Improving Wheat Leaf Disease Image Classification with Point Rend Segmentation Technique
SN Computer Science(2024)
Abstract
The biggest contribution to the Indian economy is made through agriculture. It is one of the main sources of income for farmers. The major concern for the farmers during cultivation is to produce crops of good quality and quantity. For a wide range of productivity and protection from various diseases, several techniques must be revised. Machine learning techniques were utilized for the categorization of leaf diseases, because earlier methods were time-consuming and labor-intensive. Better results are achieved using machine learning techniques, along with certain limitations. Deep Convolutional Neural networks are now one of the finest methods for categorizing leaf diseases in crops. There are still issues with getting access to trustworthy models for identifying a variety of diseases and segmenting lesion areas for assessing severity in real-world field conditions, despite the growing popularity of deep learning approaches for detecting various diseases. Under Deep Convolutional Neural Networks, various architectures perform well for image classification. The quantitative evaluation of features that increase a plant’s ability to withstand disease is crucial in the selection of plant breeders. As a result, it is vital to take advantage of the disease-affected regions’ ability to determine how badly diseased the leaves are. To address these issues, CNN-based segmentation model was proposed to separate the wheat leaf diseases from the wheat leaf image dataset at the pixel level. This is superior to the segmentation algorithms currently in use. Our ultimate goal is to assist farmers in detecting and learning about early-stage illnesses in wheat leaves. To classify the disorders, a Deep Convolutional Neural Network was used. The investigation made use of a dataset including 4000 images of wheat leaves infected with three different types of leaf diseases: powdery mildew, leaf rust, and spot blotch. This manuscript includes the proposed method for feature extraction using the Point Rend deep segmentation model, followed by classification through the EfficientNet architecture. The results reveal that the proposed model is more accurate, showing a classification accuracy of 99.43% in comparison with classification performed on wheat leaf diseases without employing segmentation networks.
MoreTranslated text
Key words
Machine learning,Deep learning,Convolutional neural networks,Agriculture,Image processing
AI Read Science
Must-Reading Tree
Example
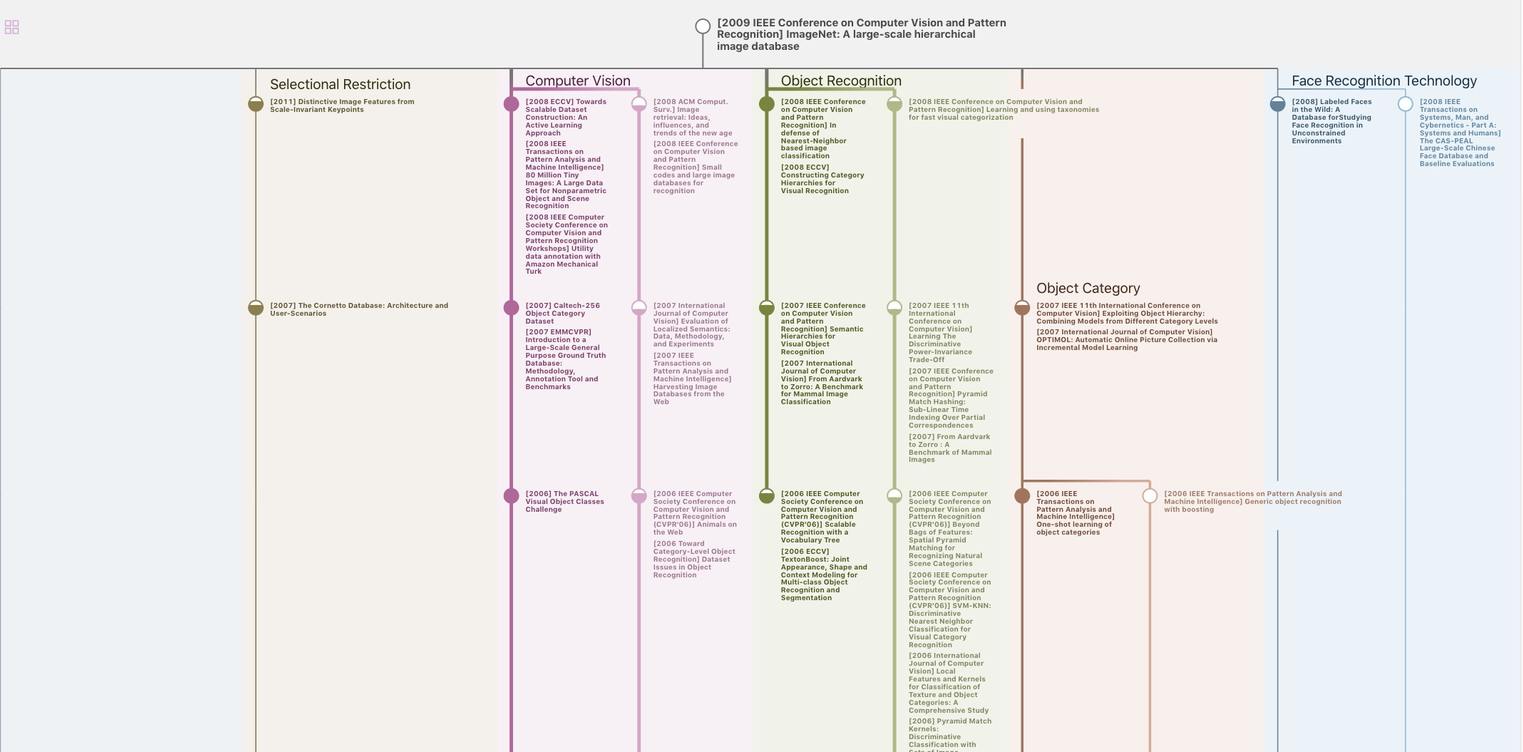
Generate MRT to find the research sequence of this paper
Chat Paper
Summary is being generated by the instructions you defined