Generating Out-of-Distribution Examples via Feature Crossover against Overconfidence Issue
2023 IEEE 11th International Conference on Information, Communication and Networks (ICICN)(2023)
摘要
The problem of overconfident predictions on out-of-distribution (OOD) samples poses a significant challenge to the reliability and robustness of deep neural networks (DNNs). The reason for OOD overconfidence issue is that DNNs only learn the features of in-distribution (ID) samples in the training stage, while lacking the supervisory signal of OOD samples. Therefore, we can alleviate this problem by constructing an auxiliary OOD dataset. If the auxiliary OOD dataset belongs to OOD and is close to ID, it can teach the model more OOD knowledge, and further distinguish OOD samples from ID, which is manifested by the model outputting more evenly distributed confidence score for OOD samples. The key to solving this problem lies in how to construct such a high-quality auxiliary OOD dataset. In this paper, we have made preliminary explorations into the fusion of high-level features between samples, and proposed a simple but efficient method of generating OOD samples through feature crossover in the feature space. This method only requires ID data to generate a large number of OOD samples. Experimental results have demonstrated that using our method to construct OOD samples can effectively alleviate the problem of DNNs being overly confident on OOD samples.
更多查看译文
关键词
out-of-distribution detection,virtual outlier synthesis,deep learning
AI 理解论文
溯源树
样例
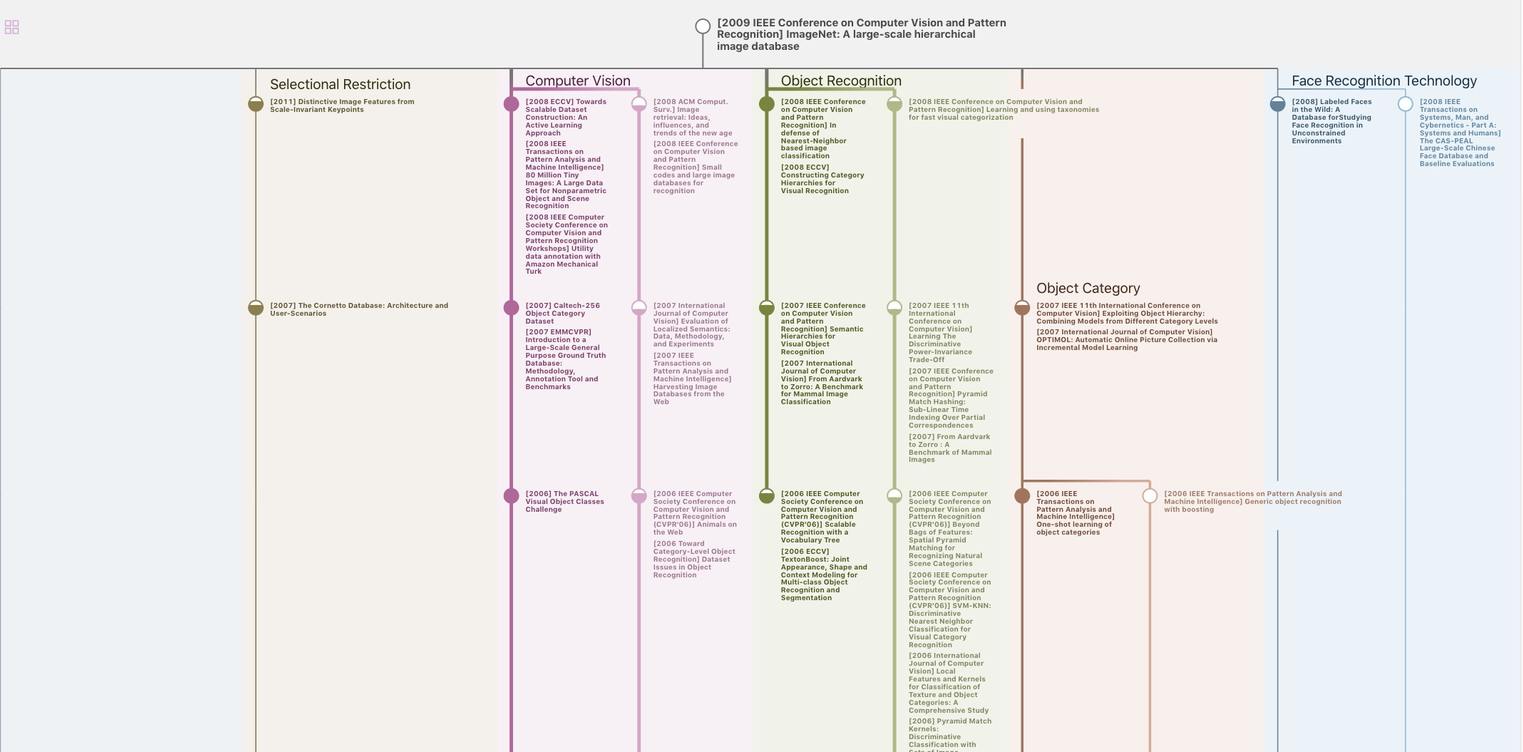
生成溯源树,研究论文发展脉络
Chat Paper
正在生成论文摘要