Analytically tractable heteroscedastic uncertainty quantification in Bayesian neural networks for regression tasks
NEUROCOMPUTING(2024)
Abstract
The tractable approximate Gaussian inference (TAGI) method allows for analytical parameter inference in Bayesian neural networks. In its current form, TAGI can only model homoscedastic aleatory uncertainty that is quantified by a constant error variance across the input covariate-domain. In this paper, we present the approximate Gaussian variance inference (AGVI) method that enables analytical inference of the error variance term as a Gaussian random variable. The combined framework regrouping TAGI and AGVI, referred to as TAGIV, enables modeling heteroscedastic aleatory uncertainty in Bayesian neural networks. TAGI-V outperforms the homoscedastic version of TAGI in terms of predictive performance for the benchmark regression datasets. In comparison with other approximate inference methods, TAGI-V is an order of magnitude faster and exhibits a comparable or superior predictive performance.
MoreTranslated text
Key words
Tractable approximate Gaussian inference,Approximate Gaussian variance inference,Aleatory uncertainty,Closed-form inference,Gaussian multiplicative approximation
AI Read Science
Must-Reading Tree
Example
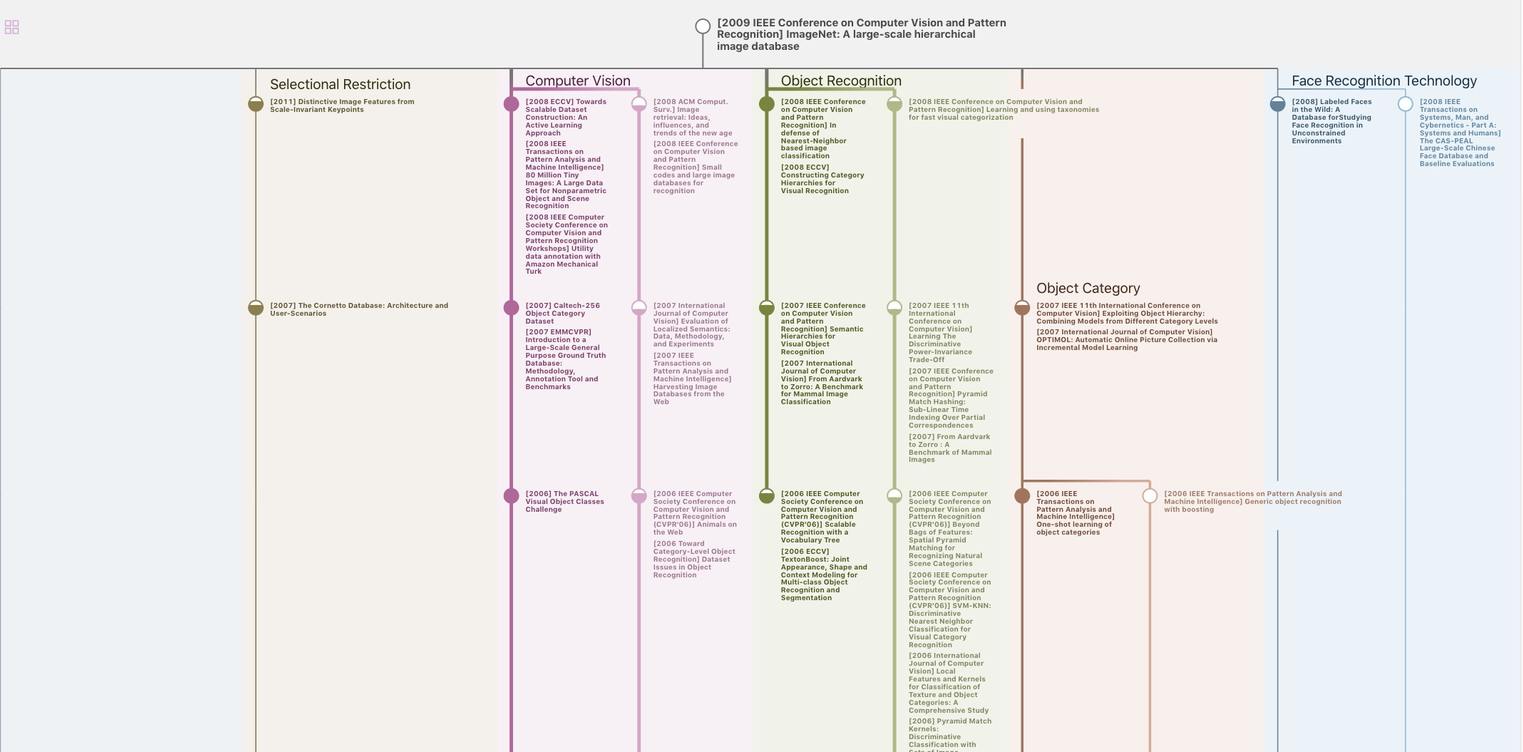
Generate MRT to find the research sequence of this paper
Chat Paper
Summary is being generated by the instructions you defined