Prediction of collapsibility of loess site based on artificial intelligence: comparison of different algorithms
Environmental Earth Sciences(2024)
摘要
Collapsibility affects loess engineering stability; the straightforward prediction for the self-weight collapsibility coefficient of loess is useful to determine the collapsibility type of loess site. In this study, three representative machine learning algorithms: multi-expression programming (MEP), random forest (RF) and support vector machine (SVM) are used to develop three straightforward prediction models for the loess self-weight collapsibility coefficient values, aiming to evaluate the collapsibility of loess sites according to the basic physical properties. Considering soil depth and compression modulus, a large database including five input variables, i.e., initial water content, initial void ratio, plasticity index, soil depth and compression modulus is established. Genetic algorithm (GA) is used to optimize the hyper-parameters of the RF and SVM models. The results show that the three models developed for the training set and the test set have high prediction accuracy for the self-weight collapsibility coefficient of loess. The monotonicity, sensitivity and robustness of the three prediction models are analyzed, showing the consistency between different models, but slightly different, which verifies the feasibility of the model. On the whole, the high prediction accuracy of the RF model is first recommended, but no explicit expression. The MEP model with explicit expression is also recommended for ease of application. The SVM model is the last option according to the situation.
更多查看译文
关键词
Loess collapsibility parameters,Machine learning,Multi-expression programming,Random forest,Support vector machine,Genetic algorithm
AI 理解论文
溯源树
样例
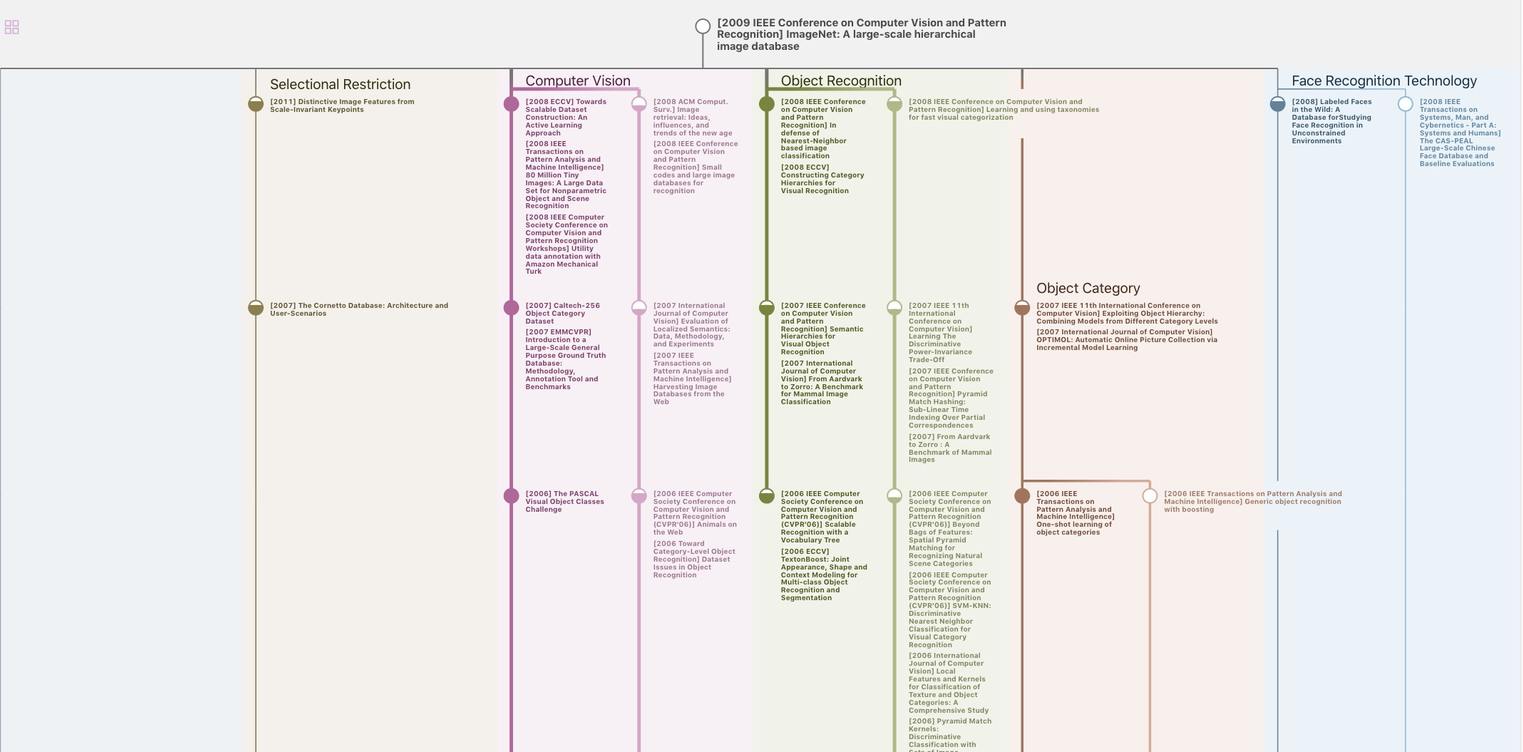
生成溯源树,研究论文发展脉络
Chat Paper
正在生成论文摘要