Cascaded Fuzzy Reward Mechanisms in Deep Reinforcement Learning for Comprehensive Path Planning in Textile Robotic Systems
APPLIED SCIENCES-BASEL(2024)
Abstract
With the rapid advancement of industrial automation and artificial intelligence technologies, particularly in the textile industry, robotic technology is increasingly challenged with intelligent path planning and executing high-precision tasks. This study focuses on the automatic path planning and yarn-spool-assembly tasks of textile robotic arms, proposing an end-to-end planning and control model that integrates deep reinforcement learning. The innovation of this paper lies in the introduction of a cascaded fuzzy reward system, which is integrated into the end-to-end model to enhance learning efficiency and reduce ineffective exploration, thereby accelerating the convergence of the model. A series of experiments conducted in a simulated environment demonstrate the model's exceptional performance in yarn-spool-assembly tasks. Compared to traditional reinforcement learning methods, our model shows potential advantages in improving task success rates and reducing collision rates. The cascaded fuzzy reward system, a core component of our end-to-end deep reinforcement learning model, offers a novel and more robust solution for the automated path planning of robotic arms. In summary, the method proposed in this study provides a new perspective and potential applications for industrial automation, especially in the operation of robotic arms in complex and uncertain environments.
MoreTranslated text
Key words
fuzzy reward,end-to-end network,trajectory planning,forward kinematics,deep reinforcement learning
AI Read Science
Must-Reading Tree
Example
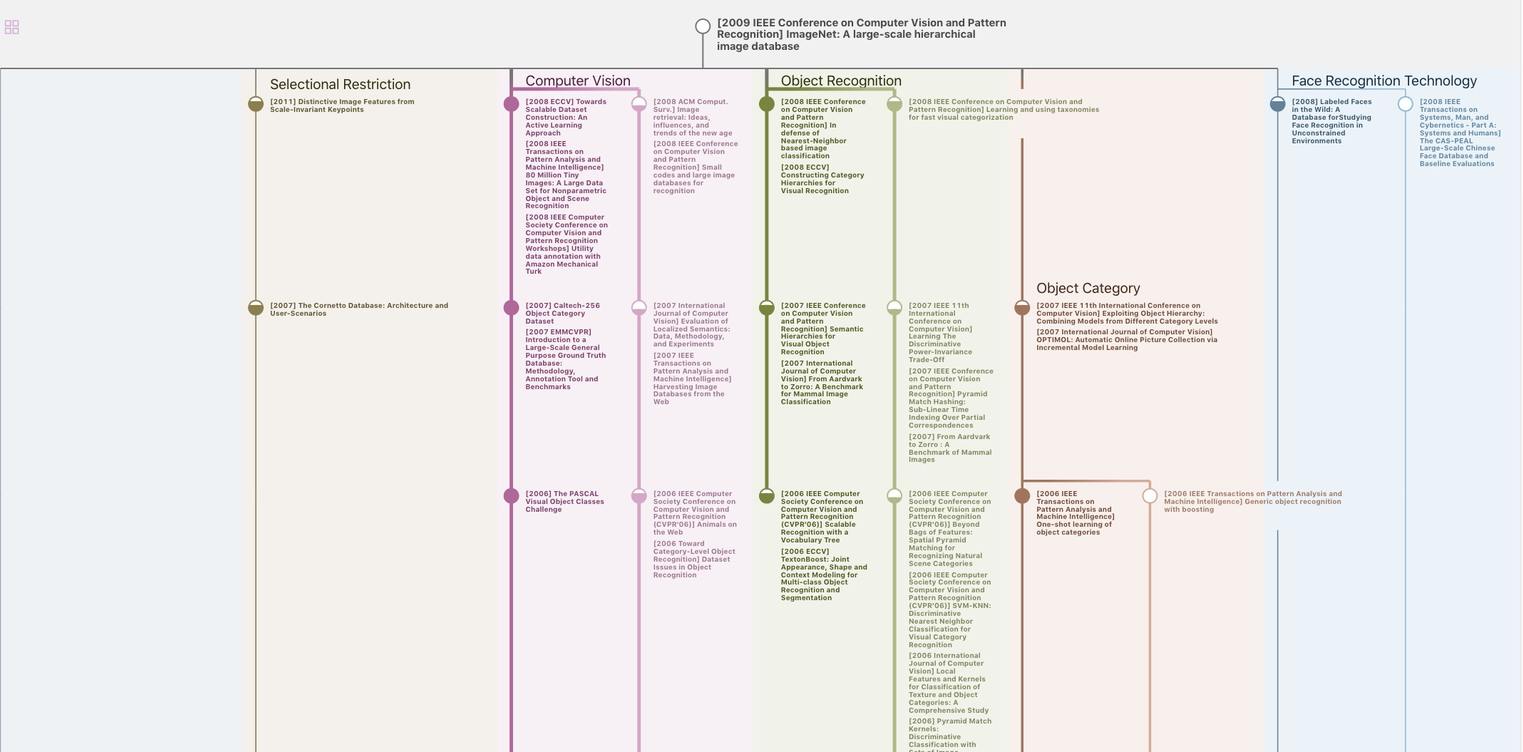
Generate MRT to find the research sequence of this paper
Chat Paper
Summary is being generated by the instructions you defined