Stochastic Weakly Convex Optimization Beyond Lipschitz Continuity
CoRR(2024)
摘要
This paper considers stochastic weakly convex optimization without the
standard Lipschitz continuity assumption. Based on new adaptive regularization
(stepsize) strategies, we show that a wide class of stochastic algorithms,
including the stochastic subgradient method, preserve the 𝒪 ( 1 /
√(K)) convergence rate with constant failure rate. Our analyses rest on
rather weak assumptions: the Lipschitz parameter can be either bounded by a
general growth function of x or locally estimated through independent
random samples.
更多查看译文
AI 理解论文
溯源树
样例
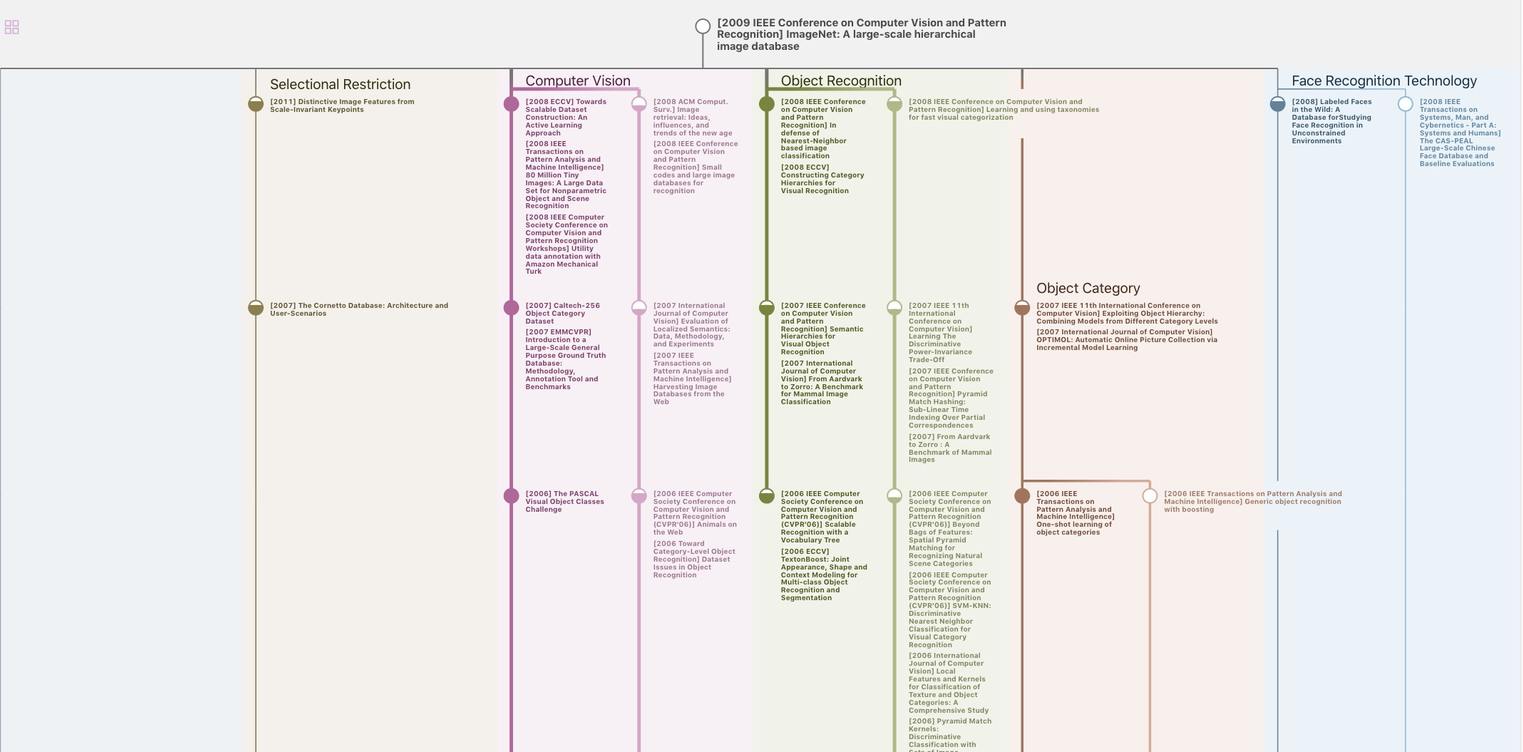
生成溯源树,研究论文发展脉络
Chat Paper
正在生成论文摘要