Sag-flownet: self-attention generative network for airfoil flow field prediction
Soft Computing(2024)
Abstract
Flow field prediction is essential for airfoil design. It is a time-consuming task to obtain the flow fields around an airfoil. Convolution neural networks (CNN) have been applied for flow field prediction in recent years. However, CNN-based methods rely heavily on convolutional kernels to process information within local neighborhoods, making it difficult to capture global information. In this paper, we propose a novel self-attention generative network referred to as SAG-FlowNet, both for original and optimization airfoil flow field prediction. We investigate the self-attention mechanism with a multi-layer convolutional generative network. We use the self-attention module to capture various information within and between flow fields, and with the help of the attention module, the CNN can utilize the information with stronger relationships regardless of their distances to achieve better flow field prediction results. Through extensive experiments, we explore the proposed SAG-FlowNet performance. The experimental results show that the method has accurate and universal performance for the reconstruction and prediction of the flow field both for original and optimized airfoils. SAG-FlowNet is promising for fast flow field prediction and has potential applications in accelerating airfoil design.
MoreTranslated text
Key words
Flow field,Generative networks,Self-attention,Prediction
AI Read Science
Must-Reading Tree
Example
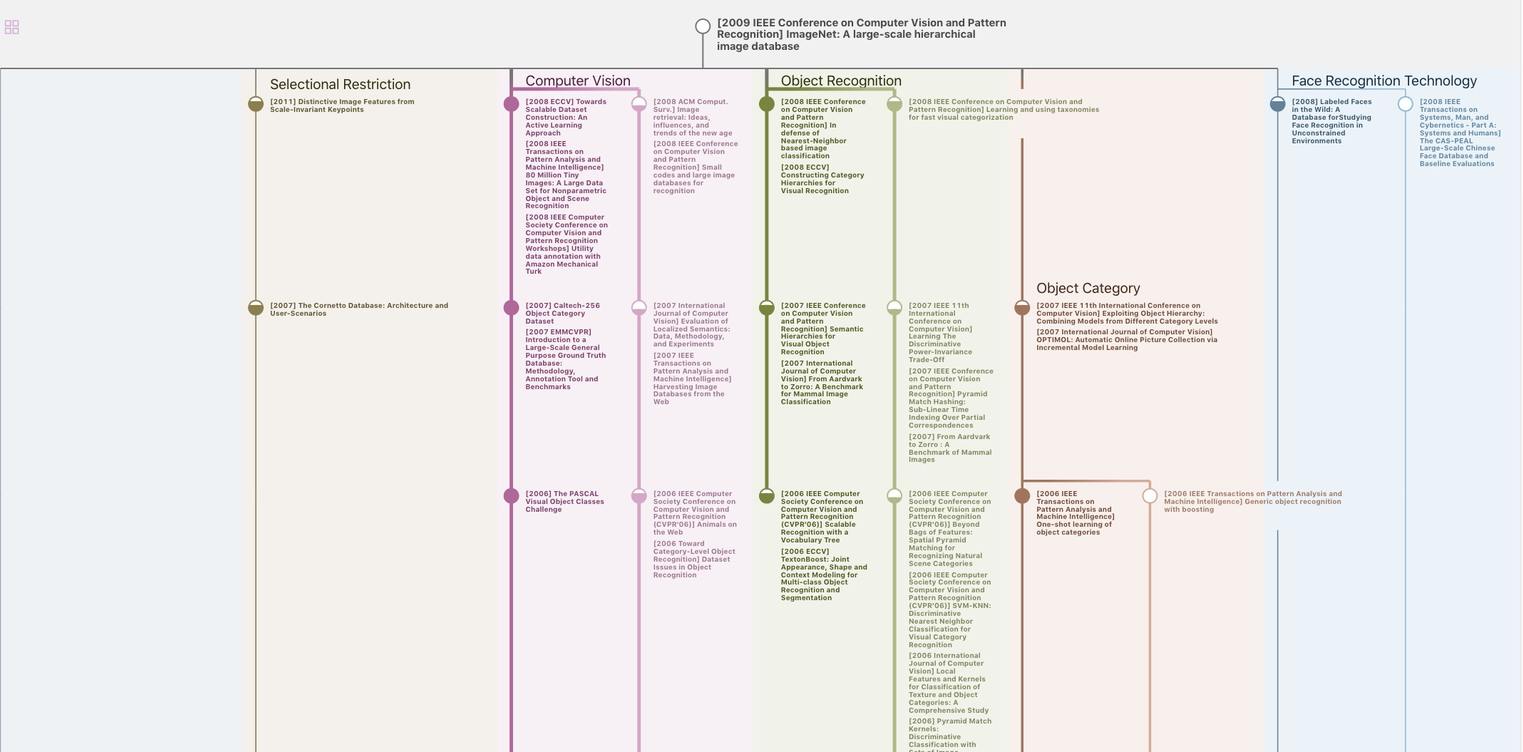
Generate MRT to find the research sequence of this paper
Chat Paper
Summary is being generated by the instructions you defined