ATT-LSTM: An Interpretable Deep Learning Framework for COVID Outbreak Prediction.
International Conference of Distributed Computing and Networking(2024)
Abstract
The outbreak of the new coronavirus (COVID-19), which causes respiratory infections in humans, has been a significant concern worldwide since December 2019. As of September 12, 2020, there had been 1 million reported deaths worldwide and 29 million certified cases. The urgent need to observe and predict the distribution of COVID-19 has been found to manage this spread. Among the various popular models of the COVID-19 forecast, statistical models have received a lot of media attention. However, it has been found that these statistical models have shown little accuracy with long-term forecasts due to insufficient data available and high levels of uncertainty. In this paper, we propose a comparison-based analysis of various deep learning models to predict the prevalence of COVID-19 as a more effective alternative to statistical methods. We proposed a new attention-based encoder-decoder model called Attention-Long Short-Term Memory (ATT-LSTM). The LSTM-based neural network layer architecture uses the idea of a fine-grained attention mechanism, which means paying attention to the hidden state rather than the hidden state vectors themselves. This can draw attention to the importance and contributions of each hidden state dimension. It is useful in locating and concentrating relevant temporary data, leading to a distinctly interpretable network. A learnable vector embedded for time has been implemented because time in a vector representation can be easily added with one or more architectures. This vector representation is known as Time2Vec. The proposed deep learning model predicts the trend and patterns of COVID-19 outbreaks in some of the worst-affected European countries, such as Italy, Spain, France, and Canada. We have used the COVID-19 database by Johns Hopkins University’s Center for Systems Science and Engineering (CSSE) to test and analyze the proposed model. The proposed model has higher predictive accuracy compared to other existing models.
MoreTranslated text
AI Read Science
Must-Reading Tree
Example
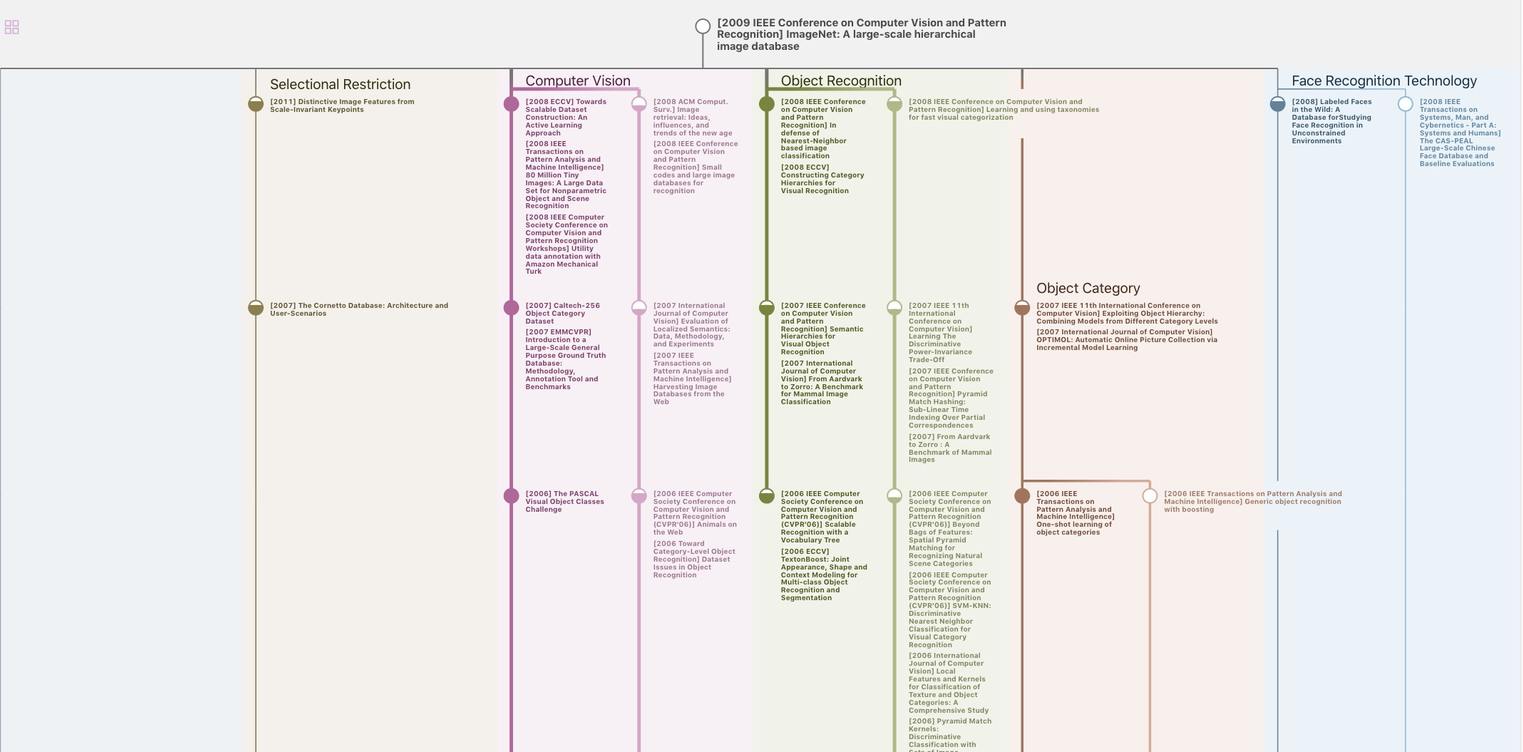
Generate MRT to find the research sequence of this paper
Chat Paper
Summary is being generated by the instructions you defined