Combining Optimized Quantization and Machine Learning for Real-Time Data Reduction at the Edge
2022 IEEE Nuclear Science Symposium and Medical Imaging Conference (NSS/MIC)(2022)
Abstract
More and more physics and light source experiments are set to surpass TB/s data rates, which are unsustainable for data acquisition, transfer and storage systems. To keep costs, power requirements, and storage facilities feasible, data must be reduced at the source: adjacent to the detector itself. Not all data are equivalent; what is truly valuable is the information contained within. Fast Edge Machine Learning inference models on FPGAs can extract this useful information from raw data, categorize events and apply actions with low latencies. Moreover, state-of-the-art optimized nonuniform quantization can be used even closer to the source – directly at the digitizers – to maximize useful information representation. This enables the use of leaner and more robust Edge Machine Learning models. Combining optimized nonuniform quantization with fast Edge Machine Learning inference models on FPGAs reduces data generation directly at the source and thus reduces data acquisition, transfer, and storage complexity by several orders of magnitude. We demonstrate this approach by combining two prior proofs of concept targeting the CookieBox, an angular array of electron spectrometers detector developed for LCLS-II. Data is first optimally quantized before being streamed to a parallel and pipeline-ready inference model to extract the desired information. With this scheme, we measured directly on FPGA an inference latency of 5.78 µs with marginal drop in accuracy compared to simulations. If we tolerate another 3 % drop in accuracy, we reach inference latency of 1.93 µs.
MoreTranslated text
Key words
Quantization,Machine Learning,Field programmable gate arrays,Information theory,Free electron lasers,Data acquisition
AI Read Science
Must-Reading Tree
Example
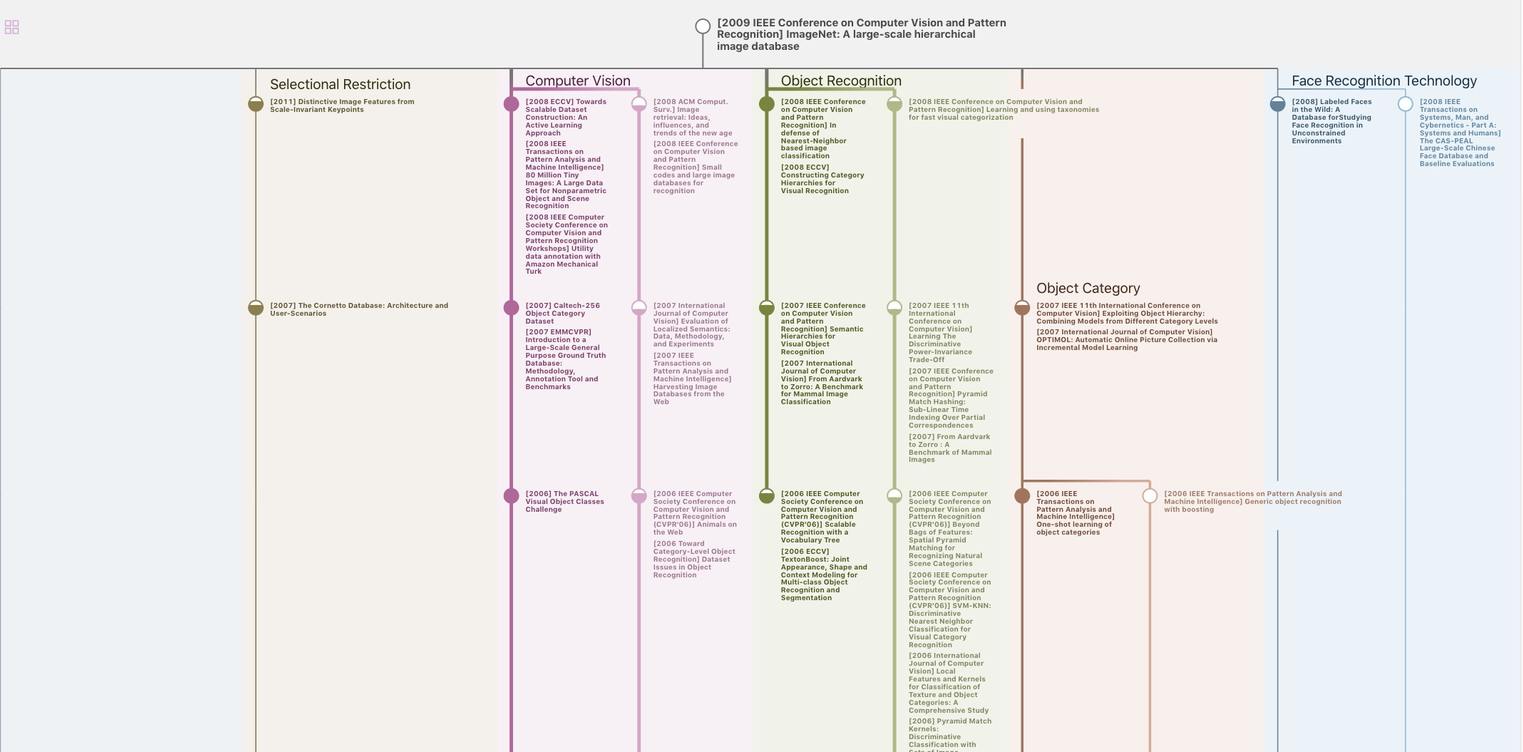
Generate MRT to find the research sequence of this paper
Chat Paper
Summary is being generated by the instructions you defined