Relation-CNN: Enhancing website fingerprinting attack with relation features and NFS-CNN
EXPERT SYSTEMS WITH APPLICATIONS(2024)
摘要
Website fingerprinting (WF) attacks enable a local eavesdropper to infer the visiting websites of a user even in an encrypted connection. To improve the prediction performance of WF attacks, we approach from two perspectives, i.e., features and models. In terms of features, the paper proposes relation features, which contain useful classifying information that previous features do not reveal. Besides, we propose a CNN -based framework called NFS-CNN, whose key innovations include two aspects. First, we make three optimizations for ResNet18 to reduce the loss of data information. Second, we design a feature -attention block to automatically learn the contribution that the data of different channels makes to the prediction performance. Based on relation features and NFS-CNN, we put forward Relation -CNN, further design comprehensive evaluations to test relation features and Relation -CNN on typical Tor datasets, including webpage access and onion service access. Experimental results show that relation features do reveal a lot of unique discriminative information, which is hardly learned by DNNs. Further, we explain the results by theoretical analysis, i.e., information leakage analysis. Evaluation results also indicate that the accuracy of WF attacks is always improved when adding the relation features into the input. On top of that, Relation -CNN consistently performs the best on all evaluations, including the scenarios of closed -world and open -world, defended and non -defended. Remarkably, Relation -CNN can outperform the best baseline attack by about 5% and 8% on defended and non -defended datasets, respectively.
更多查看译文
关键词
Deep learning,Multimodal learning,Traffic analysis,Website fingerprinting,Relation features
AI 理解论文
溯源树
样例
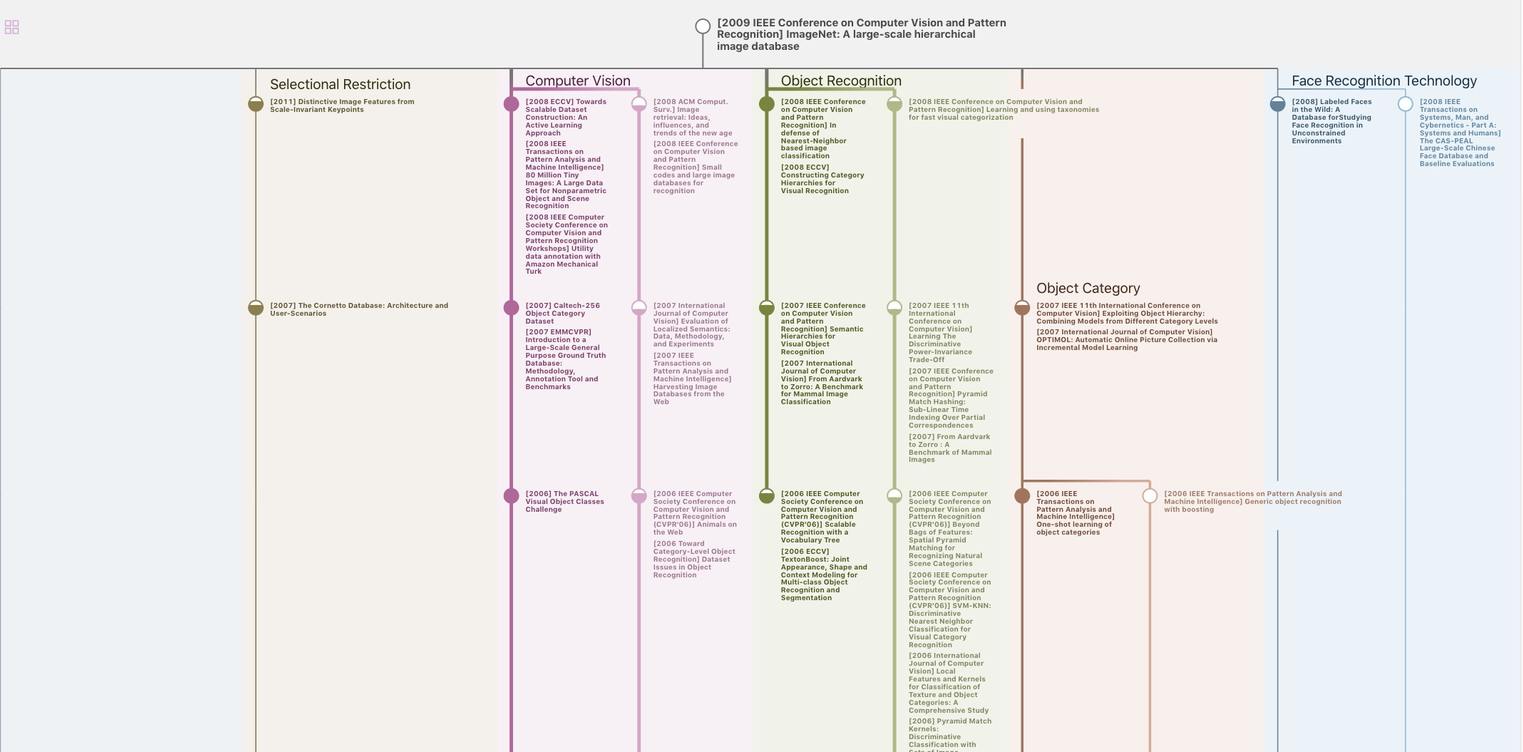
生成溯源树,研究论文发展脉络
Chat Paper
正在生成论文摘要