A quantitative evaluation of the deep learning model of segmentation and measurement of cervical spine MRI in healthy adults
JOURNAL OF APPLIED CLINICAL MEDICAL PHYSICS(2024)
摘要
PurposeTo evaluate the 3D U-Net model for automatic segmentation and measurement of cervical spine structures using magnetic resonance (MR) images of healthy adults.Materials and methodsMR images of the cervical spine from 160 healthy adults were collected retrospectively. A previously constructed deep-learning model was used to automatically segment anatomical structures. Segmentation and localization results were checked by experienced radiologists. Pearson's correlation analyses were conducted to examine relationships between patient and image parameters.ResultsNo measurement was significantly correlated with age or sex. The mean values of the areas of the subarachnoid space and spinal cord from the C2/3 (cervical spine 2-3) to C6/7 intervertebral disc levels were 102.85-358.12 mm2 and 53.71-110.32 mm2, respectively. The ratios of the areas of the spinal cord to the subarachnoid space were 0.25-0.68. The transverse and anterior-posterior diameters of the subarachnoid space were 14.77-26.56 mm and 7.38-17.58 mm, respectively. The transverse and anterior-posterior diameters of the spinal cord were 9.11-16.02 mm and 5.47-10.12 mm, respectively.ConclusionA deep learning model based on 3D U-Net automatically segmented and performed measurements on cervical spine MR images from healthy adults, paving the way for quantitative diagnosis models for spinal cord diseases.
更多查看译文
关键词
cervical spine,deep learning,magnetic resonance imaging,morphometrics
AI 理解论文
溯源树
样例
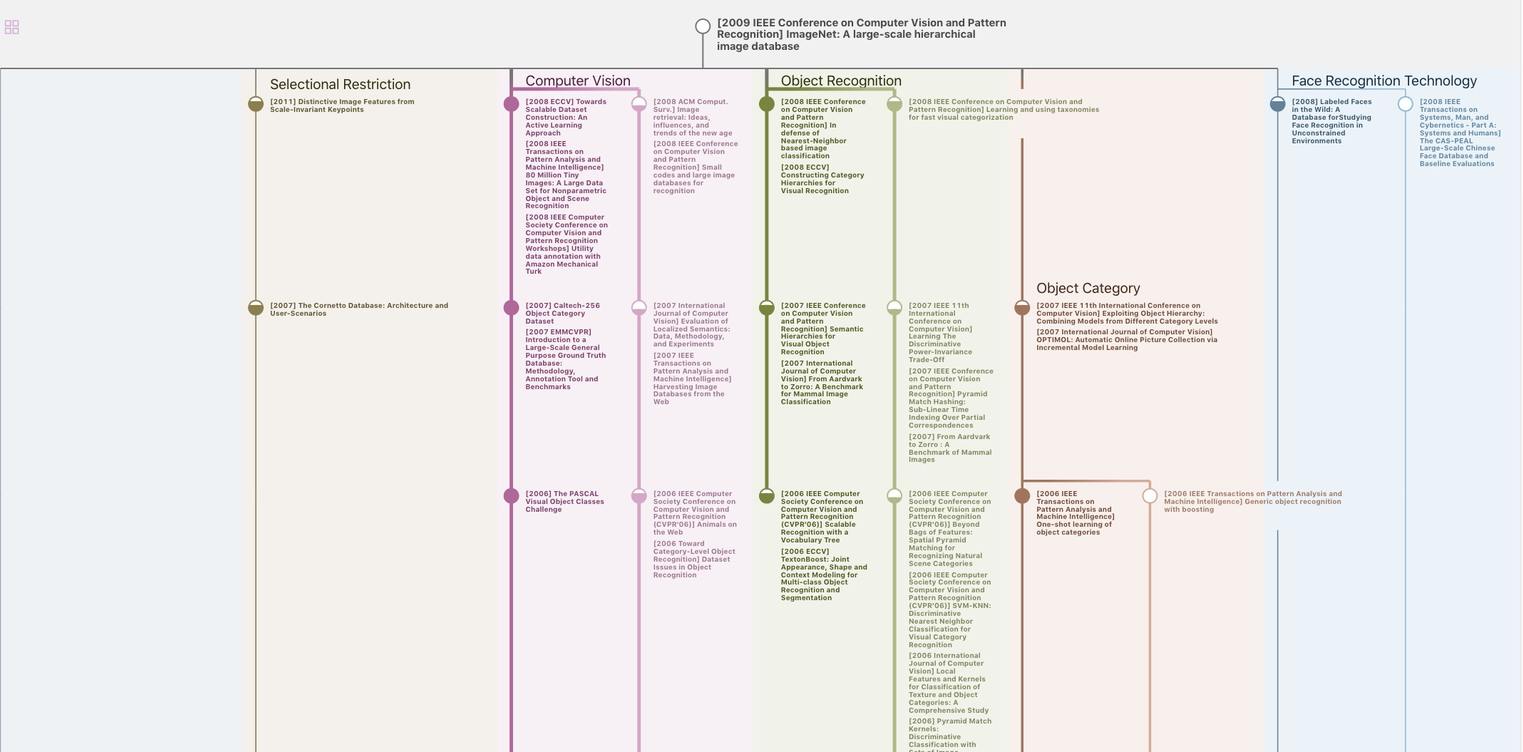
生成溯源树,研究论文发展脉络
Chat Paper
正在生成论文摘要