Predicting below and above-ground peanut biomass and maturity using multi-target regression
COMPUTERS AND ELECTRONICS IN AGRICULTURE(2024)
摘要
Accurate prediction of peanut growth and maturity is crucial to improve crop management and strengthening breeding programs. Remote sensing technology, such as satellites and drones, can facilitate in-season crop growth monitoring through the collection of high temporal and spatial imagery that capture differences in crop spectral reflectance. Current robust algorithms for predicting multiple peanut growth variables have not been proposed. This study aimed to develop algorithms for prediction of multiple peanut growth variables using a multi-output regression (MTR) approach. Two commercial irrigated fields, one of 8.6 ha located in Society Hill Alabama (AL), U.S. (study area 1), and the second of 54.76 ha (ha) located in Eufaula, Alabama (AL), U.S. (Study area 2) were used for data collection. Peanut biomass samples were collected weekly from 20 locations which each field. Two Peanut Maturity Indices (PMI orange to black and brown to black) were measured from manual assessment of maturity using the peanut profile board. MTR models were built to establish a functional relationship between peanut aboveground biomass, maturity, and spectral reflectance changes of the canopy over time using Random Forest (RF) and K-nearest neighbor. Reflectance from individual spectral bands and vegetation indices (VI) of the biomass sampling location were extracted from Planet scope (R) satellite images. The algorithms were developed using toolkits available in the Scikit-learn python library and were evaluated using the mean absolute error (MAE) metric. The RF algorithm was able to output multiple numeric values of peanut maturity indices upon VI and spectral bands, supporting the hypothesis that MTR can predict peanut maturity at the field level. The use of spectral reflectance from satellite images resulted in a small prediction error of 9 % for PMI using brown to black pods and 10 % when predicting PMI using orange to black pods. The MTR model was also accurate in predicting aboveground biomass (MAE = 1301 kg ha-1) compared to pod weight (MAE = 1103 kg ha-1). The study demonstrated a promising method to assess within-field variability of peanut maturity using remote sensing images, which could reduce the subjectivity of the manual method.
更多查看译文
关键词
Biomass,Crop maturity,Machine learning,Multi-task learning,Remote sensing
AI 理解论文
溯源树
样例
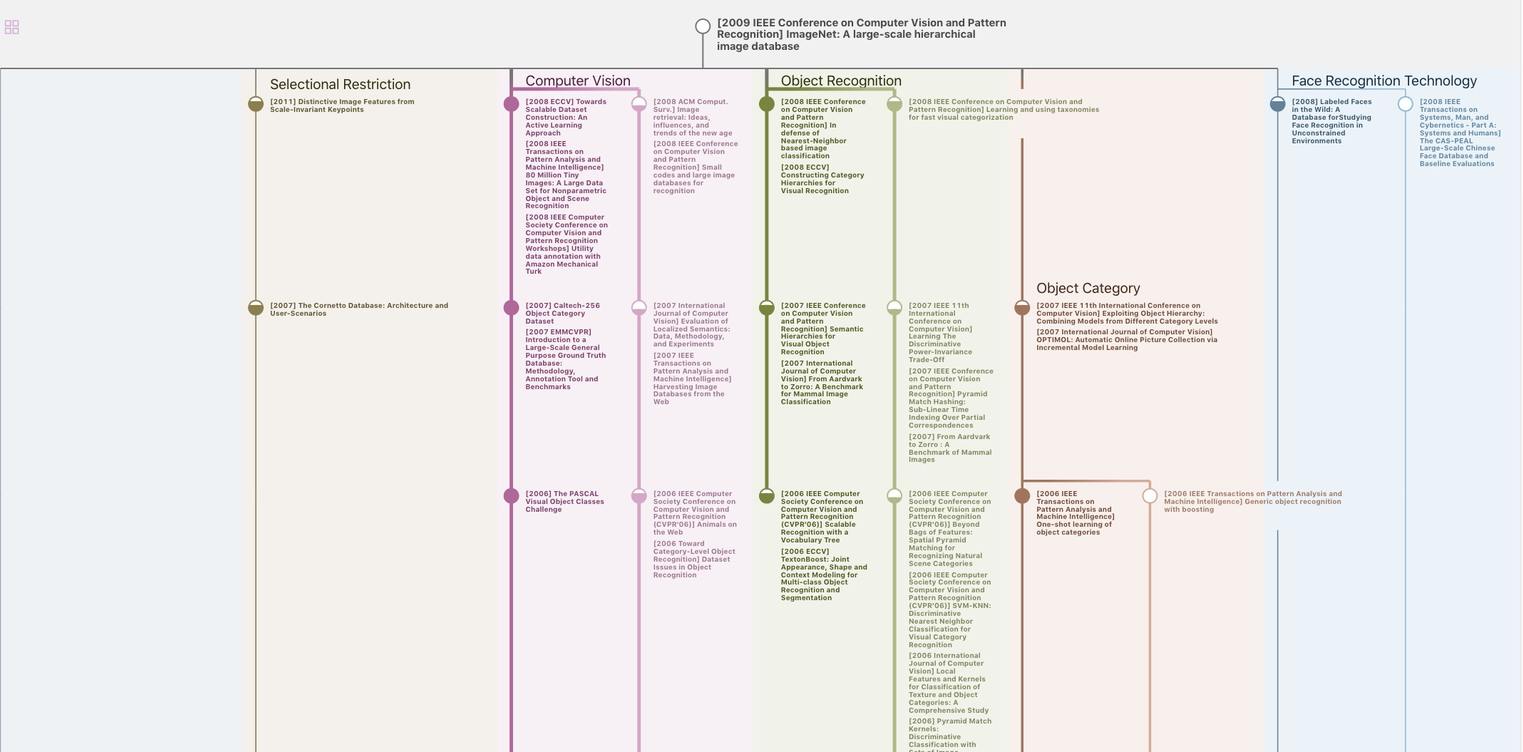
生成溯源树,研究论文发展脉络
Chat Paper
正在生成论文摘要