Ridesplitting demand prediction via spatiotemporal multi-graph convolutional network
EXPERT SYSTEMS WITH APPLICATIONS(2024)
摘要
Ridesplitting demand prediction plays an important role in vehicle scheduling and intelligent transportation system construction. Accurate ridesplitting demand prediction is crucial for alleviating supply-demand imbalance and increasing vehicle utilization. Ridesplitting refers to the sharing of a vehicle by multiple passengers with similar routes, thereby achieving a reduction in the adverse effects of increased congestion caused by ridehailing. However, most existing works mainly address the region-based ride-hailing demand prediction, whereas few works focus on the ridesplitting demand prediction, which takes into account the correlation between shared orders. To address the issue, we develop several intersection-based graphs to character the ridesplitting demand correlations between the intersections. The multi-graph convolutional networks (MGCN) with different graph combinations are constructed to capture the spatial topologies. Furthermore, we introduce the probabilistic model structure to predict the uncertainty of ridesplitting demand. A novel deep learning model, referred to as the Multi-graph Convolutional Gated Recurrent Unit with Probabilistic prediction (MGCGRU-P) is proposed. We conduct extensive numerical experiments on the real-world ride-hailing demand dataset (from Beijing, China). The results demonstrate that the model we propose performs the best against baseline models.
更多查看译文
关键词
Ridesplitting,Demand prediction,Multi -graph convolutional network,Probabilistic prediction
AI 理解论文
溯源树
样例
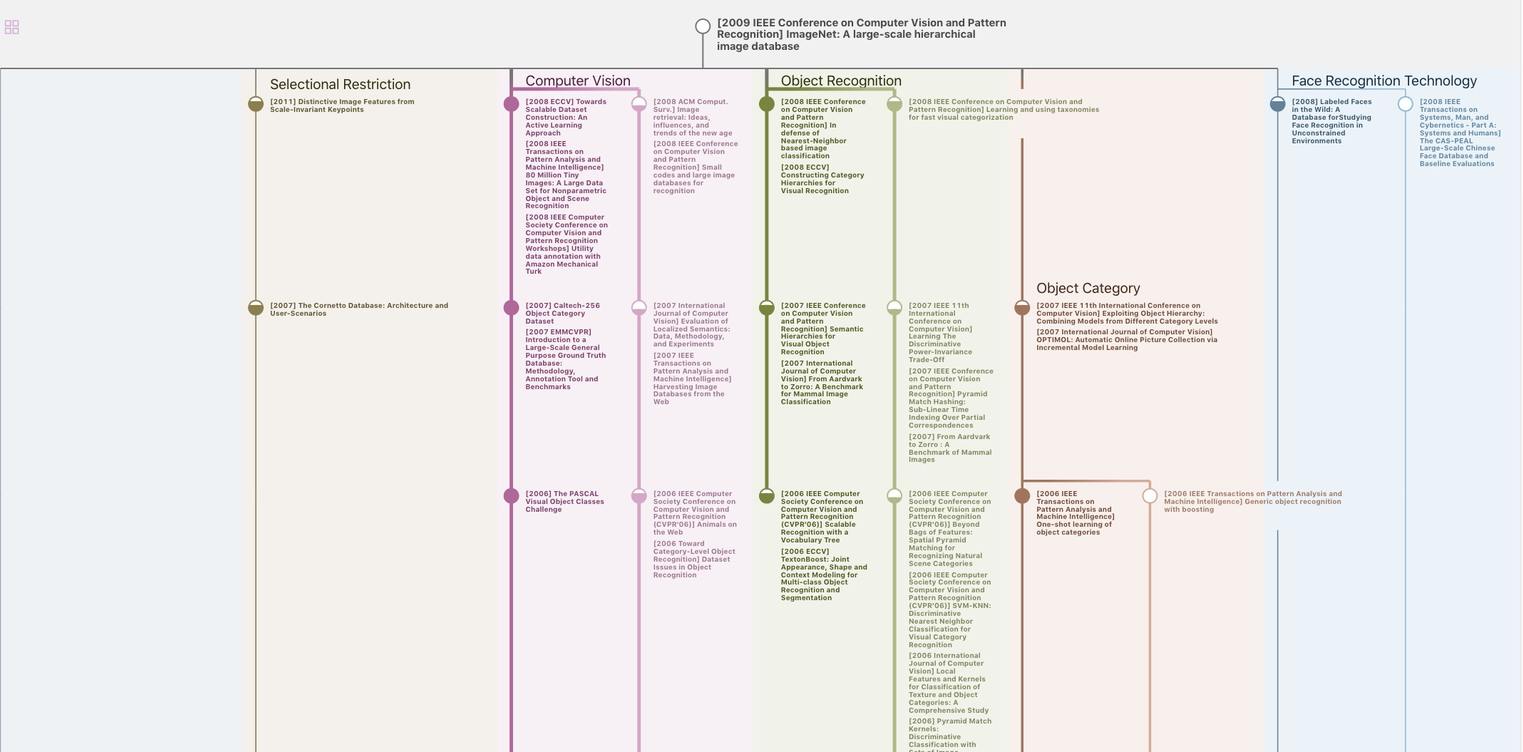
生成溯源树,研究论文发展脉络
Chat Paper
正在生成论文摘要