Multi-Agent Graph-Attention Deep Reinforcement Learning for Post-Contingency Grid Emergency Voltage Control
IEEE TRANSACTIONS ON NEURAL NETWORKS AND LEARNING SYSTEMS(2024)
摘要
Grid emergency voltage control (GEVC) is paramount in electric power systems to improve voltage stability and prevent cascading outages and blackouts in case of contingencies. While most deep reinforcement learning (DRL)-based paradigms perform single agents in a static environment, real-world agents for GEVC are expected to cooperate in a dynamically shifting grid. Moreover, due to high uncertainties from combinatory natures of various contingencies and load consumption, along with the complexity of dynamic grid operation, the data efficiency and control performance of the existing DRL-based methods are challenged. To address these limitations, we propose a multi-agent graph-attention (GATT)-based DRL algorithm for GEVC in multi-area power systems. We develop graph convolutional network (GCN)-based agents for feature representation of the graph-structured voltages to improve the decision accuracy in a data-efficient manner. Furthermore, a cutting-edge attention mechanism concentrates on effective information sharing among multiple agents, synergizing different-sized subnetworks in the grid for cooperative learning. We address several key challenges in the existing DRL-based GEVC approaches, including low scalability and poor stability against high uncertainties. Test results in the IEEE benchmark system verify the advantages of the proposed method over several recent multi-agent DRL-based algorithms.
更多查看译文
关键词
Power system dynamics,Voltage control,Power system stability,Generators,Uncertainty,Load shedding,Contingency management,Attention,dynamic power system,graph convolutional network (GCN),grid emergency control,multi-agent deep reinforcement learning (DRL),voltage control
AI 理解论文
溯源树
样例
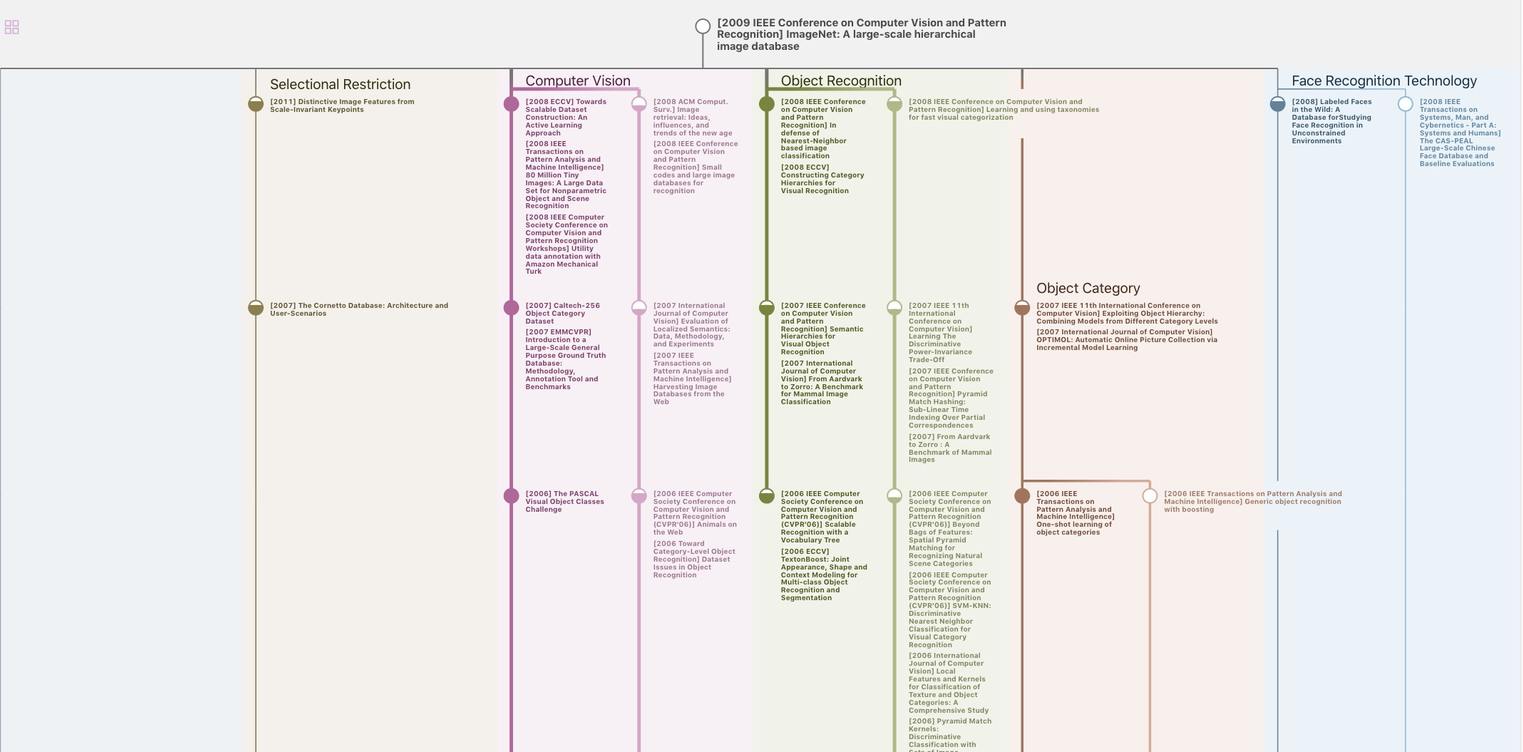
生成溯源树,研究论文发展脉络
Chat Paper
正在生成论文摘要