Box-Cox transformation based state-space modeling as a unified prognostic framework for degradation linearization and RUL prediction enhancement
RELIABILITY ENGINEERING & SYSTEM SAFETY(2024)
摘要
Remaining useful life (RUL) prediction is a core of prognostics and health management (PHM), which is beneficial to arranging preventive maintenance and avoiding sudden equipment breakdown. Accurate modeling of various degradation data and appropriate data preprocessing are important prerequisites for precise RUL prediction. Practically, nonlinear degradation data will increase the difficulty of degradation modeling, thereby affecting RUL prediction accuracy. In currently existing research, data linearization using Box -Cox transformation (BCT) is often treated as a separate data preprocessing step and its integration with prognostic modeling is seldom considered and reported. This paper considers the integration of BCT with state -space modeling as a unified prognostic framework to effectively linearize degradation data and thus simplify degradation modeling and enhance RUL prediction accuracy. The proposed unified prognostic framework is validated by using both simulated data and experimental gearbox degradation data. Experimental results demonstrate that the proposed unified prognostic framework exhibits excellent performance in degradation data linearization and RUL prediction enhancement. Moreover, comparisons with a recently advanced prognostic method based on BCT and Bayesian model parameters updating demonstrate the superiority of the integration of BCT with state -space modeling for achieving higher RUL prediction accuracy and less prediction errors.
更多查看译文
关键词
Box-cox transformation,State-space modeling,Linearization,Degradation modeling,Prognostics,Remaining useful life
AI 理解论文
溯源树
样例
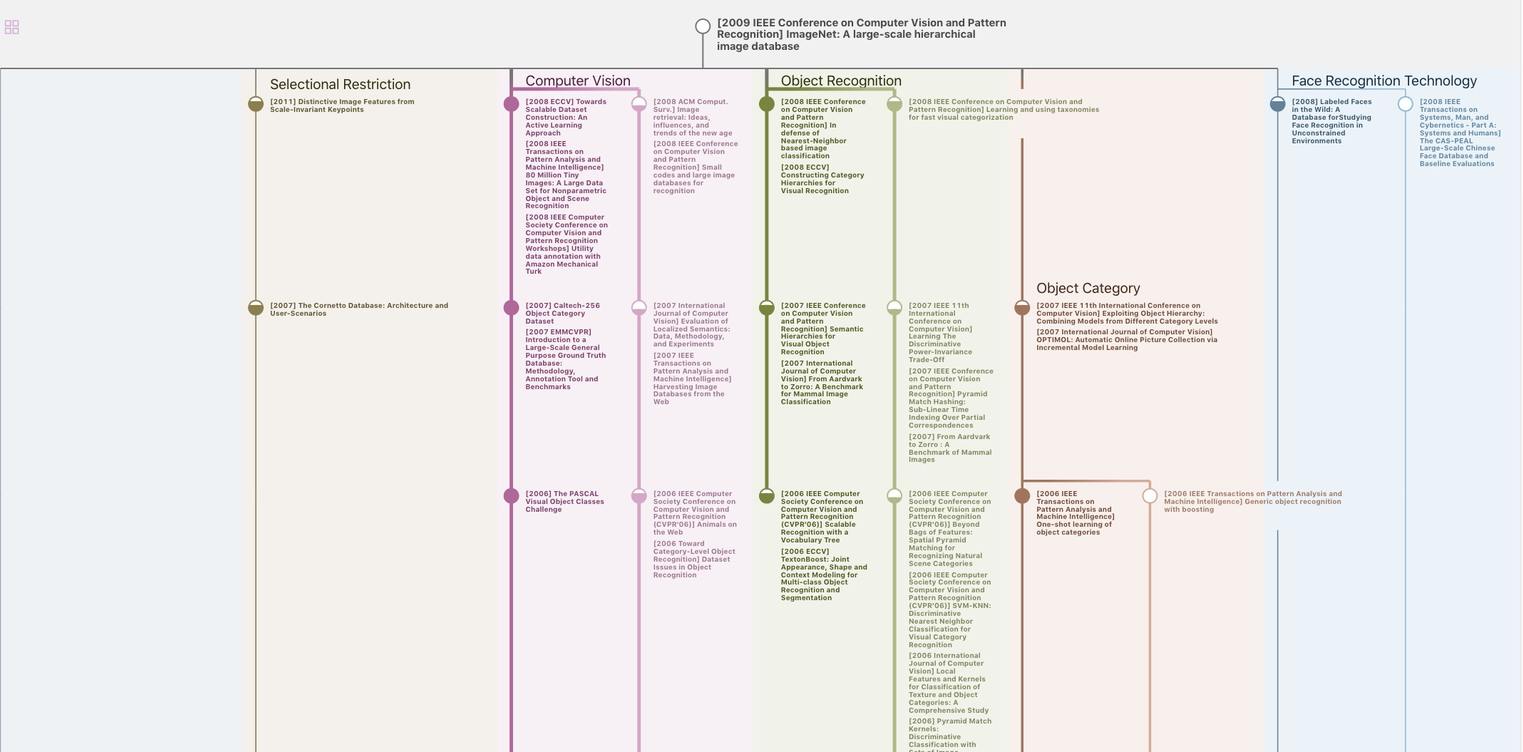
生成溯源树,研究论文发展脉络
Chat Paper
正在生成论文摘要