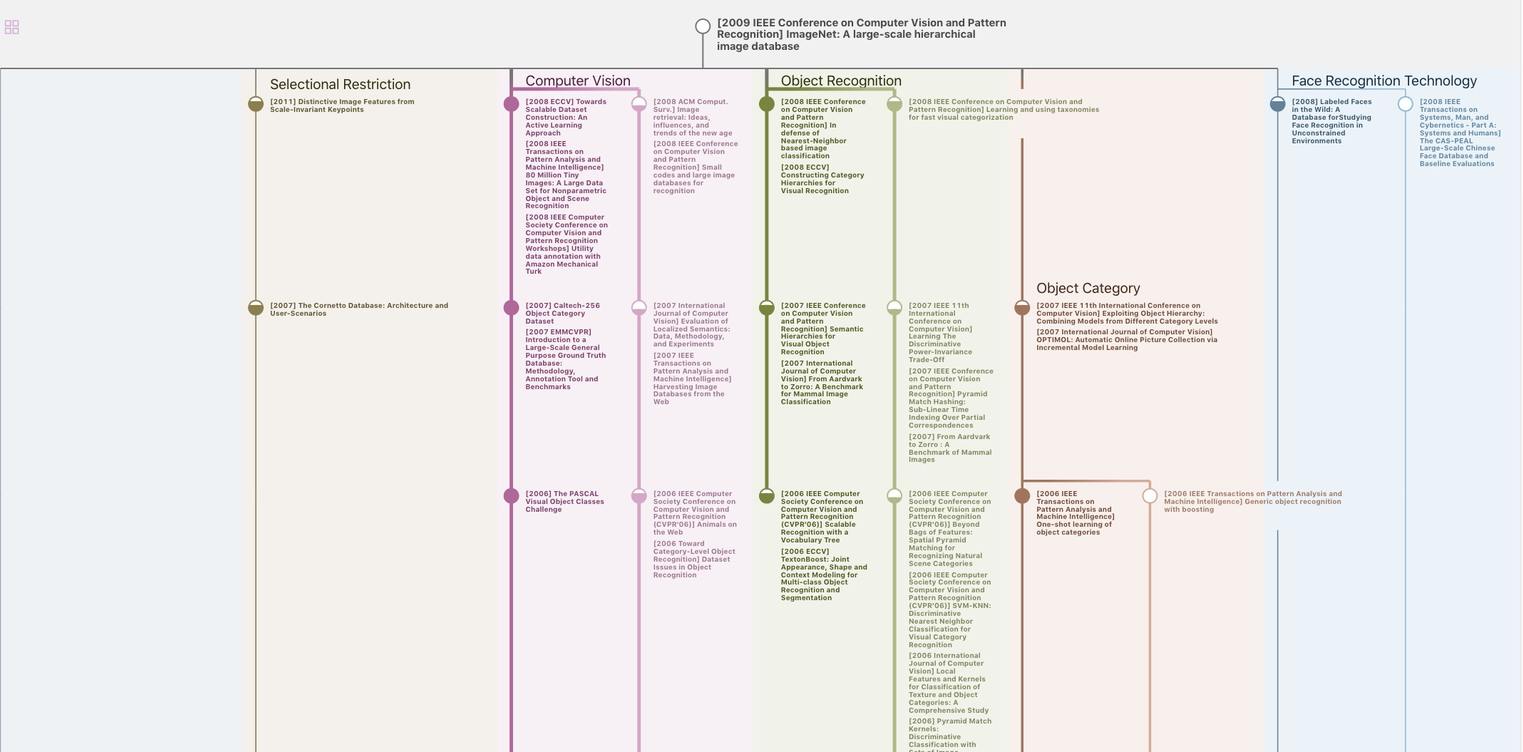
生成溯源树,研究论文发展脉络
Chat Paper
正在生成论文摘要