MHA-Net: Multi-source heterogeneous aerodynamic data fusion neural network embedding reduced-dimension features
AEROSPACE SCIENCE AND TECHNOLOGY(2024)
摘要
Developing a high -fidelity, cost-effective aerodynamic database is crucial for addressing the balance of accuracy and cost in aircraft design. Recently, data fusion technology has achieved breakthroughs in aerodynamics, offering new insights for enhancing efficiency and reducing costs in aerodynamic acquisition. Nevertheless, most techniques focus on homogeneous data, neglecting the utilization of heterogeneous aerodynamic data that includes crucial physical information. In this research, a novel heterogeneous aerodynamic data fusion method embedding reduced-dimension features (MHA-Net) is proposed. When constructing the state-to-aerodynamic model, the MHA-Net efficiently incorporates neglected distributed load and embeds reduced-dimension features to form a novel physical-embedded neural network. This method significantly enhances model accuracy and robustness in few-shot learning. A comparative analysis and verification are conducted on the variable state experimental case of a wind turbine airfoil and the variable shape simulation of RAE2822. The results demonstrate that this method significantly decreases both the average error and dispersion of aerodynamic models. In the few-shot learning region, an average error reduction can reach over 20%, accompanied by a dispersion decrease exceeding 50% on average.
更多查看译文
关键词
Multi-source modeling,Heterogeneous model,Aerodynamic data fusion,Reduced-dimension extraction,Feature embedding
AI 理解论文
溯源树
样例
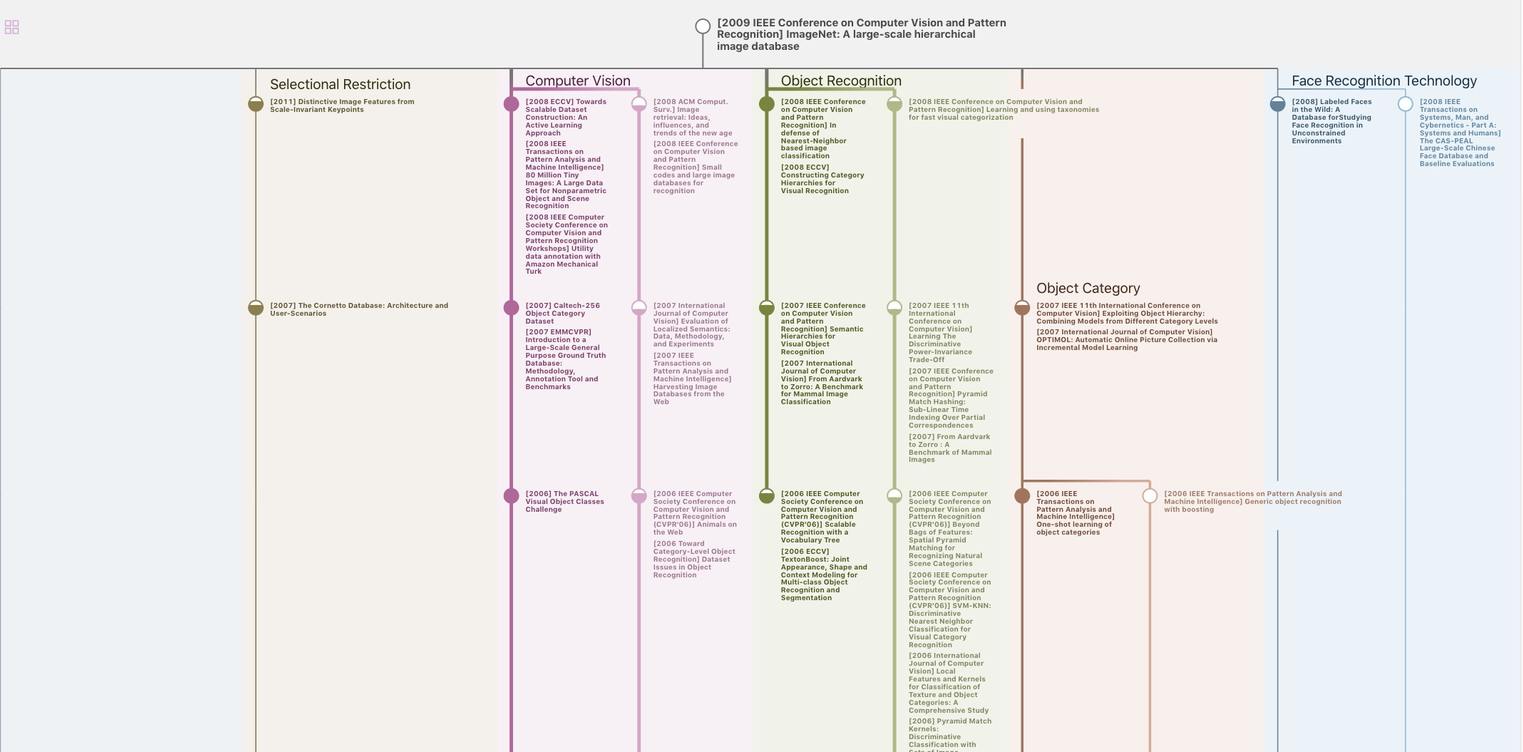
生成溯源树,研究论文发展脉络
Chat Paper
正在生成论文摘要