TFAN: Twin-Flow Axis Normalization for Human Motion Prediction.
IEEE Signal Processing Letters(2024)
摘要
Human motion prediction involves forecasting upcoming body poses from historically observed sequences. Presently, various methods focus on modeling the positions of skeletal joints to generate future movements. Beyond solely using the joint position information, this letter further explores the informative bone vector information between joints for human motion prediction. Therefore, we propose TFAN, which integrates joint position and bone vector information to achieve more precise predictions. Additionally, joint positions and bone vectors, both represented as 3D vectors, were frequently imprecisely modeled due to neglect of variations in data distribution across axes in prior methods. Instead, we introduce Axis Normalization, which standardizes each coordinate axis individually, enhancing the model's sensitivity to data distribution disparities. Through experimental evaluations on the Human3.6M, AMASS, and 3DPW datasets, we consistently demonstrate that TFAN outperforms other existing methods. Our code will be available at
https://github.com/Deante-dx/TFAN
.
更多查看译文
关键词
Motion prediction,skeleton data,normalization method
AI 理解论文
溯源树
样例
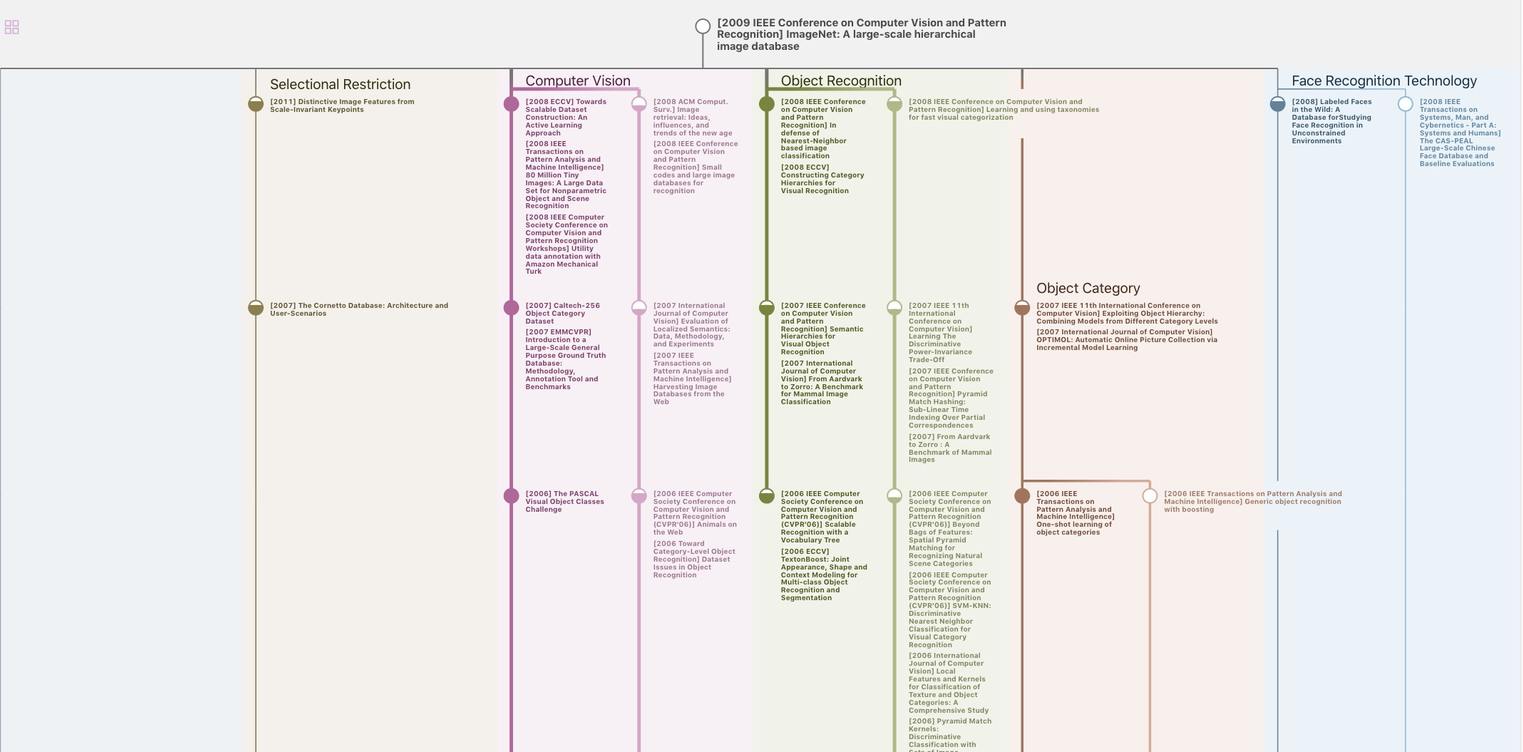
生成溯源树,研究论文发展脉络
Chat Paper
正在生成论文摘要