A deep neural network physics-based reduced order model for dynamic stall
arxiv(2024)
摘要
Dynamic stall is a challenging fluid dynamics phenomenon occurring during
rapid transient motion of airfoils where the angle of attack exceeds the static
stall angle. Understanding dynamic stall is essential for designing efficient
aerodynamic systems in applications such as helicopters, wind turbines, and
specific aircraft maneuvers. The highly non-linear unsteady aerodynamic
phenomena involved in dynamic stall are challenging to be included in a reduced
order model. In this work, a physics-based machine learning framework is
developed to fast predict the aerodynamic forces and momentum for a pitching
NACA0012 airfoil incurring in light and deep dynamic stall. Three deep neural
network architectures of increasing complexity are investigated, ranging from
two multilayer perceptrons to a convolutional neural network. The proposed
model is able to robustly predict pressure and skin friction distributions over
the airfoil for an entire pitching cycle. Periodic conditions are implemented
to grant the physical smoothness of the solution both in space and time.
Furthermore, an improved loss function incorporating physical knowledge to
compute the airfoil loads is presented. An analysis of the dataset point
distributions to cover the flight envelope is performed highlighting the
effects of adopting low discrepancy sequences, like Latin hypercube, Sobol',
and Halton, compared to random and uniform grid ones. The current model shows
unprecedented performances in predicting forces and momentum in a broad range
of flight conditions.
更多查看译文
AI 理解论文
溯源树
样例
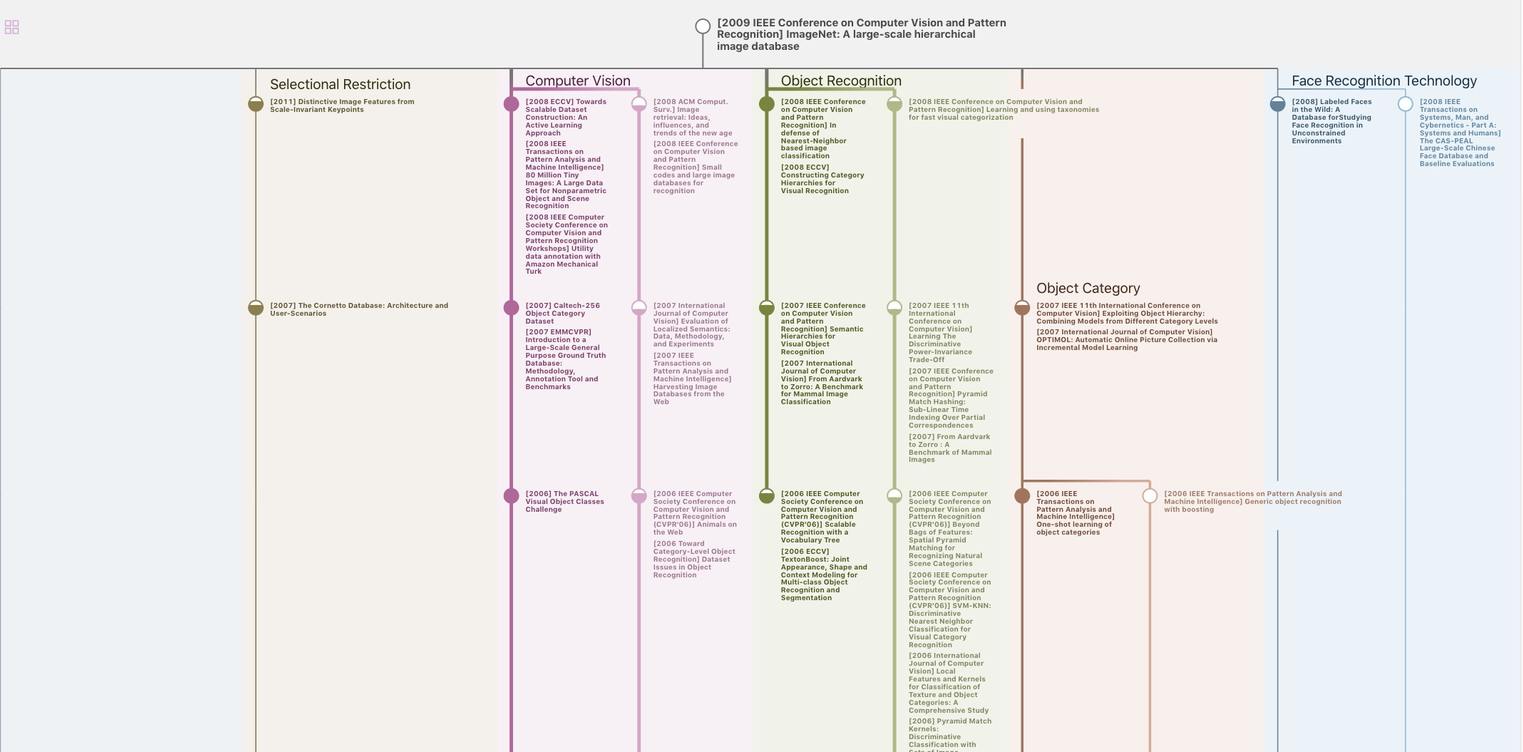
生成溯源树,研究论文发展脉络
Chat Paper
正在生成论文摘要