Reconstruction of Large-Scale Missing Data in Remote Sensing Images Using Extend-GAN
IEEE GEOSCIENCE AND REMOTE SENSING LETTERS(2024)
摘要
Numerous studies have been conducted on missing data recovery in remote sensing images, such as cloud removal and dead pixels restoration. Nevertheless, reconstructing continuous, extensive, and complete missing areas still poses a significant challenge. In this letter, we propose a new architecture named Extend-generative adversarial network (GAN), which leverages only a low-resolution image with relaxed requirements on spatial resolution and acquisition time as a condition to reconstruct a high-resolution image with large-scale missing areas. We equip Extend-GAN with learnable adaptive region normalization (LARN) to adjust the intensity distribution of pixels to reduce color distortion. We also introduce a new loss function into the training process of Extend-GAN, namely the structural similarity (SSIM)-based triplet loss, which helps to preserve the between missing parts and known regions. Gaofen-2 and Landsat-9 image pairs are used to validate the proposed method. Extend-GAN performs better when comprehensively evaluated on visual effect, quantitative metrics, processing speed, etc. Code is available at https://github.com/yc-cui/Extend-GAN.
更多查看译文
关键词
Training,Earth,Artificial satellites,Generative adversarial networks,Spatial resolution,Remote sensing,Image reconstruction,Generative adversarial network (GAN),image reconstruction,remote sensing images,triplet loss
AI 理解论文
溯源树
样例
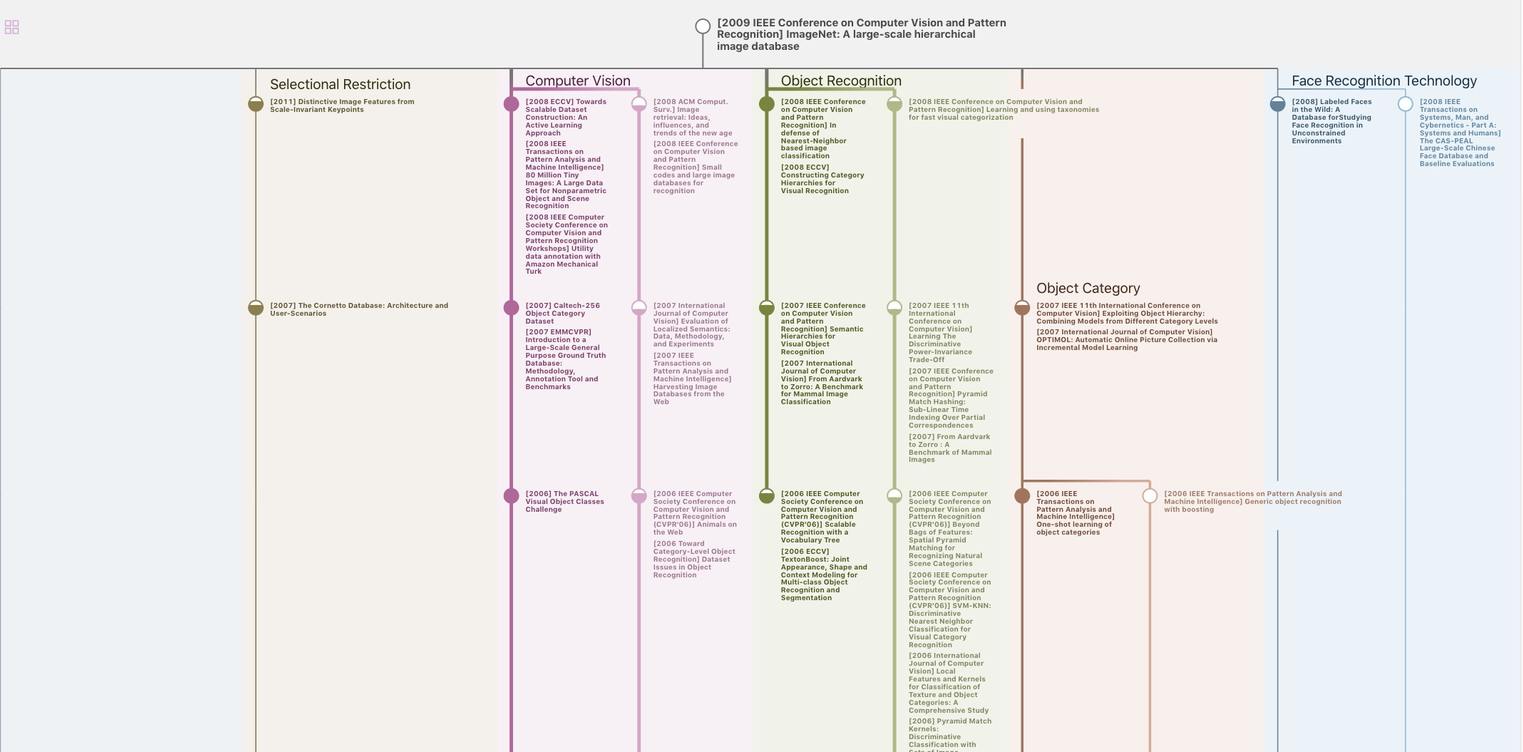
生成溯源树,研究论文发展脉络
Chat Paper
正在生成论文摘要