Constraining uncertainty in boron isotope systematics using a Bayesian inversion engine reveals contrasting parameter sensitivities
CHEMICAL GEOLOGY(2024)
摘要
Physical parameters within boron isotope systematics form a complex interplay that determine the boron isotopic composition of rocks, minerals, and fluids, but to date, providing constraints on uncertainty within boron equilibrium isotope modelling remains elusive. This underlying uncertainty limits the potency of boron isotopes as a tool for detecting fluid-rock exchange. A new equilibrium boron mineral-fluid fractionation modelling approach, named EquiB, coupled with a Bayesian inversion engine is presented, providing robust and reproducible constraints on the uncertainty of physical parameters encoded into a boron isotopic composition of a rock in equilibrium with a fluid. We demonstrate the validity of our approach by applying the model to several basaltfluid and peridotite-fluid exchange scenarios. The model output generates multi-dimensional posterior probability distributions that show temperature is the greatest control on mineral-fluid fractionation in all applied scenarios. At high temperatures (defined as >50 degree celsius) pH-dependent fractionation is negligible, but at low temperatures (defined as <50 degree celsius) pH-dependent fractionation is a control on boron isotopic compositions. At geologically reasonable conditions other parameters such as salinity, fluid density, and pressure have little effect on the extent of boron mineral-fluid fractionation. Model outputs agree with experimentally derived fractionation factors at typical hydrothermal conditions but diverge at low temperatures. This approach provides robust constraints of parameter uncertainty, enabling meaningful interpretation of boron isotope analyses and the ability to fingerprint isotopic compositions with greater confidence.
更多查看译文
关键词
Boron isotopes,Serpentinization,Bayesian inversion,Fluid -rock exchange,Equilibrium isotope fractionation,Parameter estimation
AI 理解论文
溯源树
样例
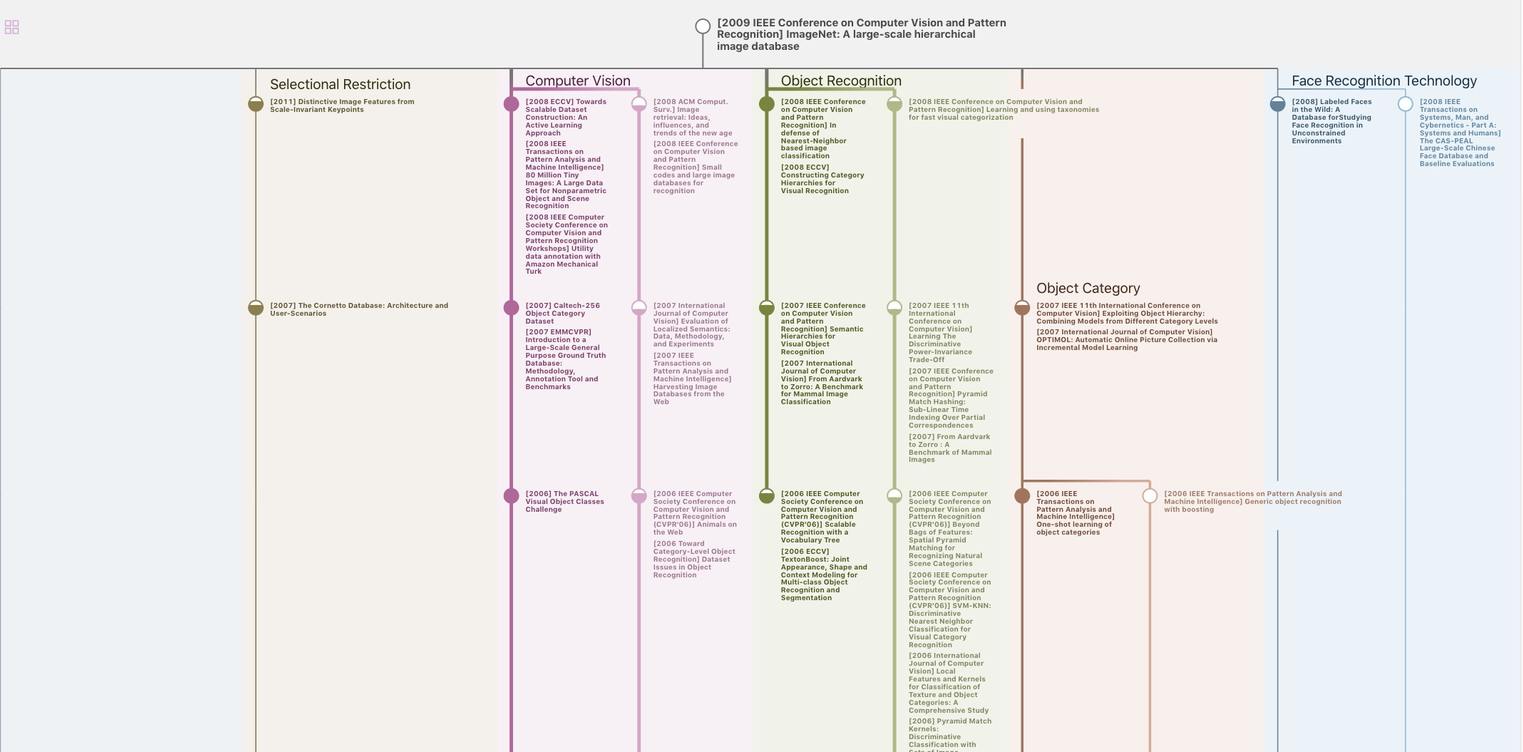
生成溯源树,研究论文发展脉络
Chat Paper
正在生成论文摘要