Augmented Deep Learning-Based Motion Estimation for Extreme Pose Tracking of Awake Rodents
2022 IEEE Nuclear Science Symposium and Medical Imaging Conference (NSS/MIC)(2022)
摘要
Motion-compensated PET imaging of awake rodents is an exciting experimental regime in molecular imaging, but it relies heavily on the challenging task of sampling an animal’s motion accurately. This work investigates the feasibility of deep neural-network (DNN) based motion tracking for this application. In particular, we address the situation of estimating extreme poses (i.e. edge cases), which can occur frequently in awake animal studies, but where there are likely to be few, if any, ground-truth poses for proper training of a model. A conditional generative adversarial network (GAN) with pix2pix architecture was used to generate realistic synthetic stereo views for arbitrary poses of the animal. When combined with a DNN-based regression approach to estimate six rigid-body motion parameters, we show that synthetic augmentation provides significant performance gains in accuracy: RMSE 0.23° and 0.19 mm for rotation and translation estimation, respectively. Our advanced augmented DL-based rigid-body motion estimation from multi-view stereo appears promising as a solution for extreme pose tracking.
更多查看译文
关键词
Motion Estimation,Pose Tracking,Extreme Poses,Awake Rodents,Deep Neural Network,Molecular Imaging,Generative Adversarial Networks,Motion Tracking,Rigid Body Motion,Conditional Generative Adversarial Network,Edge Cases,Multi-view Stereo,Linear Interpolation,Pose Estimation,Synthetic Generation,Rat Head
AI 理解论文
溯源树
样例
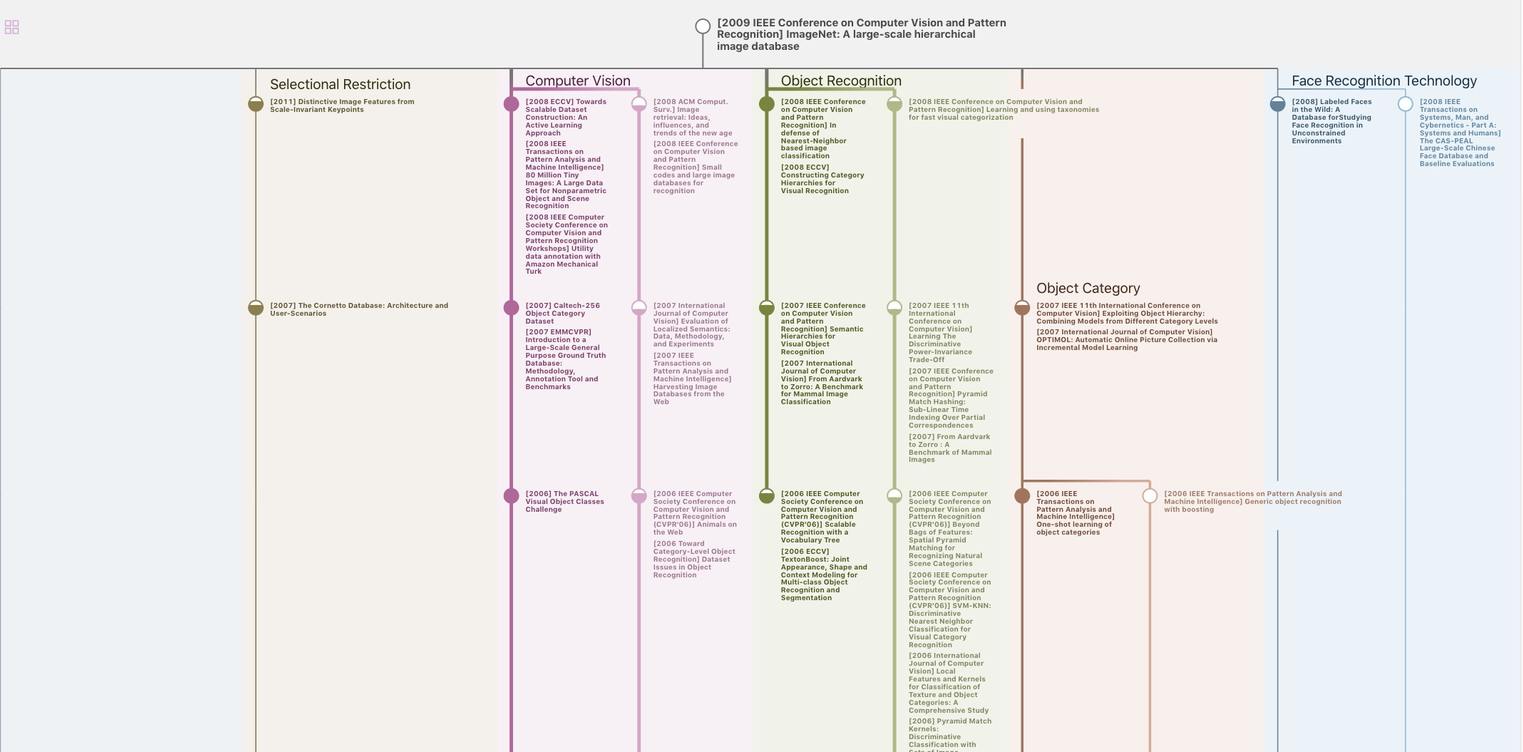
生成溯源树,研究论文发展脉络
Chat Paper
正在生成论文摘要