Principles and applications of convolutional neural network for spectral analysis in food quality evaluation: A review
Journal of Food Composition and Analysis(2024)
Abstract
The spectroscopic technologies have been successfully applied to food quality evaluation owing to their abilities of wavelengths being sensitive to biological components of food, and they also have advantages of non-destructive, rapid, convenient. For the food quality evaluation, it’s a critical step to build mapping relationships between the spectral data and the specific food quality targets. Convolutional neural network (CNN), as a data-driven deep learning method, provides an end-to-end modeling approach to build mapping without spectral preprocessing techniques, which reduce the need from prior knowledge and human efforts. Meanwhile, in terms of model accuracy, robustness and generalization, CNN also have exhibited superior performances in the recent studies. In this review, we provide a brief overview of the CNN technique firstly, and then systematically analyze existing studies on CNN techniques for spectral analysis into three categories, including end-to-end modeling, spectral dimension reduction and interoperability. Meanwhile, we review the latest applications of CNN techniques for both qualitative and quantitative evaluation of food quality. Then, based on the large number of CNN models in the existing studies, we provide some guidelines for new users to develop a CNN model in their data analysis process, including the design of network structure, tuning the hyperparameters and training strategies. Finally, the advantages, limitations and future perspectives for food quality evaluation by spectral analysis and CNN approaches are also discussed in the study.
MoreTranslated text
Key words
Convolutional neural network,Spectroscopic technologies,Evaluation,Food quality
AI Read Science
Must-Reading Tree
Example
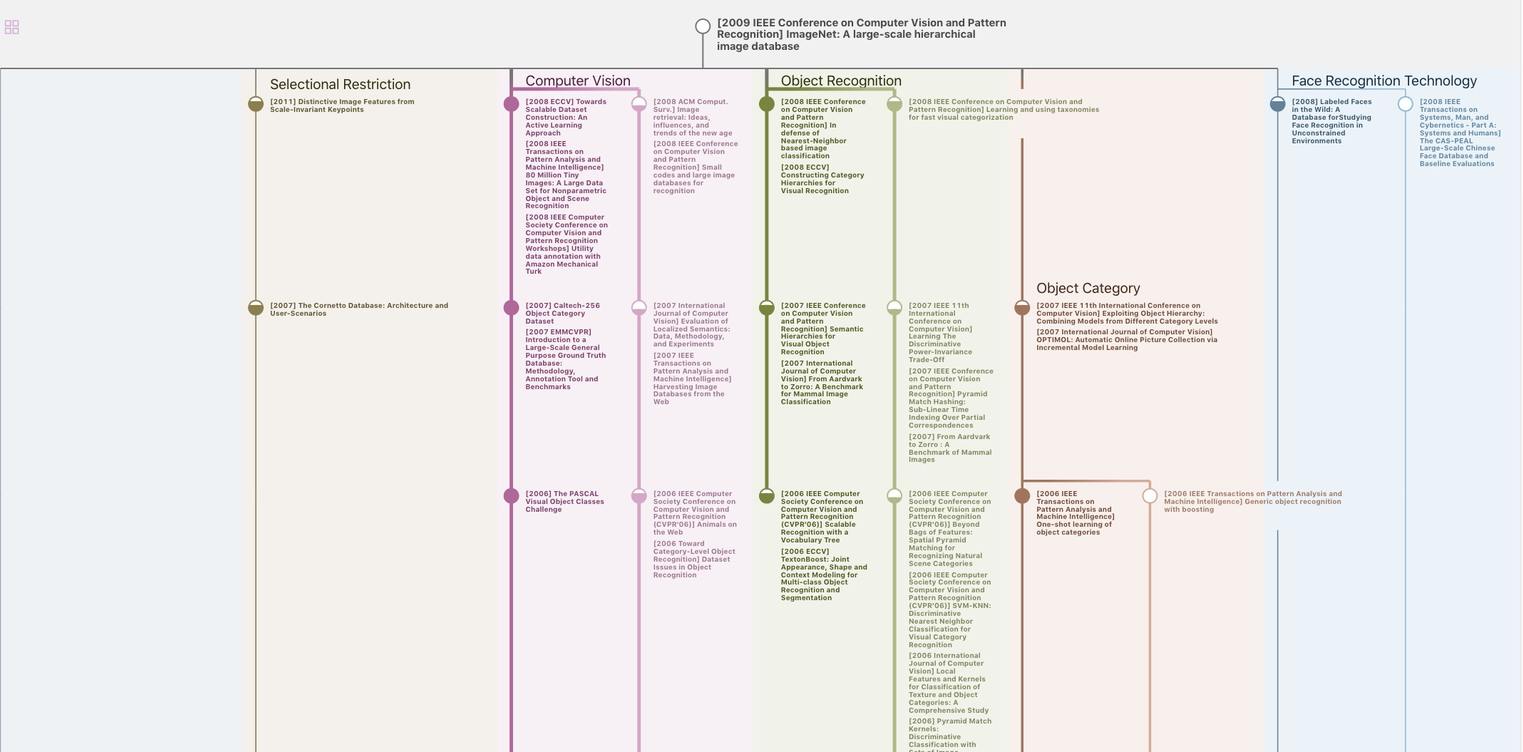
Generate MRT to find the research sequence of this paper
Chat Paper
Summary is being generated by the instructions you defined