From Rocks to Pixels: A Protocol for Reproducible Mineral Imaging and its Applications in Machine Learning
Minerals(2023)
摘要
Identifying minerals is essential for geology, mineral exploration, engineering, and environmental sciences. Recent advances in machine learning have illustrated its potential as a fast, cost-effective, and reliable tool for identifying minerals from photographs or photomicrographs. However, in the recent literature, few studies have been dedicated to image acquisition. Machine learning generally requires reproducible, high-quality data to perform complicated tasks such as mineral identification to avoid common pitfalls. In this paper, we propose a practical image acquisition protocol for optical microscopes. This protocol focuses on ensuring reproducibility and enhancing image quality. To favor reproducibility, we detail dealing with camera errors, using reference color gauges, and establishing experimental parameters such as the external light source and temperature. For image enhancement, we explain the importance of lighting and its impact on machine learning precision, selection of the objective, and white balance calibration. In addition, we trialed the protocol on heavy mineral concentrate from till samples (20 species) with a typical deep learning model and it revealed that minor lighting modification (<5% difference in one channel) significantly increased misclassification rates: kyanite from 6.4% to 24.9% and monazite from 6.5% to 42.9%.
更多查看译文
关键词
machine learning,computer vision,image,acquisition,calibration,optical microscope
AI 理解论文
溯源树
样例
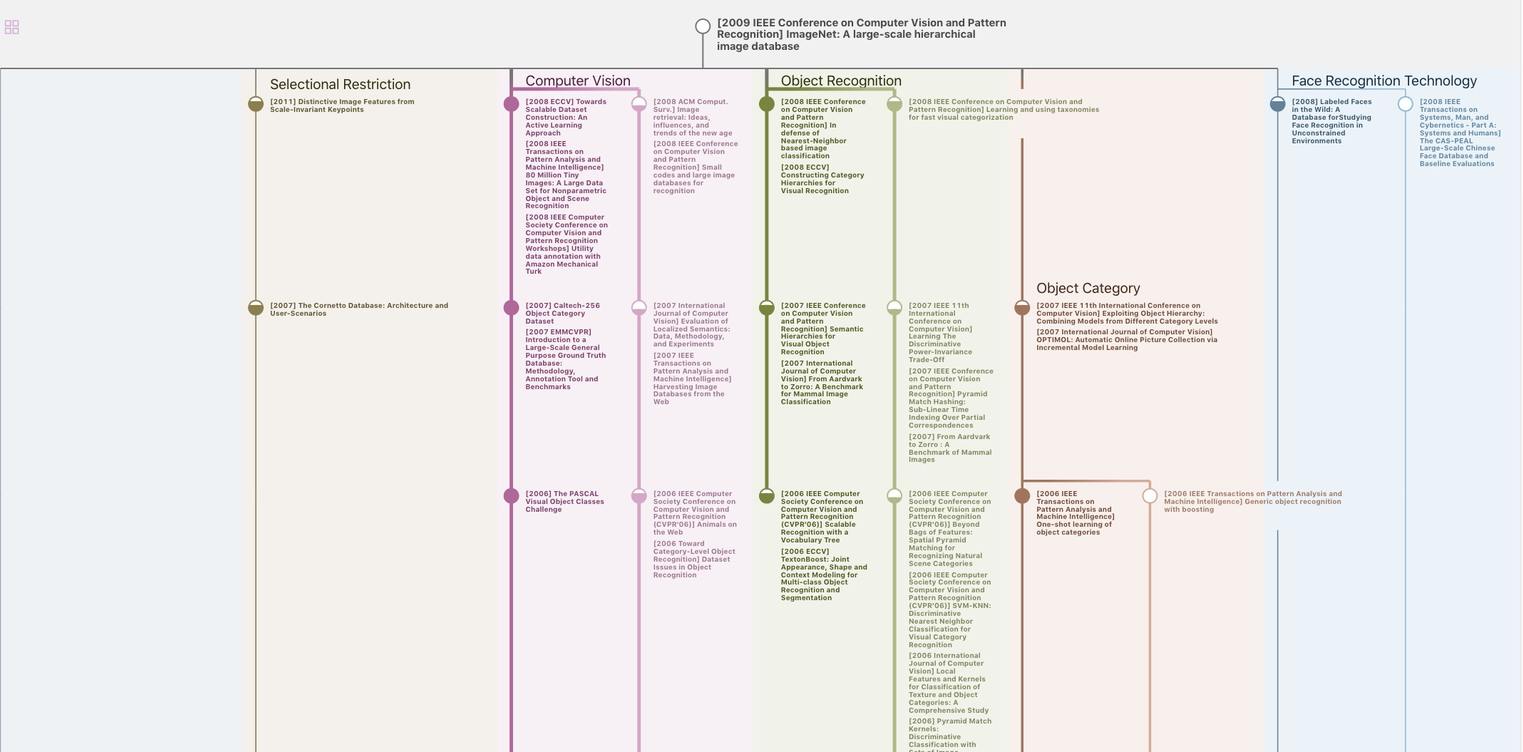
生成溯源树,研究论文发展脉络
Chat Paper
正在生成论文摘要