Deep Reinforcement Learning for Autonomous Driving with an Auxiliary Actor Discriminator
SENSORS(2024)
摘要
In the research of robot systems, path planning and obstacle avoidance are important research directions, especially in unknown dynamic environments where flexibility and rapid decision makings are required. In this paper, a state attention network (SAN) was developed to extract features to represent the interaction between an intelligent robot and its obstacles. An auxiliary actor discriminator (AAD) was developed to calculate the probability of a collision. Goal-directed and gap-based navigation strategies were proposed to guide robotic exploration. The proposed policy was trained through simulated scenarios and updated by the Soft Actor-Critic (SAC) algorithm. The robot executed the action depending on the AAD output. Heuristic knowledge (HK) was developed to prevent blind exploration of the robot. Compared to other methods, adopting our approach in robot systems can help robots converge towards an optimal action strategy. Furthermore, it enables them to explore paths in unknown environments with fewer moving steps (showing a decrease of 33.9%) and achieve higher average rewards (showning an increase of 29.15%).
更多查看译文
关键词
autonomous driving,reinforcement learning (RL),auxiliary actor discriminator (AAD),heuristic knowledge (HK)
AI 理解论文
溯源树
样例
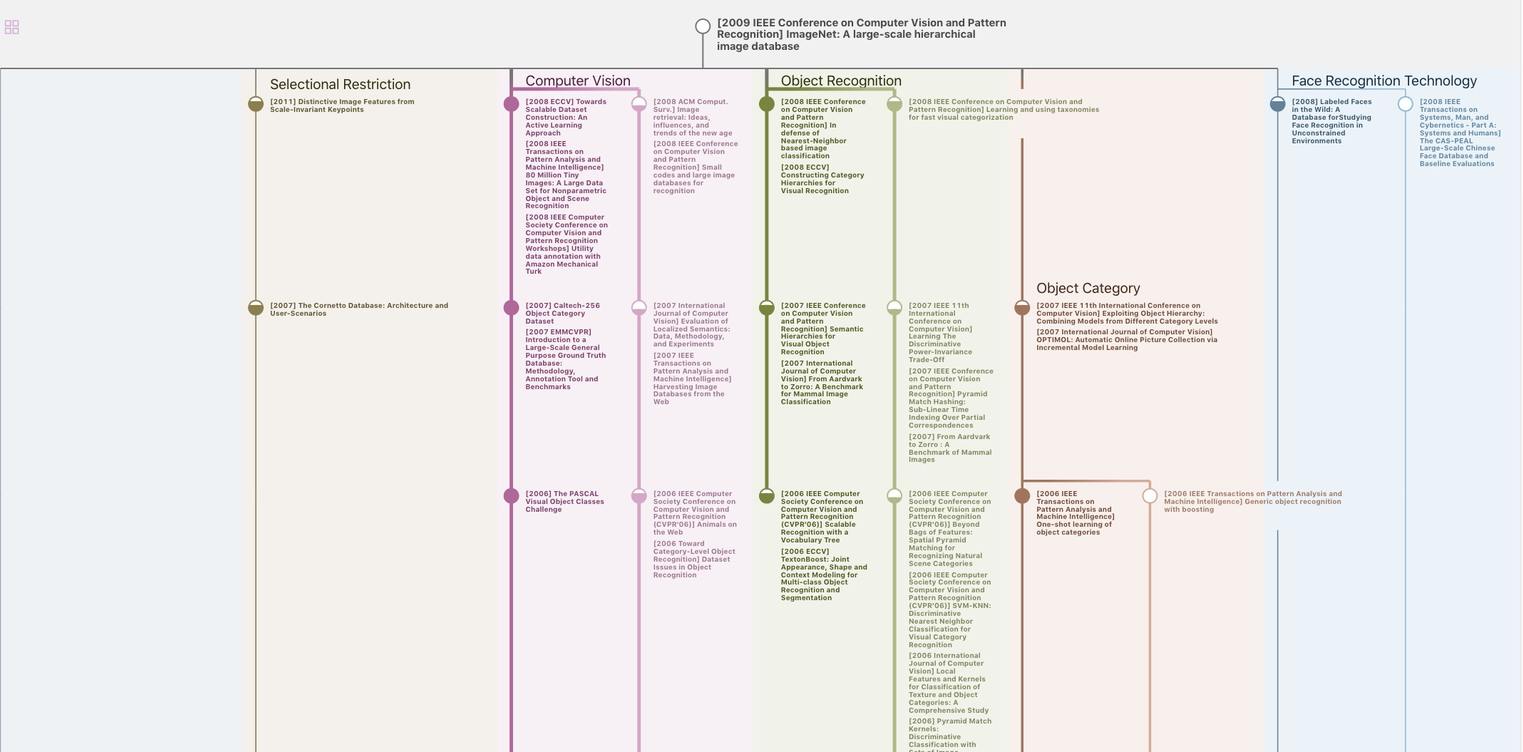
生成溯源树,研究论文发展脉络
Chat Paper
正在生成论文摘要