A new convex model for linear hyperspectral unmixing
JOURNAL OF COMPUTATIONAL AND APPLIED MATHEMATICS(2024)
摘要
Over the past two decades, Minimum Simplex Volume-based (MV) methods for linear hyper spectral unmixing have attracted considerable academic attention due to their robustness in the absence of pure pixels. Within this framework, certain prevalent MV models incorporate a Log-Absolute-Determinant Function (LADF) that operates on a matrix variable Q. In practical applications, the LADF inherently exhibits non-convex characteristics, thus limiting its applicability in real-world scenarios. To address this challenge, this paper employs Tikhonov regularization, combined with a series of equivalent transformations, to construct a positively definite matrix, thereby rendering the corresponding LADF convex. Theoretical foundations linking the newly convexified LADF and the original non-convex function are rigorously established. Experimental validation, conducted on standard hyperspectral benchmark datasets, confirms its efficiency and necessity. Notably, this paper introduces a unified strategy for ensuring convexity in MV models for linear hyperspectral unmixing.
更多查看译文
关键词
Linear hyperspectral unmixing (LHU),Matrix function optimization,Strongly convex,Adaptive moment method
AI 理解论文
溯源树
样例
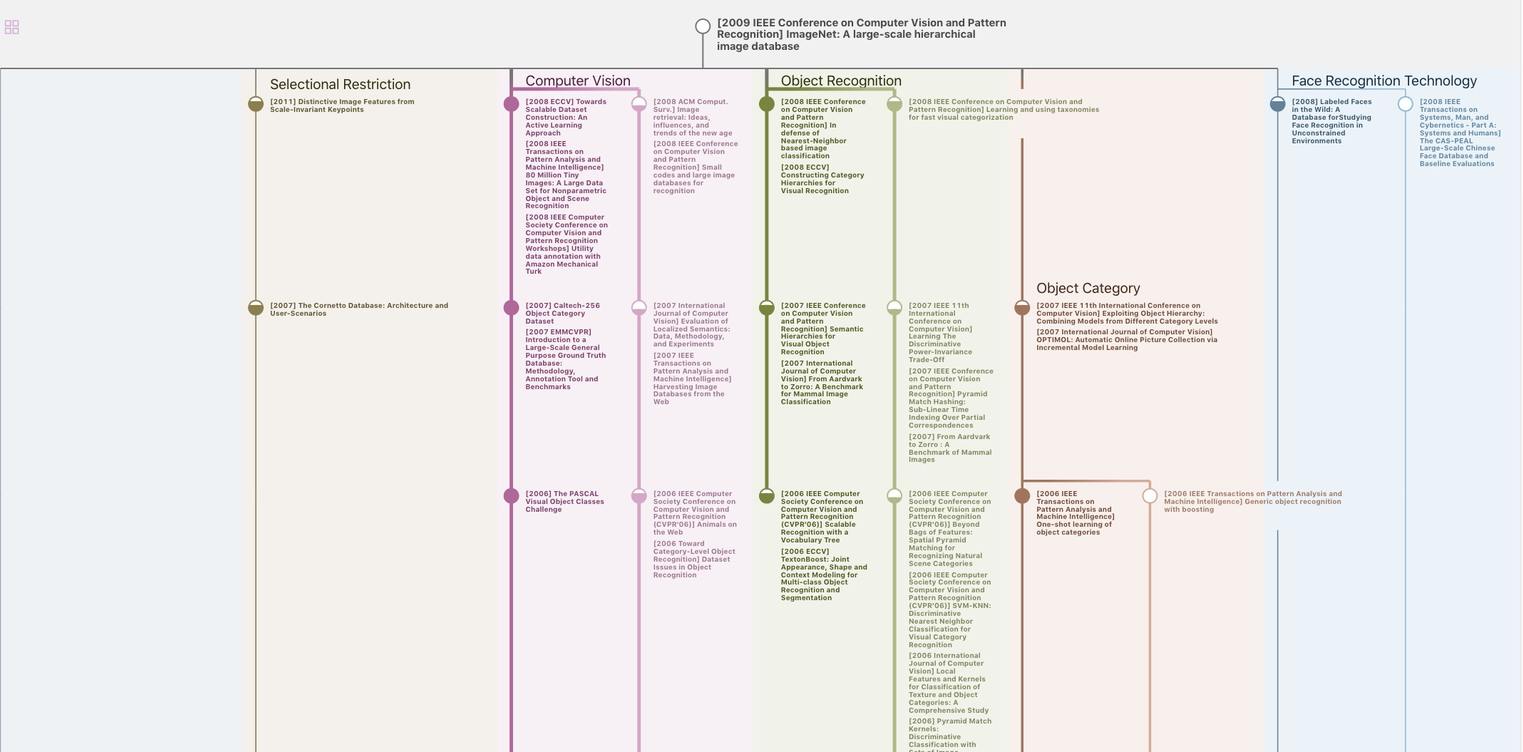
生成溯源树,研究论文发展脉络
Chat Paper
正在生成论文摘要