A cascading elimination-based evolutionary algorithm with variable classification mutation for many-objective optimization
INFORMATION SCIENCES(2024)
摘要
Many -objective evolutionary algorithms have gained significant achievements over the years. However, the difficulty in balancing convergence and diversity of the population remains. In this paper, we propose a cascading elimination based evolutionary algorithm with variable classification mutation, termed CEEA, for many -objective optimization. In CEEA, a cascading elimination mechanism based on the binary quality indicator and balanceable fitness estimation (BFE) is proposed for eliminating poor individuals one by one and further balancing convergence and diversity of the population. To be specific, two individuals with the minimum binary quality indicator value are found from the population, where these two individuals may exist in the dominance relation and have more similar search directions. If the relation of two selected individuals is dominance, the dominated individual is eliminated. Otherwise, one individual is eliminated by the BFE method. In addition, a binary quality indicator based variable classification mutation strategy is developed to produce promising individuals, and further improve the search efficiency of CEEA. Experimental studies on three well-known benchmark test suites, a combinational optimization problem, and a real -world engineering application have demonstrated that our CEEA has a superior performance to its peer competitors on various manyobjective problems.
更多查看译文
关键词
Evolutionary algorithms,Many-objective optimization,Cascading elimination mechanism,Binary quality indicator,Balanceable fitness estimation
AI 理解论文
溯源树
样例
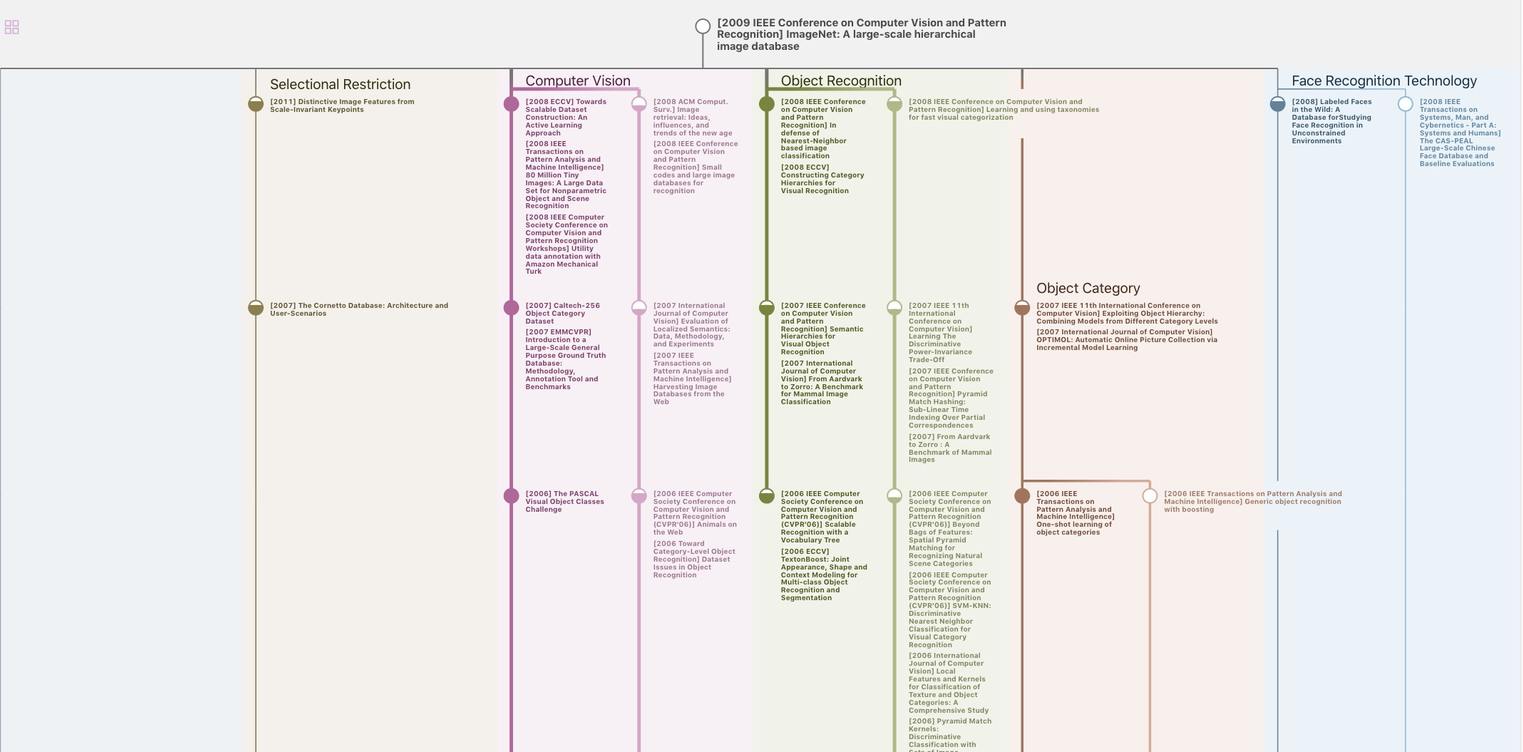
生成溯源树,研究论文发展脉络
Chat Paper
正在生成论文摘要