A Reconstructing Model Based on Time-Space-Depth Partitioning for Global Ocean Dissolved Oxygen Concentration
REMOTE SENSING(2024)
摘要
Dissolved oxygen (DO) is essential for assessing and monitoring the health of marine ecosystems. The phenomenon of ocean deoxygenation is widely recognized. Nevertheless, the limited availability of observations poses a challenge in achieving a comprehensive understanding of global ocean DO dynamics and trends. The study addresses the challenge of unevenly distributed Argo DO data by developing time-space-depth machine learning (TSD-ML), a novel machine learning-based model designed to enhance reconstruction accuracy in data-sparse regions. TSD-ML partitions Argo data into segments based on time, depth, and spatial dimensions, and conducts model training for each segment. This research contrasts the effectiveness of partitioned and non-partitioned modeling approaches using three distinct ML regression methods. The results reveal that TSD-ML significantly enhances reconstruction accuracy in areas with uneven DO data distribution, achieving a 30% reduction in root mean square error (RMSE) and a 20% decrease in mean absolute error (MAE). In addition, a comparison with WOA18 and GLODAPv2 ship survey data confirms the high accuracy of the reconstructions. Analysis of the reconstructed global ocean DO trends over the past two decades indicates an alarming expansion of anoxic zones.
更多查看译文
关键词
BGC-Argo,machine learning,ocean dissolved oxygen,spatiotemporal partition modeling,regression
AI 理解论文
溯源树
样例
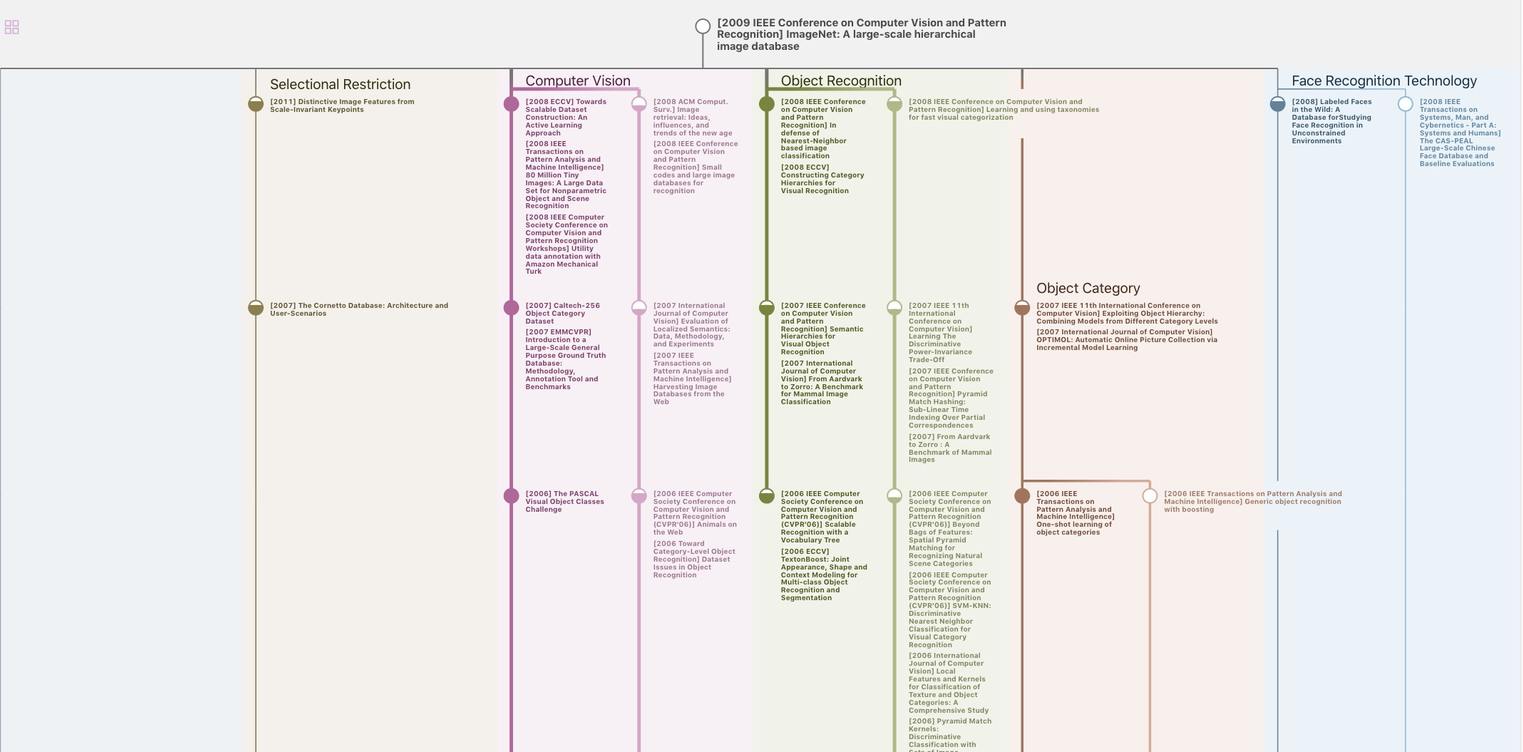
生成溯源树,研究论文发展脉络
Chat Paper
正在生成论文摘要