Non-quadratic Regularization Parameters Selection for Model-based Geometrical Distortion Correction of MRI images
2022 IEEE Nuclear Science Symposium and Medical Imaging Conference (NSS/MIC)(2022)
摘要
Nowadays, MRI has received lots of attention as the only method of imaging in radiation therapy. However, MR images suffer from distortions induced by magnetic susceptibility effects that can be up to 2-4 mm at air-tissue interfaces, which is the main concern in precision radiation therapy planning. The conventional method for MR distortion correction is the field-mapping which is not able to completely correct distortions due to residual intensity and geometrical distortions in the field-map. To address this issue, a model-based reconstruction which has two regularization terms, one for image and the other for field-map correction was presented. Due to the edge smoothing effect of the quadratic penalty function, non-quadratic penalties were proposed and investigated for the model-based MR image reconstruction. In this regard, selecting proper regularization parameters for non-quadratic regularization is challenging due to the lack of prior knowledge about the ground truth image. This work set out to propose an approximation method for selecting optimized parameters for image and field-map regularization in the model-based reconstruction. To validate the proposed method, BrainWeb phantom simulation data was used. When the Huber and Lange1 penalty functions were employed, the estimated regularization parameters by the proposed method led to the minimum root mean square error (RMSE) and maximum peak signal-to-noise ratio (PSNR) for the reconstructed image versus the ground truth data. The results demonstrated a good agreement between the results obtained from the proposed method and the ground truth data. The simulation study demonstrated the effectiveness of the proposed method in reducing the MR image distortion for the purpose of precision radiation therapy planning.
更多查看译文
关键词
Regularization Parameter,Geometric Distortion,Magnetic Resonance Imaging,Root Mean Square Error,Mean Square Error,Imaging Methods,Image Reconstruction,Main Concern,Regularization Term,Penalty Function,Peak Signal-to-noise Ratio,Ground Truth Image,Model-based Reconstruction,Minimum Root Mean Square,Loss Function,Image Edge,True Image,Subset Of Values,Hyperfunction,K-space Data
AI 理解论文
溯源树
样例
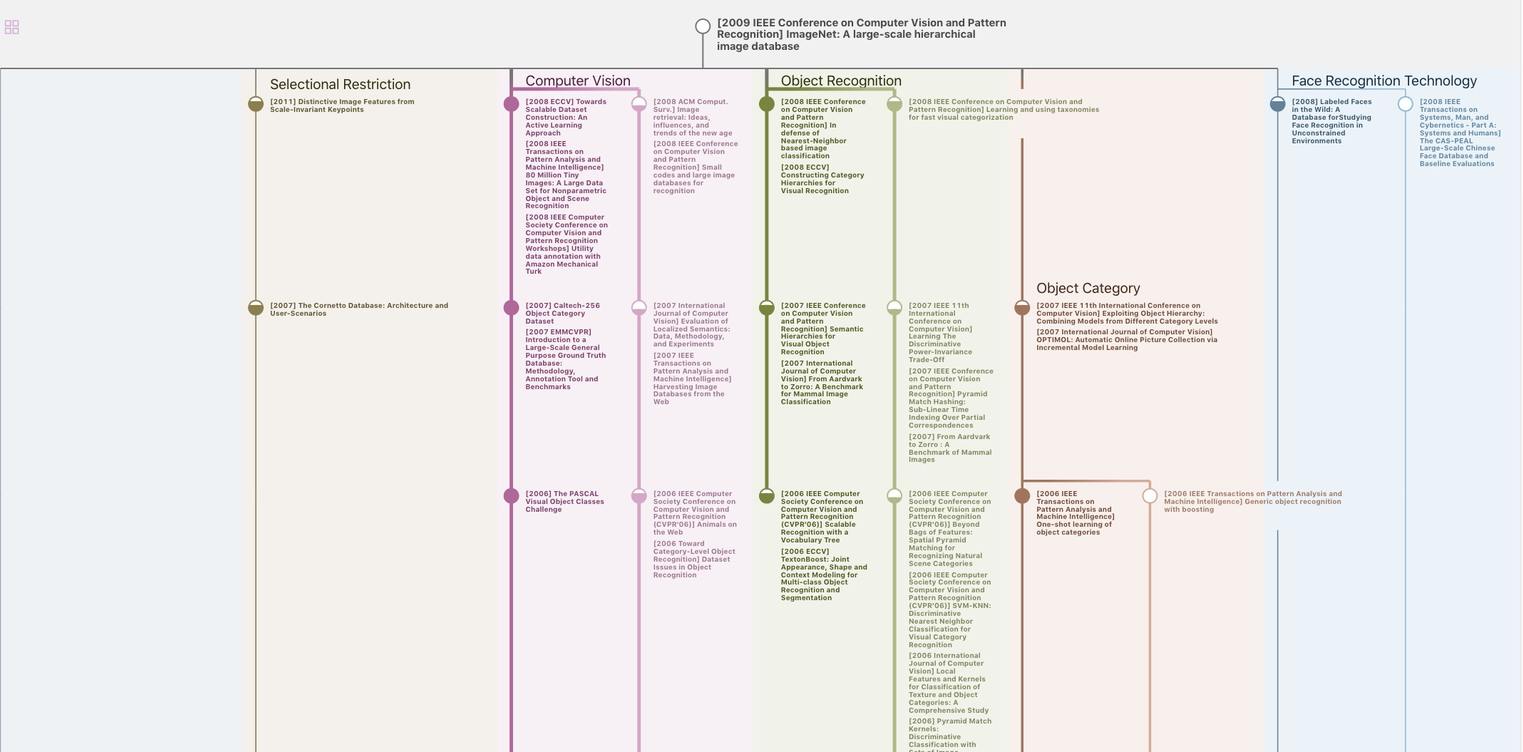
生成溯源树,研究论文发展脉络
Chat Paper
正在生成论文摘要