Grammar-based evolutionary approach for automated workflow composition with domain-specific operators and ensemble diversity
APPLIED SOFT COMPUTING(2024)
摘要
The process of extracting valuable and novel insights from raw data involves a series of complex steps. In the realm of Automated Machine Learning (AutoML), a significant research focus is on automating aspects of this process, specifically tasks like selecting algorithms and optimising their hyper -parameters. A particularly challenging task in AutoML is automatic workflow composition (AWC). AWC aims to identify the most effective sequence of data preprocessing and machine learning algorithms, coupled with their best hyper -parameters, for a specific dataset. However, existing AWC methods are limited in how many and in what ways they can combine algorithms within a workflow. Addressing this gap, this paper introduces EvoFrow, a grammar -based evolutionary approach for AWC. EvoFrow enhances the flexibility in designing workflow structures, empowering practitioners to select algorithms that best fit their specific requirements. EvoFrow stands out by integrating two innovative features. First, it employs a suite of genetic operators, designed specifically for AWC, to optimise both the structure of workflows and their hyper -parameters. Second, it implements a novel updating mechanism that enriches the variety of predictions made by different workflows. Promoting this diversity helps prevent the algorithm from overfitting. With this aim, EvoFrow builds an ensemble whose workflows differ in their misclassified instances. To evaluate EvoFrow's effectiveness, we carried out empirical validation using a set of classification benchmarks. We begin with an ablation study to demonstrate the enhanced performance attributable to EvoFrow's unique components. Then, we compare EvoFrow with other AWC approaches, encompassing both evolutionary and non -evolutionary techniques. Our findings show that EvoFrow's specialised genetic operators and updating mechanism substantially outperform current leading methods in predictive performance. Additionally, EvoFrow is capable of discovering workflow structures that other approaches in the literature have not considered.
更多查看译文
关键词
AutoML,Automated workflow composition,Algorithm selection,Hyper-parameter optimisation,Grammar-guided genetic programming,Ensemble learning,Classification
AI 理解论文
溯源树
样例
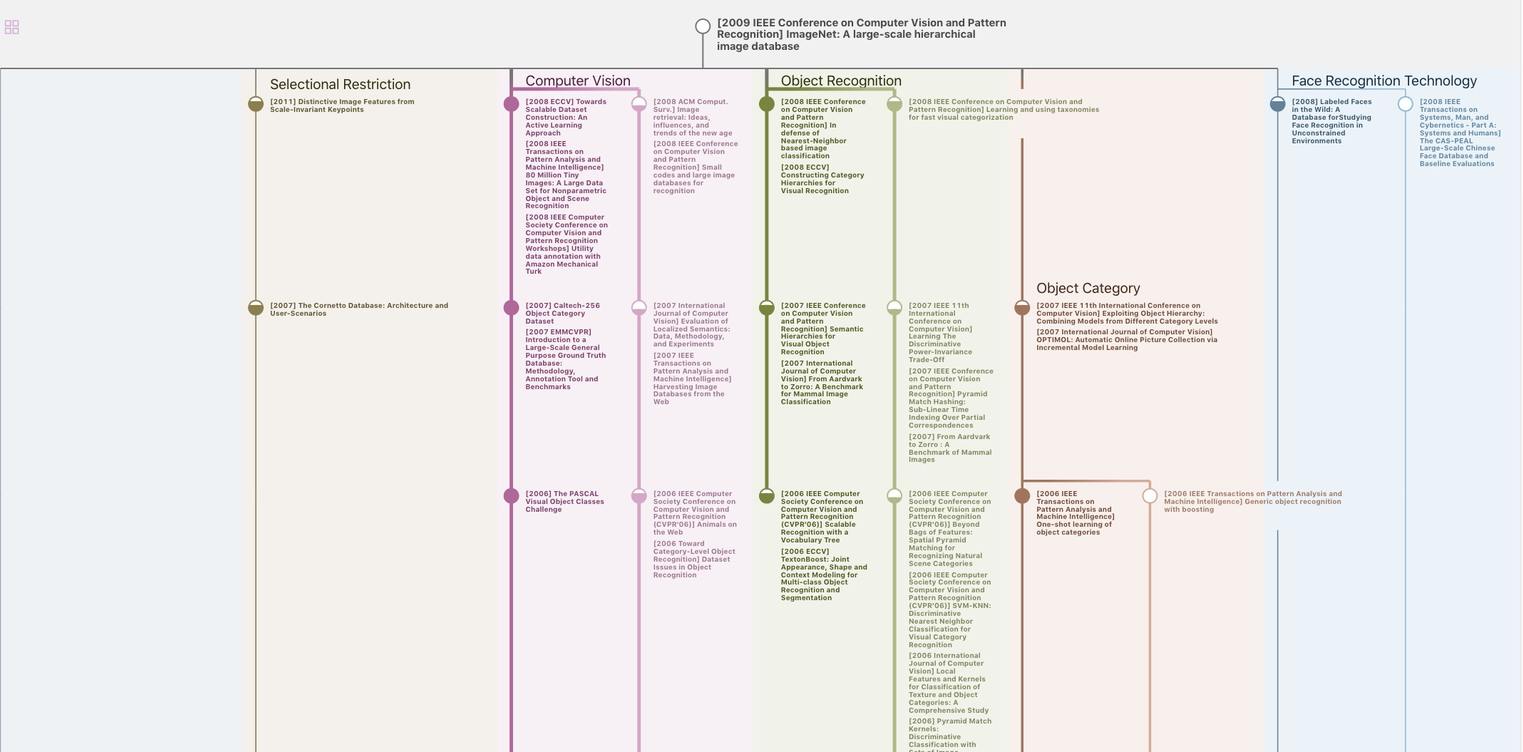
生成溯源树,研究论文发展脉络
Chat Paper
正在生成论文摘要