Beam-Space Post-Doppler Reduced-Dimension STAP Based on Sparse Bayesian Learning
REMOTE SENSING(2024)
摘要
The space-time adaptive processing (STAP) technique can effectively suppress the ground clutter faced by the airborne radar during its downward-looking operation and thus can significantly improve the detection performance of moving targets. However, the optimal STAP requires a large number of independent identically distributed (i.i.d) samples to accurately estimate the clutter plus noise covariance matrix (CNCM), which limits its application in practice. In this paper, we fully consider the heterogeneity of clutter in real-world environments and propose a sparse Bayesian learning-based reduced-dimension STAP method that achieves suboptimal clutter suppression performance using only a single sample. First, the sparse Bayesian learning (SBL) algorithm is used to estimate the CNCM using a single training sample. Second, a novel angular Doppler channel selection algorithm is proposed with the criterion of maximizing the output signal-to-clutter-noise ratio (SCNR). Finally, the reduced-dimension STAP filter is constructed using the selected channels. Simulation results show that the proposed algorithm can achieve suboptimal clutter suppression performance in extremely heterogeneous clutter environments where only one training sample can be used.
更多查看译文
关键词
space-time adaptive processing,sparse Bayesian learning,reduced dimension,angular Doppler channel
AI 理解论文
溯源树
样例
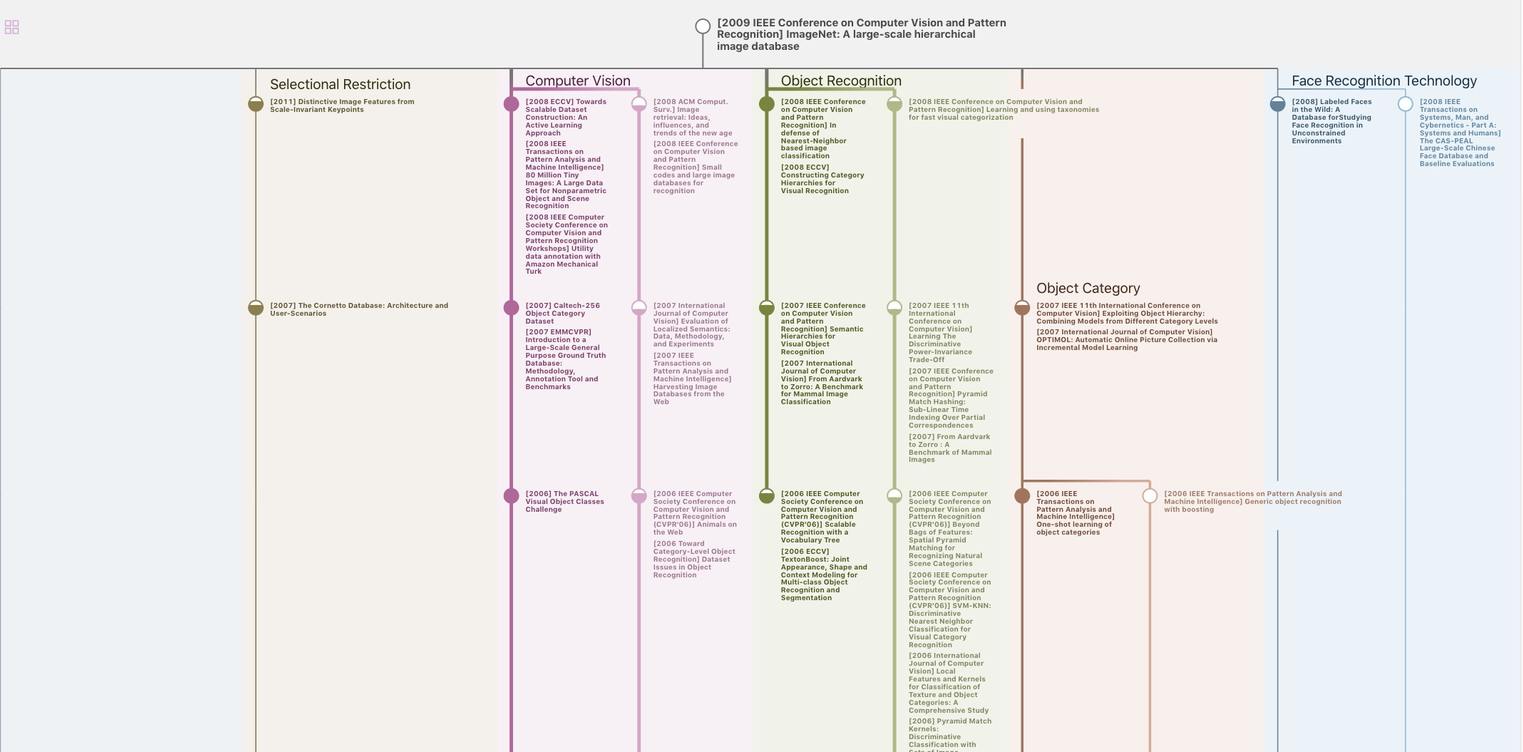
生成溯源树,研究论文发展脉络
Chat Paper
正在生成论文摘要