Light Gradient Boosting Machine (LightGBM) to forecasting data and assisting the defrosting strategy design of refrigerators
INTERNATIONAL JOURNAL OF REFRIGERATION(2024)
摘要
This study proposes using the Light Gradient Boosting Machine (LightGBM) to improve the defrosting control strategy in frost-free refrigerators. By analyzing data and optimizing control parameters, the aim is to enhance defrosting performance and reduce energy consumption using the time-temperature difference (t-dT) approach. The research involves analyzing performance and reliability data for two control strategies (time-temperature (t-T) and t-dT), creating three feature sets (FS1, FS2, and FS3) based on correlation analysis, and employing LightGBM models to forecast datasets. The control parameter threshold (Delta T-op,T-s) is optimized using the LightGBM-based data. The key findings indicate that the t-dT strategy with a fixed threshold (7.8 degrees C) outperforms the t-T method in efficiency at an ambient temperature of 38 degrees C. At 10 degrees C, the performance tests show no significant difference, but the t-T method performs better in reliability tests. The FS1-based data from the t-dT strategy in the reliability test at 10 degrees C are considered ideal input, and the LightGBM models generate FS2-based and FS3-based data for evaluation. The optimized t-dT defrosting strategy achieves favorable refrigeration conditions with minimal power consumption and optimal cooling capacity. The ideal Delta T-op,T-s threshold, based on data for the idealized frosting condition, is determined to be 8.3 degrees C.
更多查看译文
关键词
Defrosting control strategy,LightGBM,Feature sets,Correlation analysis
AI 理解论文
溯源树
样例
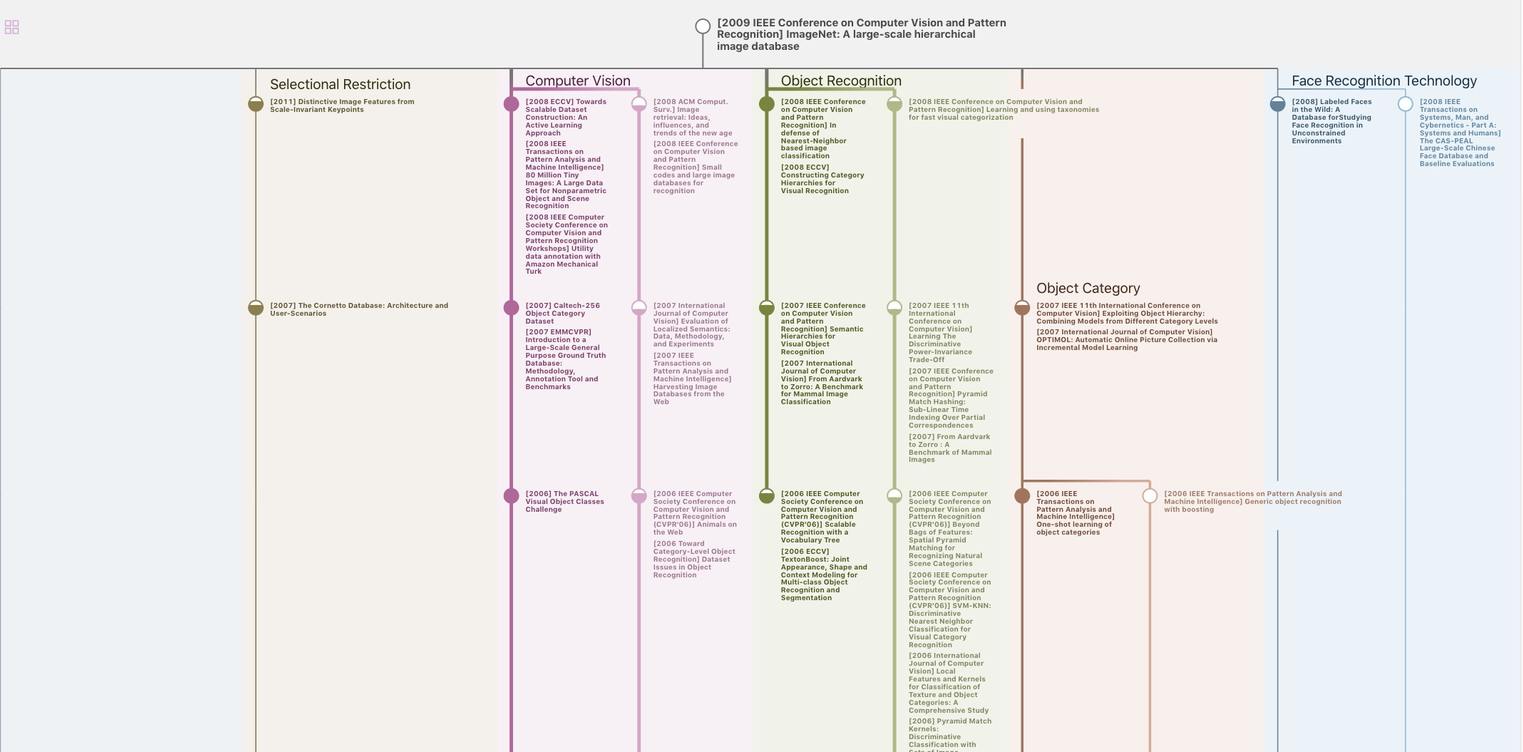
生成溯源树,研究论文发展脉络
Chat Paper
正在生成论文摘要