A separate modeling approach to noisy displacement prediction of concrete dams via improved deep learning with frequency division
ADVANCED ENGINEERING INFORMATICS(2024)
摘要
Commonly, the displacement of a concrete dam is considered as a significant indicator to assess the structure's performance during its service life. Since the displacement data exhibits non-linear, non-stationary, and timevarying characteristics, it is difficult to evaluate, interpret, and predict the dam displacement satisfactorily. Moreover, the raw monitoring data obtained by the deployed sensors always contains varying degrees of noise interference, which makes the prediction more challenge. A separate modeling approach with frequency division is thereby proposed based on an improved deep learning model, the so-called deformable temporal convolutional network (DTCN). The main aim is to pursue high-precision displacement prediction while discarding useless information in the data noise. A novel deep learning DTCN-based model is developed to adaptively adjust the range of receiving fields based on various frequency characteristics, achieving various extraction capabilities. The DTCN fuses the dilated convolution with a deformable convolution mechanism. It compensates for the problem of the conventional statistical model for concrete dam displacement prediction that ignores sudden changes in water levels and temperatures in monitoring sequences. A real-world dam project then evaluates the viability and effectiveness of the proposed method. The comprehensive model evaluations and statistical analyses are carried out. Compared with other commonly used data-driven models, the results reveal that our approach can predict dam displacements competitively because it has the minimum root mean square error, mean absolute error, and the maximum correlation coefficient in the analyzed monitoring points.
更多查看译文
关键词
Concrete dam displacement prediction,Data noise,Temporal convolutional networks,Deformable convolution,Variational mode decomposition
AI 理解论文
溯源树
样例
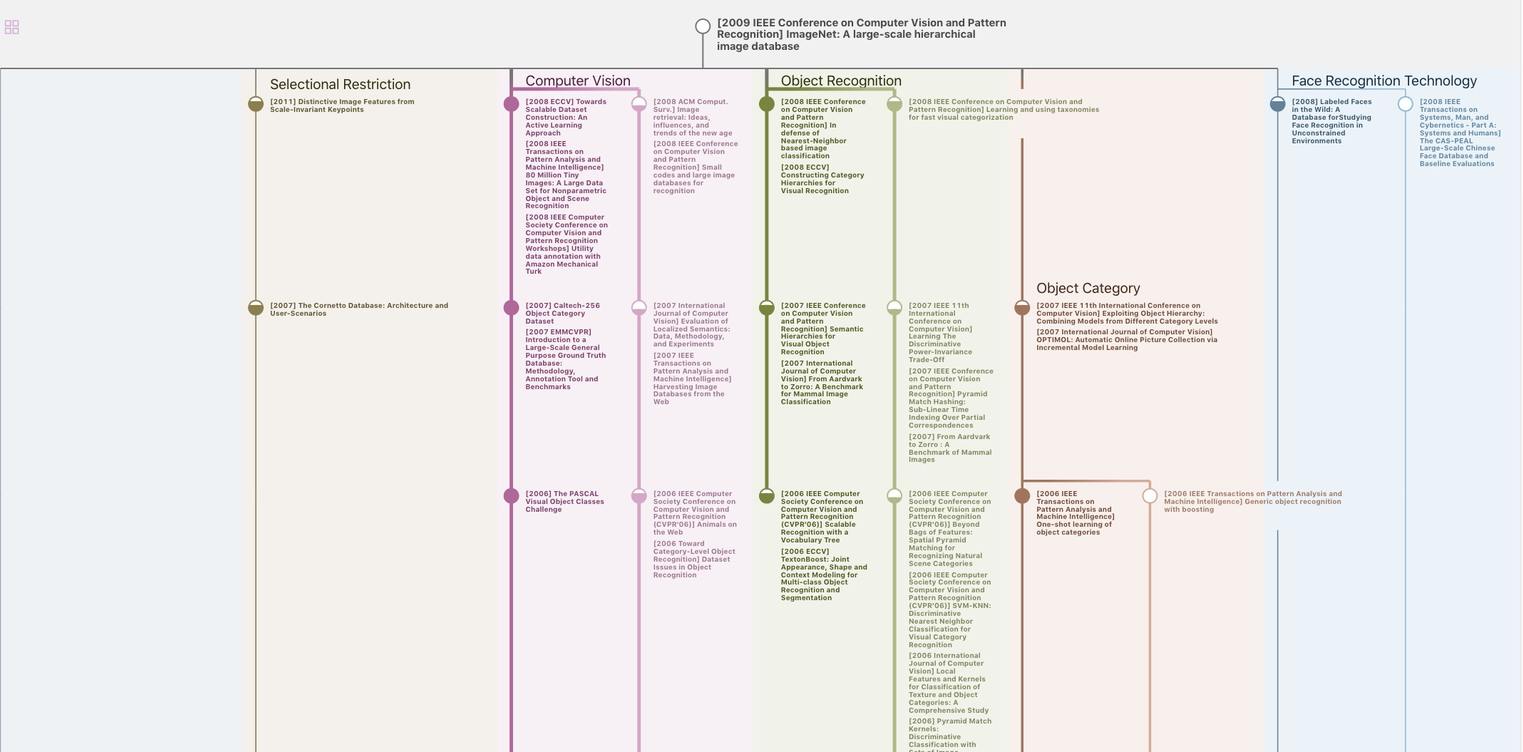
生成溯源树,研究论文发展脉络
Chat Paper
正在生成论文摘要