Empirical Risk-aware Machine Learning on Trojan-Horse Detection for Trusted Quantum Key Distribution Networks
arxiv(2024)
摘要
Quantum key distribution (QKD) is a cryptographic technique that leverages
principles of quantum mechanics to offer extremely high levels of data security
during transmission. It is well acknowledged for its capacity to accomplish
provable security. However, the existence of a gap between theoretical concepts
and practical implementation has raised concerns about the trustworthiness of
QKD networks. In order to mitigate this disparity, we propose the
implementation of risk-aware machine learning techniques that present risk
analysis for Trojan-horse attacks over the time-variant quantum channel. The
trust condition presented in this study aims to evaluate the offline assessment
of safety assurance by comparing the risk levels between the recommended safety
borderline. This assessment is based on the risk analysis conducted.
Furthermore, the proposed trustworthy QKD scenario demonstrates its numerical
findings with the assistance of a state-of-the-art point-to-point QKD device,
which operates over optical quantum channels spanning distances of 1m, 1km, and
30km. Based on the results from the experimental evaluation of a 30km optical
connection, it can be concluded that the QKD device provided prior information
to the proposed learner during the non-existence of Eve's attack. According to
the optimal classifier, the defensive gate offered by our learner possesses the
capability to identify any latent Eve attacks, hence effectively mitigating the
risk of potential vulnerabilities. The Eve detection probability is provably
bound for our trustworthy QKD scenario.
更多查看译文
AI 理解论文
溯源树
样例
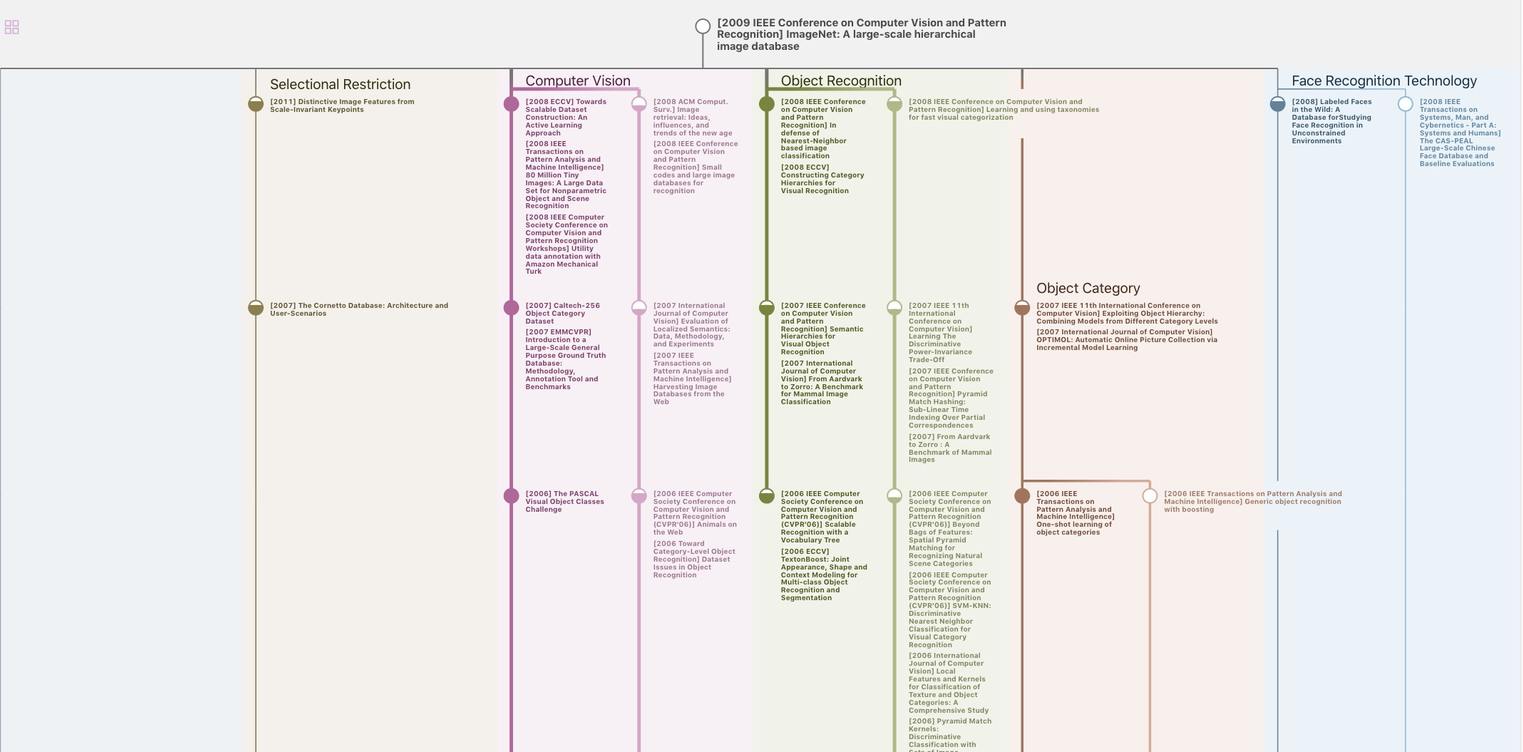
生成溯源树,研究论文发展脉络
Chat Paper
正在生成论文摘要