Dynamic Long-Term Time-Series Forecasting via Meta Transformer Networks
IEEE Transactions on Artificial Intelligence(2024)
摘要
A reliable long-term time-series forecaster is highly demanded in practice
but comes across many challenges such as low computational and memory
footprints as well as robustness against dynamic learning environments. This
paper proposes Meta-Transformer Networks (MANTRA) to deal with the dynamic
long-term time-series forecasting tasks. MANTRA relies on the concept of fast
and slow learners where a collection of fast learners learns different aspects
of data distributions while adapting quickly to changes. A slow learner tailors
suitable representations to fast learners. Fast adaptations to dynamic
environments are achieved using the universal representation transformer layers
producing task-adapted representations with a small number of parameters. Our
experiments using four datasets with different prediction lengths demonstrate
the advantage of our approach with at least 3% improvements over the
baseline algorithms for both multivariate and univariate settings. Source codes
of MANTRA are publicly available in
.
更多查看译文
关键词
time-series forecasting,concept drifts,transformers,deep learning
AI 理解论文
溯源树
样例
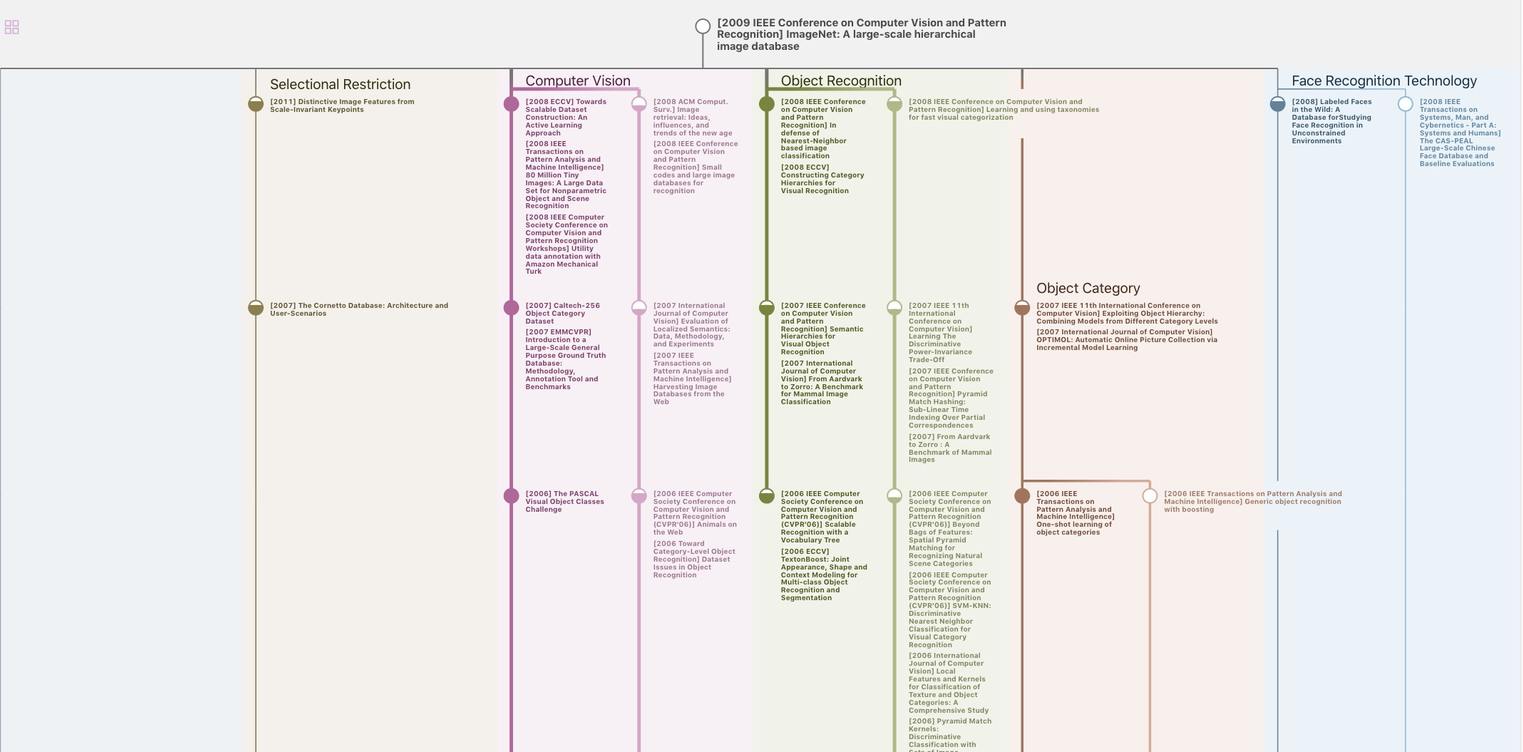
生成溯源树,研究论文发展脉络
Chat Paper
正在生成论文摘要