Machine learning evidence map reveals global differences in adaptation action
ONE EARTH(2024)
摘要
Climate change adaptation policies are urgently needed, but the large volume and variety of evidence limits the ability of practitioners to make informed decisions. Here, we create an evidence map of adaptation policy research, selecting and categorizing 8,691 documents using state-of-the-art transformers -based machine learning models. We combine policy -relevant categories, such as the NATO -typology and governance levels, with automatically extracted locations and a structural topic model to provide a detailed global assessment of the tools governments are using to address climate change risks and impacts. We find that internationallevel policies, as well as policies in North America and much of the Global South, emphasize financial instruments, whereas national policies, particularly in Europe and Oceania, favor authority -based legislation. Collaborative approaches are most common at the local level. Despite a rapidly expanding evidence base overall, we note persistent geographic inequalities and limited evidence on information -based policies, policy implementation, and structural reforms.
更多查看译文
关键词
climate change,adaptation policy,natural language processing (NLP),ClimateBERT,supervised machine learning,topic modeling,unsupervised machine learning,big data,evidence synthesis,Paris Agreement
AI 理解论文
溯源树
样例
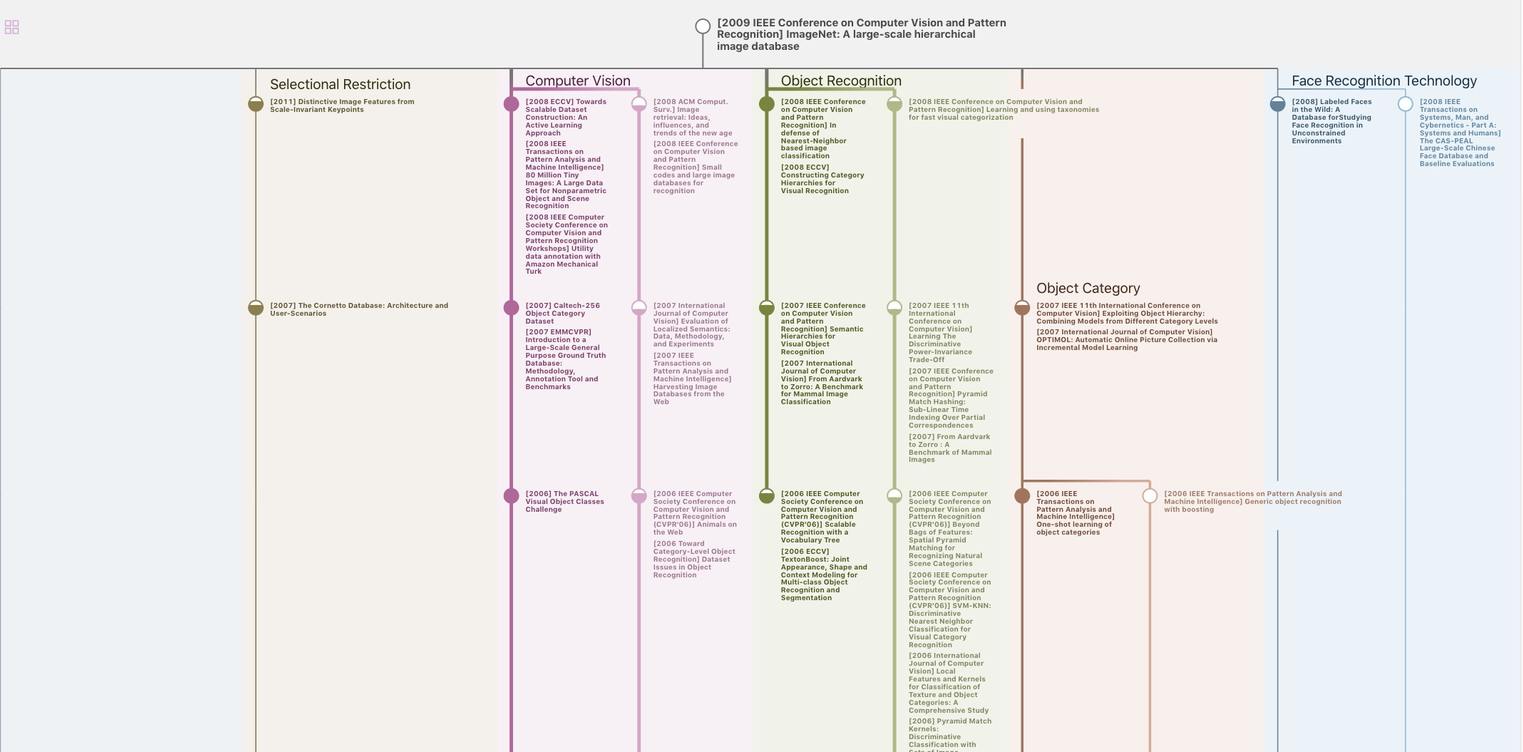
生成溯源树,研究论文发展脉络
Chat Paper
正在生成论文摘要