R-LKDepth: Recurrent Depth Learning With Larger Kernel
IEEE SIGNAL PROCESSING LETTERS(2024)
摘要
Monocular depth estimation is a critical task with significant potential for real-time applications. However, current networks for monocular depth estimation exhibit a trade-off between computational overhead and accuracy. Current top-performing coarse-to-fine networks excel in building multi-scale features and thus get a satisfactory result, but suffer from an excessive count of model parameters of its deeper backbone. In contrast, recurrent networks significantly save the use of model parameters, but their performance is often restricted by the smaller receptive field, leading to insufficient local evidence to handle complex objects and homogeneous regions. In this letter, we aim to alleviate the limitation and thus propose an efficient recurrent network R-LKDepth. Specifically, we first introduce the larger kernel scheme with a careful design to effectively extract the image features with larger receptive fields. Furthermore, we propose a group-wise multi-scale self-attention based refine-feed (GMSA-RF) module, which efficiently aggregates multi-scale information for recurrent refinement procedure. The resulting network, R-LKDepth, significantly improves the depth estimation performance over complex objects and homogeneous regions and achieves state-of-the-art performance on standard benchmarks with superior cross-dataset generalization capability.
更多查看译文
关键词
Estimation,Kernel,Feature extraction,Standards,Decoding,Training,Image resolution,Monocular depth estimation,recurrent refinement,self-supervised learning,larger receptive fields
AI 理解论文
溯源树
样例
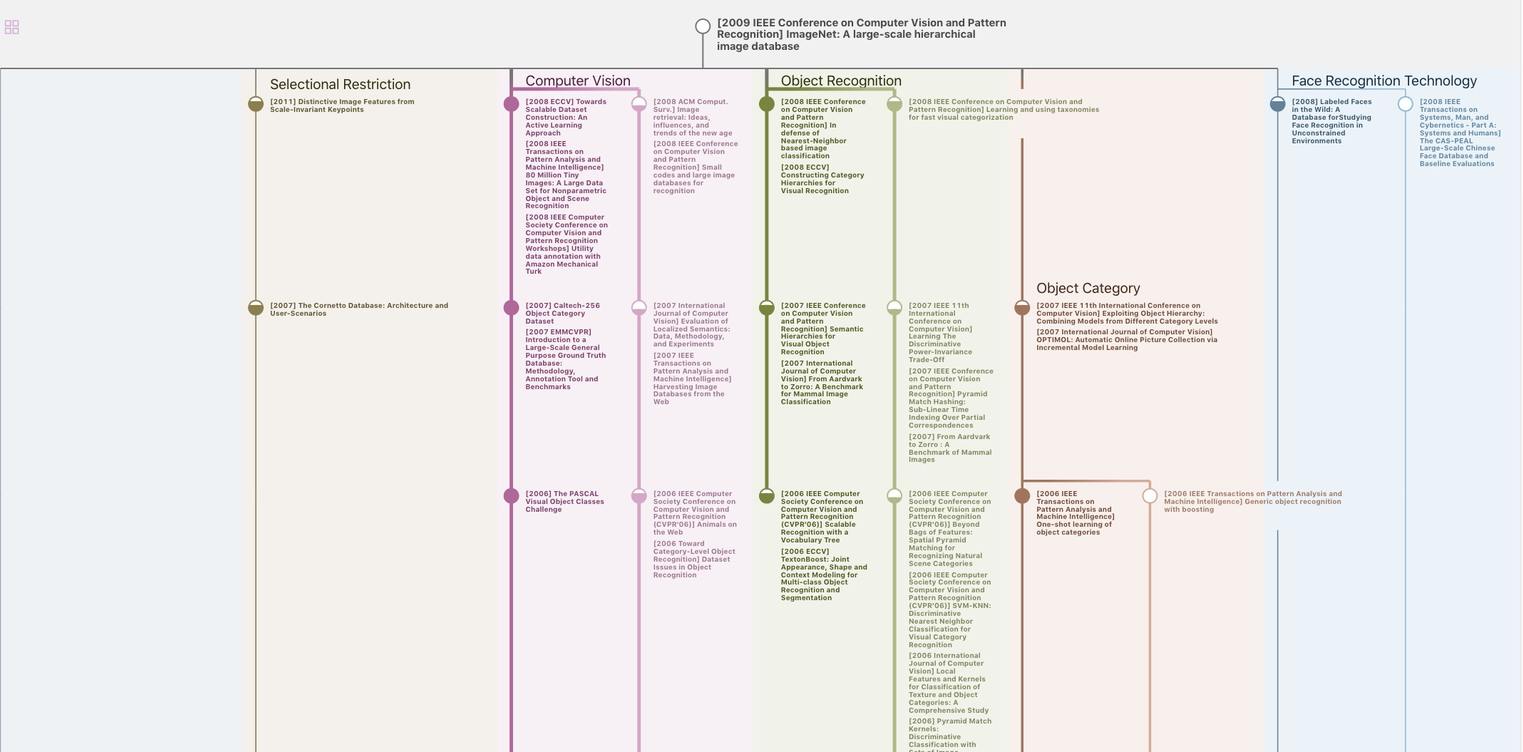
生成溯源树,研究论文发展脉络
Chat Paper
正在生成论文摘要